Prediction of pulmonary pressure after Glenn shunts by computed tomography–based machine learning models
European Radiology(2019)
摘要
Objectives This study aimed to develop non-invasive machine learning classifiers for predicting post–Glenn shunt patients with low and high risks of a mean pulmonary arterial pressure (mPAP) > 15 mmHg based on preoperative cardiac computed tomography (CT). Methods This retrospective study included 96 patients with functional single ventricle who underwent a bidirectional Glenn procedure between November 1, 2009, and July, 31, 2017. All patients underwent post-procedure CT, followed by cardiac catheterization. Overall, 23 morphologic parameters were manually extracted from cardiac CT images for each patient. The Mann-Whitney U or chi-square test was applied to select the most significant predictors. Six machine learning algorithms including logistic regression, Naive Bayes, random forest (RF), linear discriminant analysis, support vector machine, and K-nearest neighbor were used for modeling. These algorithms were independently trained on 100 train-validation random splits with a 3:1 ratio. Their average performance was evaluated by area under the curve (AUC), accuracy, sensitivity, and specificity. Results Seven CT morphologic parameters were selected for modeling. RF obtained the best performance, with mean AUC of 0.840 (confidence interval [CI] 0.832–0.850) and 0.787 (95% CI 0.780–0.794); sensitivity of 0.815 (95% CI 0.797–0.833) and 0.778 (95% CI 0.767–0.788), specificity of 0.766 (95% CI 0.748–0.785) and 0.746 (95% CI 0.735–0.757); and accuracy of 0.782 (95% CI 0.771–0.793) and 0.756 (95% CI 0.748–0.764) in the training and validation cohorts, respectively. Conclusions The CT-based RF model demonstrates a good performance in the prediction of mPAP, which may reduce the need for right heart catheterization in post–Glenn shunt patients with suspected mPAP > 15 mmHg. Key Points • Twenty-three candidate descriptors were manually extracted from cardiac computed tomography images, and seven of them were selected for subsequent modeling. • The random forest model presents the best predictive performance for pulmonary pressure among all methods. • The computed tomography–based machine learning model could predict post–Glenn shunt pulmonary pressure non-invasively.
更多查看译文
关键词
Heart diseases, Lung, Pressure, Multi-detector computed tomography, Machine learning
AI 理解论文
溯源树
样例
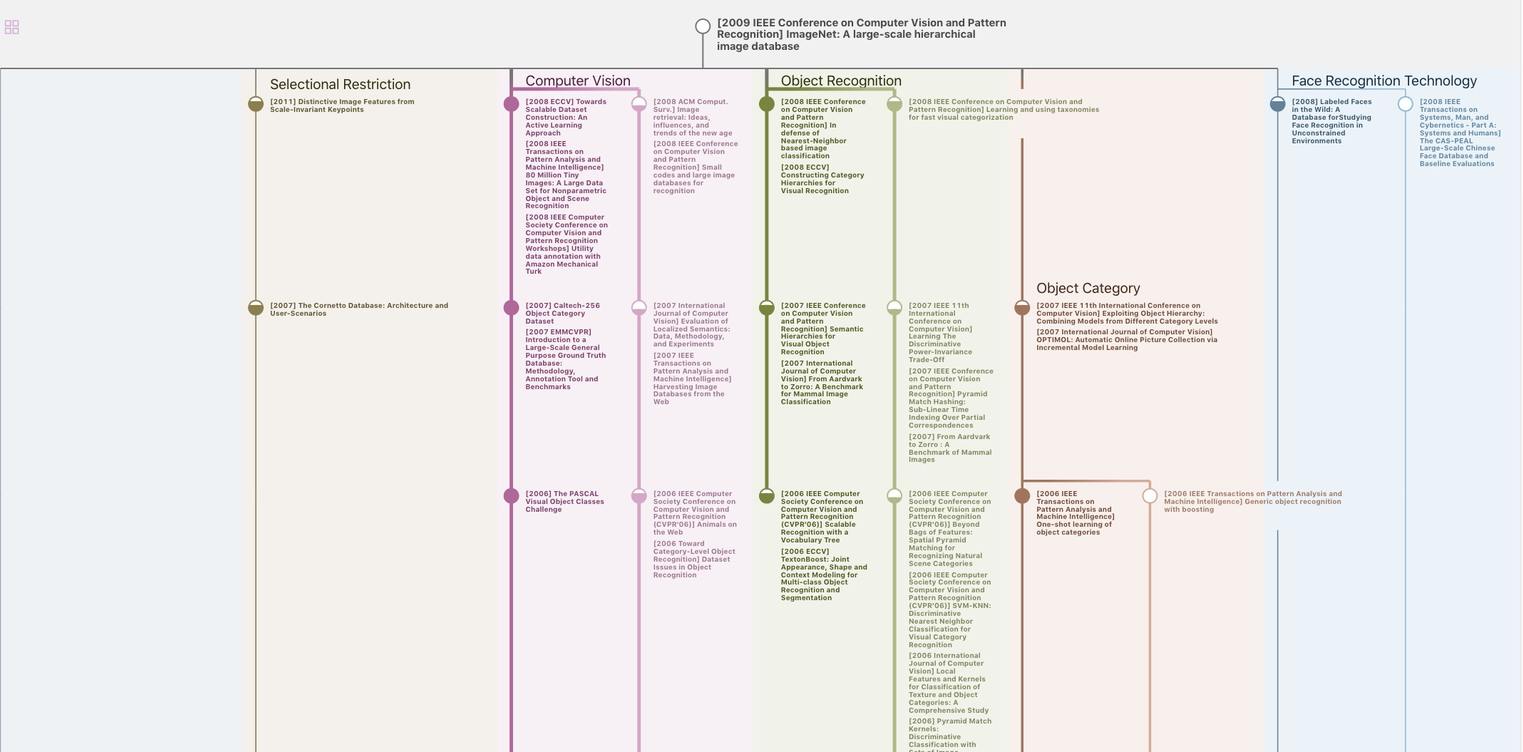
生成溯源树,研究论文发展脉络
Chat Paper
正在生成论文摘要