Malware Detection on Highly Imbalanced Data through Sequence Modeling
Proceedings of the 12th ACM Workshop on Artificial Intelligence and Security(2019)
Abstract
We explore the task of Android malware detection based on dynamic analysis of application activity sequences using deep learning techniques. We show that analyzing a sequence of the activities is informative for detecting malware, but that analyzing longer sequences does not necessarily lead to a more accurate model. In the real-world scenario, the number of malware is low compared to that of harmless applications. Our dataset has more than 180,000 samples, two-thirds of which are malware. This dataset is significantly larger than other datasets used in previous studies. We mimic real-world cases by randomly sampling a small portion of malware samples. Using the state-of-the-art model BERT, we show that it is possible to achieve desired malware detection performance with an extremely unbalanced dataset. We find that our BERT based model achieves an F1 score of 0.919 with just 0.5% of the examples being malware, which significantly outperforms current state-of-the-art approaches. The results validate the effectiveness of our proposed method in dealing with highly imbalanced datasets.
MoreTranslated text
Key words
imbalanced data, malware detection, sequence modeling
AI Read Science
Must-Reading Tree
Example
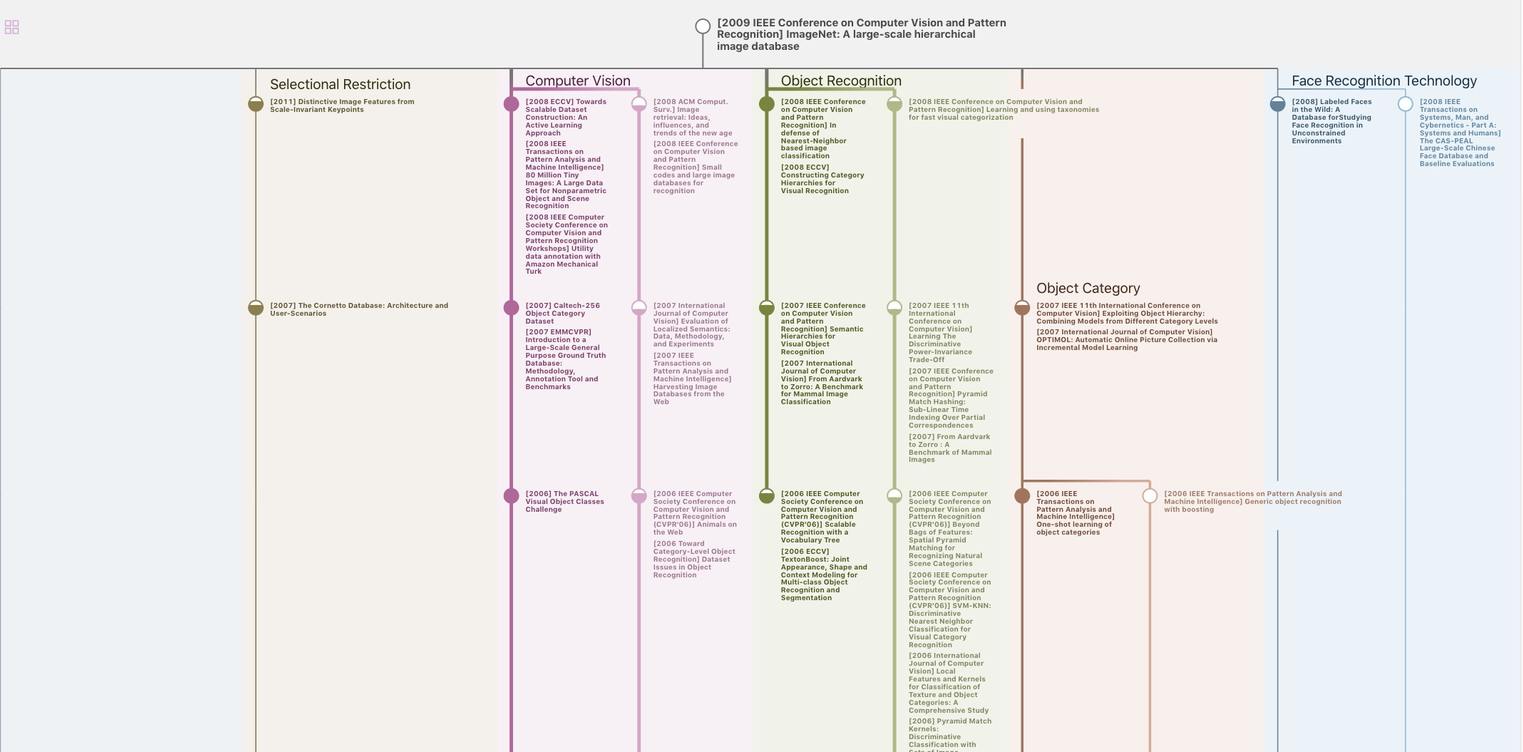
Generate MRT to find the research sequence of this paper
Chat Paper
Summary is being generated by the instructions you defined