Adder-Only Convolutional Neural Network with Binary Input Image
2019 IEEE 62nd International Midwest Symposium on Circuits and Systems (MWSCAS)(2019)
摘要
Convolutional neural networks (CNNs) have performed exceptionally well on a variety of image classification tasks but need significant amount of memory and computational resources. In this paper, we propose an adder-only CNN (AO-CNN) inference engine, that has its weights and/or outputs at each layer reduced to either powers of two or zero, thus replacing the multiplication operations with a simple right or left shift and hence reducing the computation significantly. The proposed AO-CNN architecture, demonstrated using three sets of data-sets, viz., MNIST, EMNIST, and SVHN, performs with ≈ 80% accuracy or more, while using minimal hardware and taking only binary input images, i.e., 1 and 0. This could pave the way for realization of image-sensors with 1-bit resolution images and the corresponding CNN based classification engine being integrated into low-power internet-of-things (IoT) devices.
更多查看译文
关键词
adder-only CNN inference engine,multiplication operations,AO-CNN architecture,binary input image,image sensors,image resolution,adder-only convolutional neural network,convolutional neural networks,image classification tasks,computational resources,CNN based classification engine,MNIST,EMNIST,SVHN
AI 理解论文
溯源树
样例
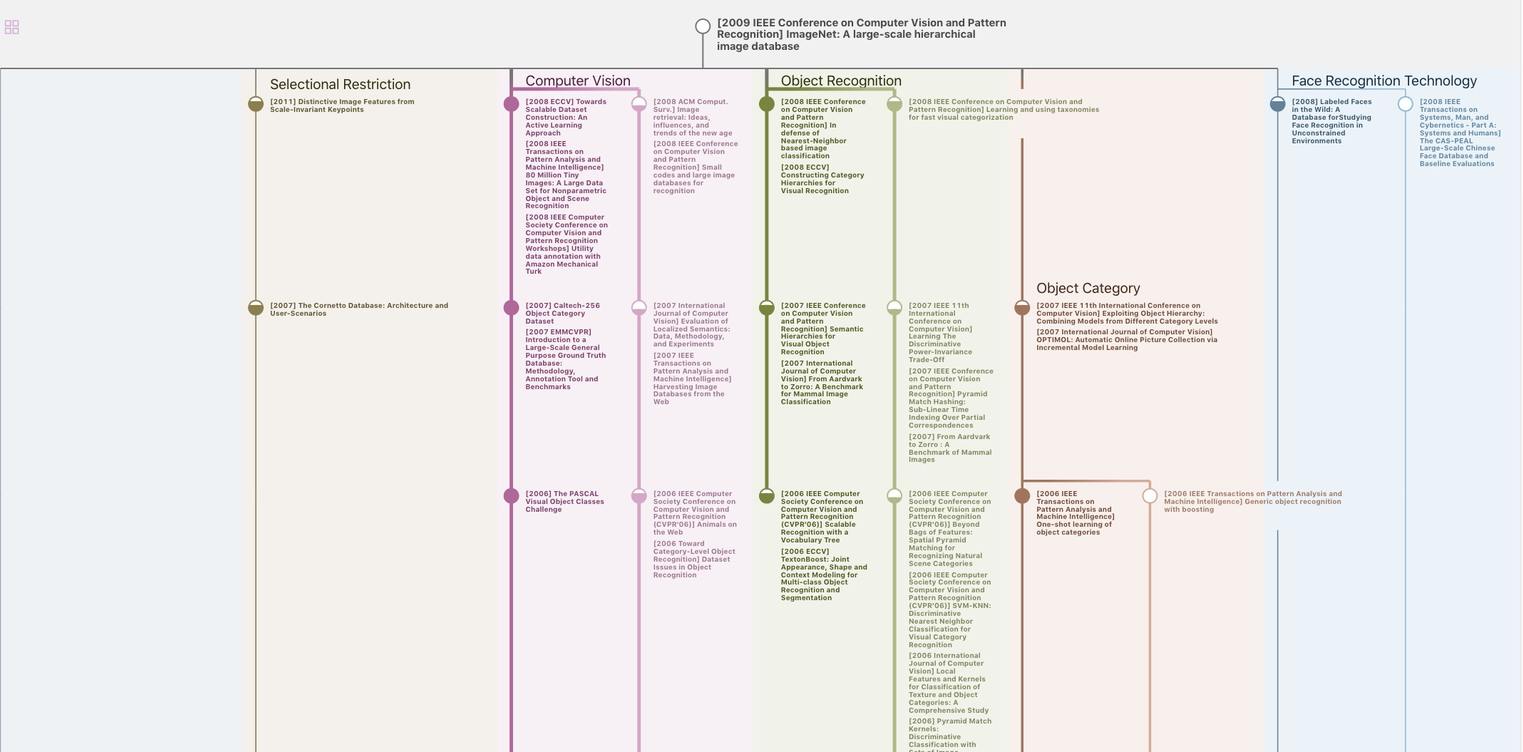
生成溯源树,研究论文发展脉络
Chat Paper
正在生成论文摘要