A deep supervised approach for ischemic lesion segmentation from multimodal MRI using Fully Convolutional Network.
Applied Soft Computing(2019)
摘要
The principle restorative step in the treatment of ischemic stroke depends on how fast the lesion is delineated from the Magnetic Resonance Imaging (MRI) images. This will serve as a vital aid to estimate the extent of damage caused to the brain cells. However, manual delineation of the lesion is time-consuming and it is subjected to intra-observer and inter-observer variability. Most of the existing methods for ischemic lesion segmentation rely on extracting handcrafted features followed by application of a machine learning algorithm. Identifying such features demand multi-domain expertise in Neuro-radiology as well as Image processing. This can be accomplished by learning the features automatically using Convolutional Neural Network (CNN). To perform segmentation, the spatial arrangement of pixel needs to be preserved in addition to learning local features of an image. Hence, a deep supervised Fully Convolutional Network (FCN) is presented in this work to segment the ischemic lesion. The highlight of this research is the application of Leaky Rectified Linear Unit activation in the last two layers of the network for a precise reconstruction of the ischemic lesion. By doing so, the network was able to learn additional features which are not considered in the existing U-Net architecture. Also, an extensive analysis was conducted in this research to select optimal hyper-parameters for training the FCN. A mean segmentation accuracy of 0.70 has been achieved from the experiments conducted on ISLES 2015 dataset. Experimental observations show that our proposed FCN method is 10% better than the existing works in terms of Dice Coefficient.
更多查看译文
关键词
Ischemic lesion,FCN,Segmentation,Deep learning,CNN,Brain MRI
AI 理解论文
溯源树
样例
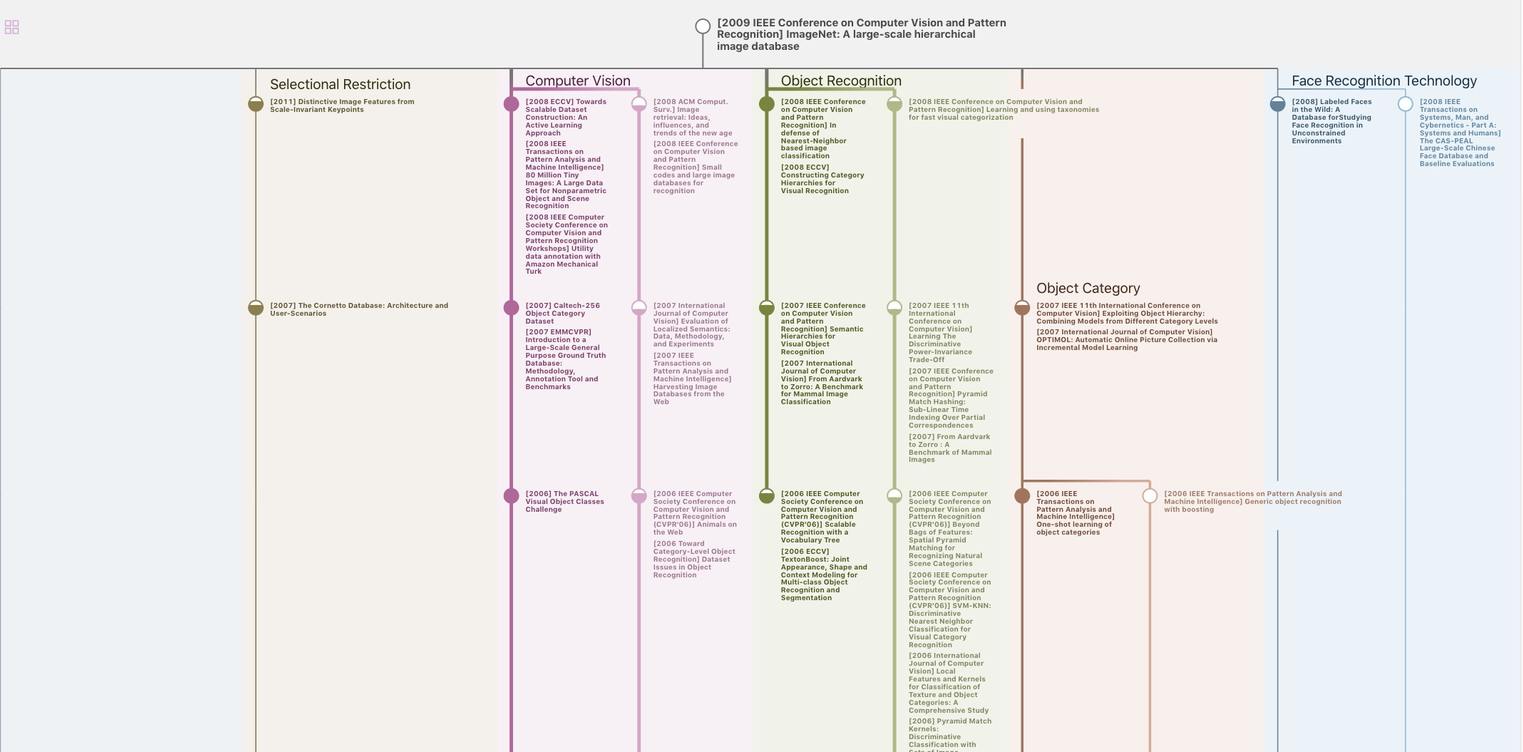
生成溯源树,研究论文发展脉络
Chat Paper
正在生成论文摘要