Big Rdf Data Storage, Computation, And Analysis: A Strawman'S Arguments
2019 39TH IEEE INTERNATIONAL CONFERENCE ON DISTRIBUTED COMPUTING SYSTEMS (ICDCS 2019)(2019)
摘要
RDF data is big and continues to grow rapidly. RDF data sets are typically viewed as heterogeneous graph data sets with complex correlations and multifaceted heterogeneity. Although there have been flurry of research on processing and analyzing RDF data, efficient storage, computation, analysis of big and growing RDF data continue to challenge multiple computer science disciplines, ranging from systems, network computing, data management to data analytics. In this paper, we make use of strawman arguments on big RDF data challenges with respect to storage, computation, analysis, focusing on identifying the grand challenges in developing high performance RDF storage, parallel computation, efficient distribution, and smart RDF analytics. We attempt to answer a number of important and frequently asked questions: (1) Do we really need RDF-specific storage techniques and algorithms for building efficient and high performance RDF stores? (2) How hard can it be to effectively parallelize big RDF data for high performance storage, computation, and mining? (3) What type of distribution models can we employ to scale RDF processing models and algorithms for real-time querying (subgraph pattern matching) and mining of big RDF data? (4) Can machine learning algorithms be leveraged to effectively mine and dive into RDF data? We attempt to answer these questions by sharing some of our results, our unique experiences, and our lessons learned from a collection of research projects in RDF systems research and development. We will also discuss our ongoing research endeavors, potential applications and avenues for future work.
更多查看译文
关键词
Storage, Graph Partitioning, Computation Framework, Query Processing, Graph Mining
AI 理解论文
溯源树
样例
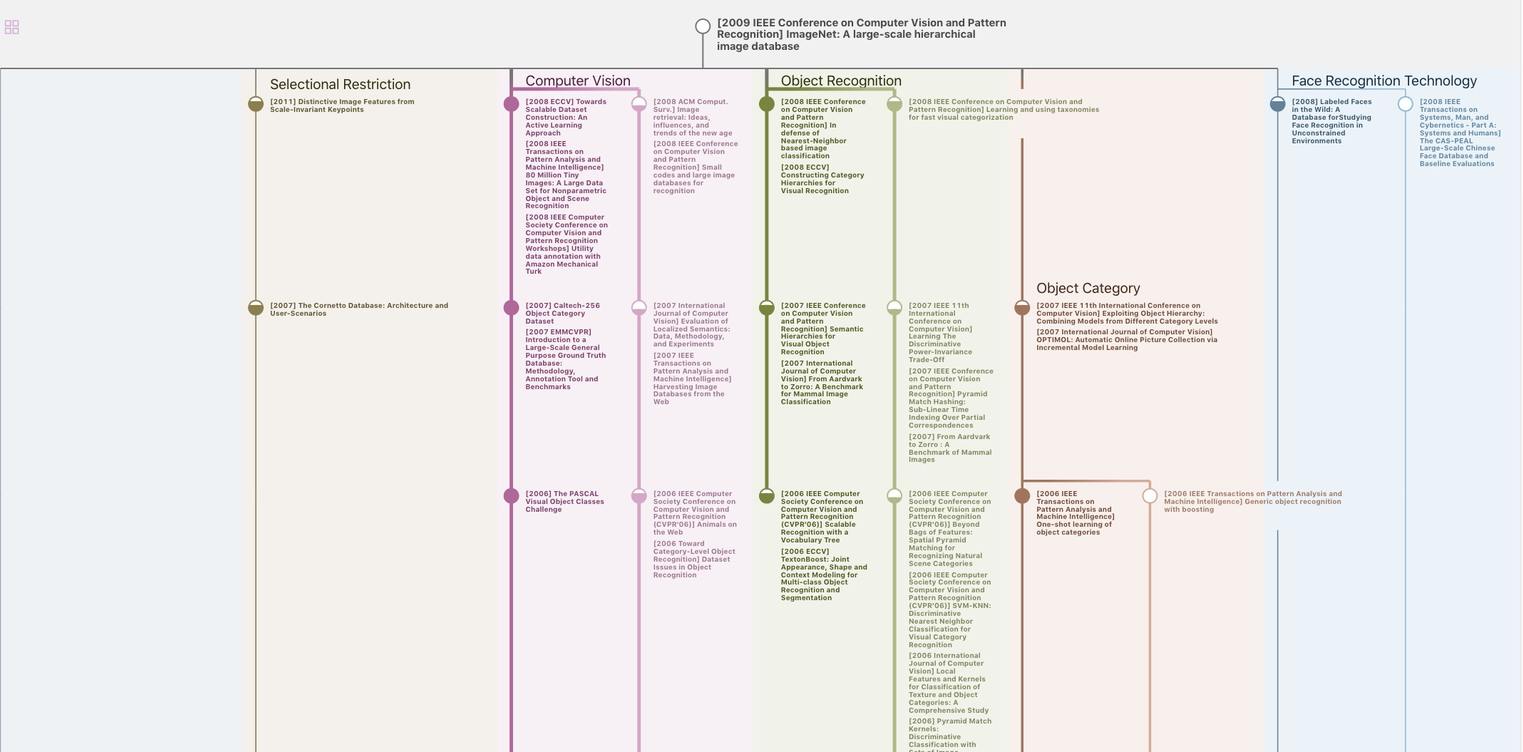
生成溯源树,研究论文发展脉络
Chat Paper
正在生成论文摘要