Predicting grasp success in the real world - A study of quality metrics and human assessment.
Robotics and Autonomous Systems(2019)
摘要
Grasp quality metrics aim at quantifying different aspects of a grasp configuration between a specific robot hand and object. They produce a numerical value that allows to rank grasp configurations and optimize based on them. Grasp quality metrics are a key part of most analytical grasp-planning approaches. Additionally, they are often used to generate ground-truth labels for synthetically generated grasp exemplars required for learning-based approaches. Recent studies have highlighted the limitations of grasp quality metrics when used to predict the outcome of a grasp execution on a real robot. In this paper, we systematically study how well seven commonly-used grasp quality metrics perform in the real world. To this end, we generated two datasets of grasp candidates in simulation, each one for a different robotic system. The quality of these synthetic grasp candidates is quantified by the aforementioned metrics. For validation, we developed an experimental procedure to accurately replicate grasp candidates on two real robotic systems and to evaluate the performance of each grasp. Given the resulting datasets, we trained different classifiers to predict grasp success using only grasp quality metrics as input. Our results show that combinations of quality metrics can achieve up to a 85% classification accuracy for real grasps.
更多查看译文
关键词
Grasping,Grasp simulation,Machine learning,Prediction model,Real grasp execution
AI 理解论文
溯源树
样例
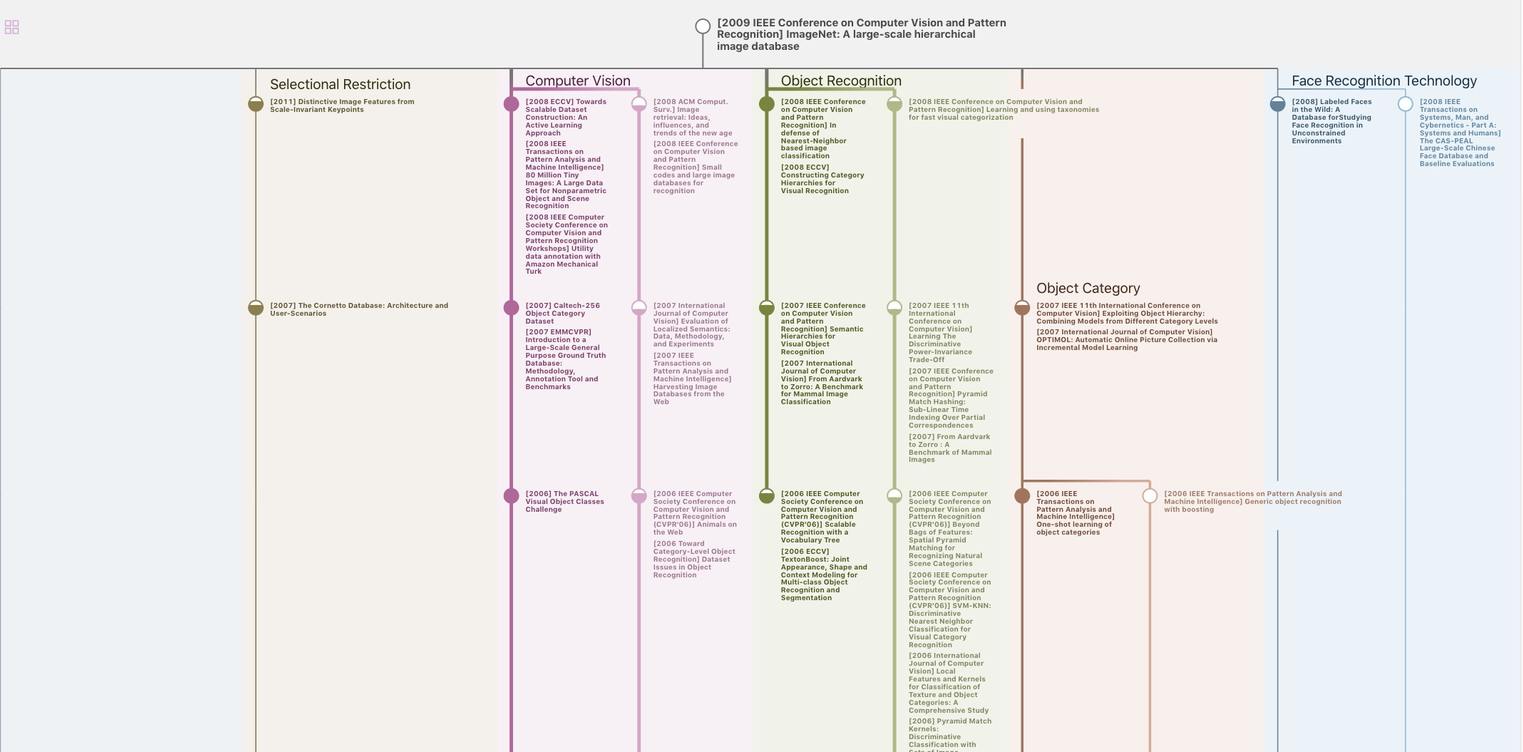
生成溯源树,研究论文发展脉络
Chat Paper
正在生成论文摘要