Post-Training 4-bit Quantization on Embedding Tables
CoRR(2019)
Abstract
Continuous representations have been widely adopted in recommender systems where a large number of entities are represented using embedding vectors. As the cardinality of the entities increases, the embedding components can easily contain millions of parameters and become the bottleneck in both storage and inference due to large memory consumption. This work focuses on post-training 4-bit quantization on the continuous embeddings. We propose row-wise uniform quantization with greedy search and codebook-based quantization that consistently outperforms state-of-the-art quantization approaches on reducing accuracy degradation. We deploy our uniform quantization technique on a production model in Facebook and demonstrate that it can reduce the model size to only 13.89% of the single-precision version while the model quality stays neutral.
MoreTranslated text
AI Read Science
Must-Reading Tree
Example
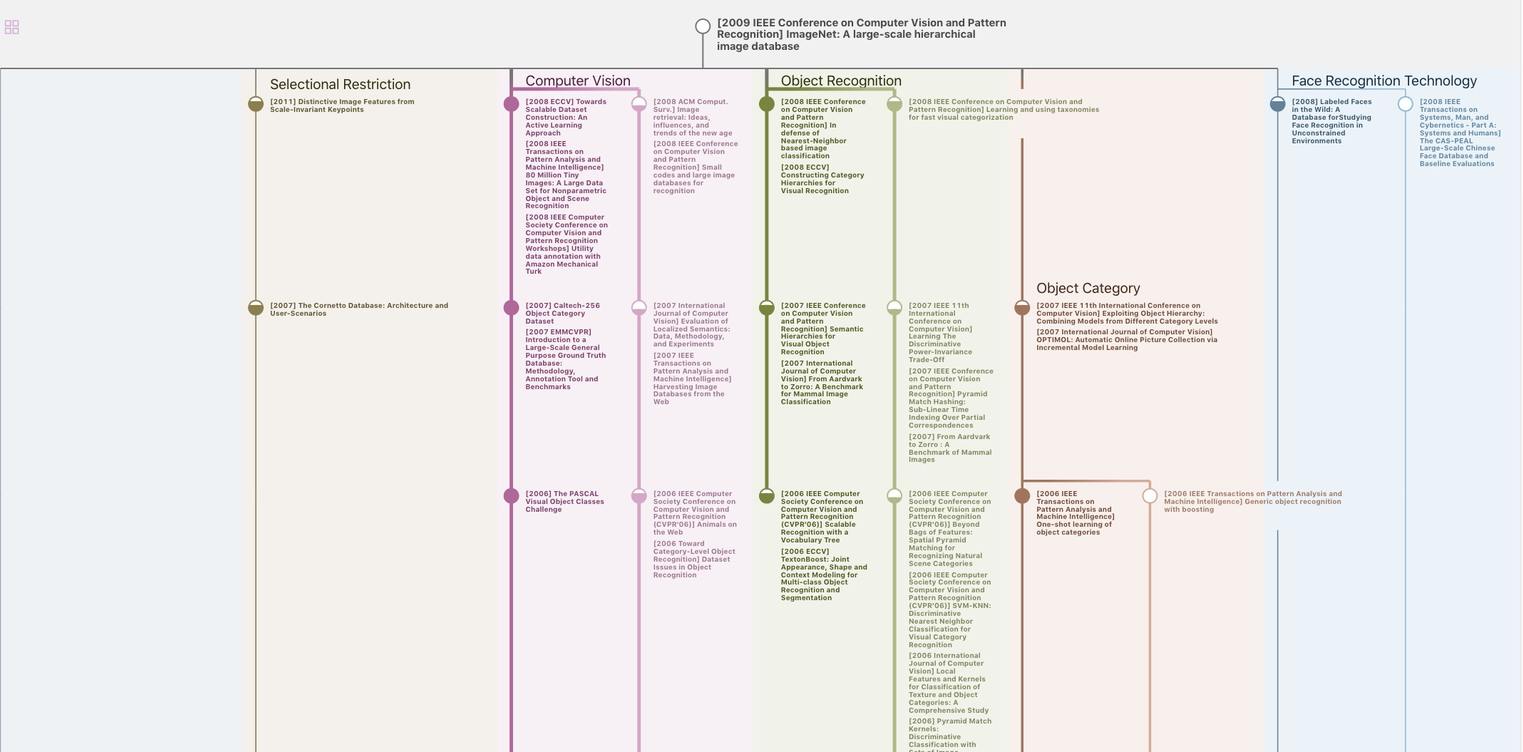
Generate MRT to find the research sequence of this paper
Chat Paper
Summary is being generated by the instructions you defined