Active Entity Recognition in Low Resource Settings
PROCEEDINGS OF THE 28TH ACM INTERNATIONAL CONFERENCE ON INFORMATION & KNOWLEDGE MANAGEMENT (CIKM '19)(2019)
Key words
Named Entity Recognition,Active Learning,Open Domain,Low Resource Settings
AI Read Science
Must-Reading Tree
Example
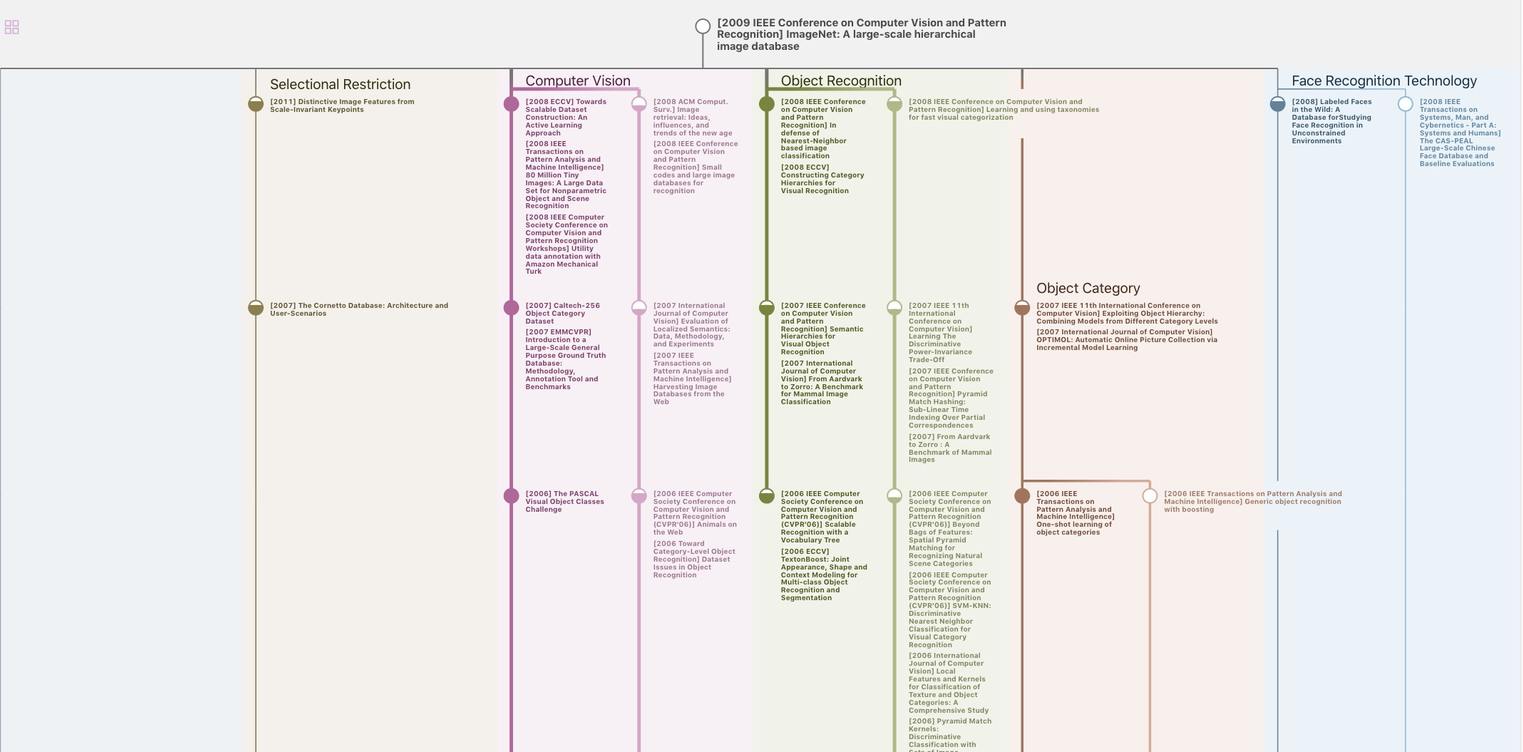
Generate MRT to find the research sequence of this paper
Chat Paper
Summary is being generated by the instructions you defined