HAES: A New Hybrid Approach for Movie Recommendation with Elastic Serendipity
Proceedings of the 28th ACM International Conference on Information and Knowledge Management(2019)
摘要
Recommendation systems provide good guidance for users to find their favorite movies from an overwhelming amount of options. However, most systems excessively pursue the recommendation accuracy and give rise to over-specialization, which triggers the emergence of serendipity. Hence, serendipity recommendation has received more attention in recent years, facing three key challenges: subjectivity in the definition, the lack of data, and users' floating demands for serendipity. To address these challenges, we introduce a new model called HAES, a H ybrid A pproach for movie recommendation with E lastic S erendipity, to recommend serendipitous movies. Specifically, we (1) propose a more objective definition of serendipity, \em content difference and \em genre accuracy, according to the analysis on a real dataset, (2) propose a new algorithm named JohnsonMax to mitigate the data sparsity and build weak ties beneficial to finding serendipitous movies, and (3) define a novel concept of elasticity in the recommendation, to adjust the level of serendipity flexibly and reach a trade-off between accuracy and serendipity. Extensive experiments on real-world datasets show that HAES enhances the serendipity of recommendations while preserving recommendation quality, compared to several widely used methods.
更多查看译文
关键词
elasticity, movie recommendation, serendipity
AI 理解论文
溯源树
样例
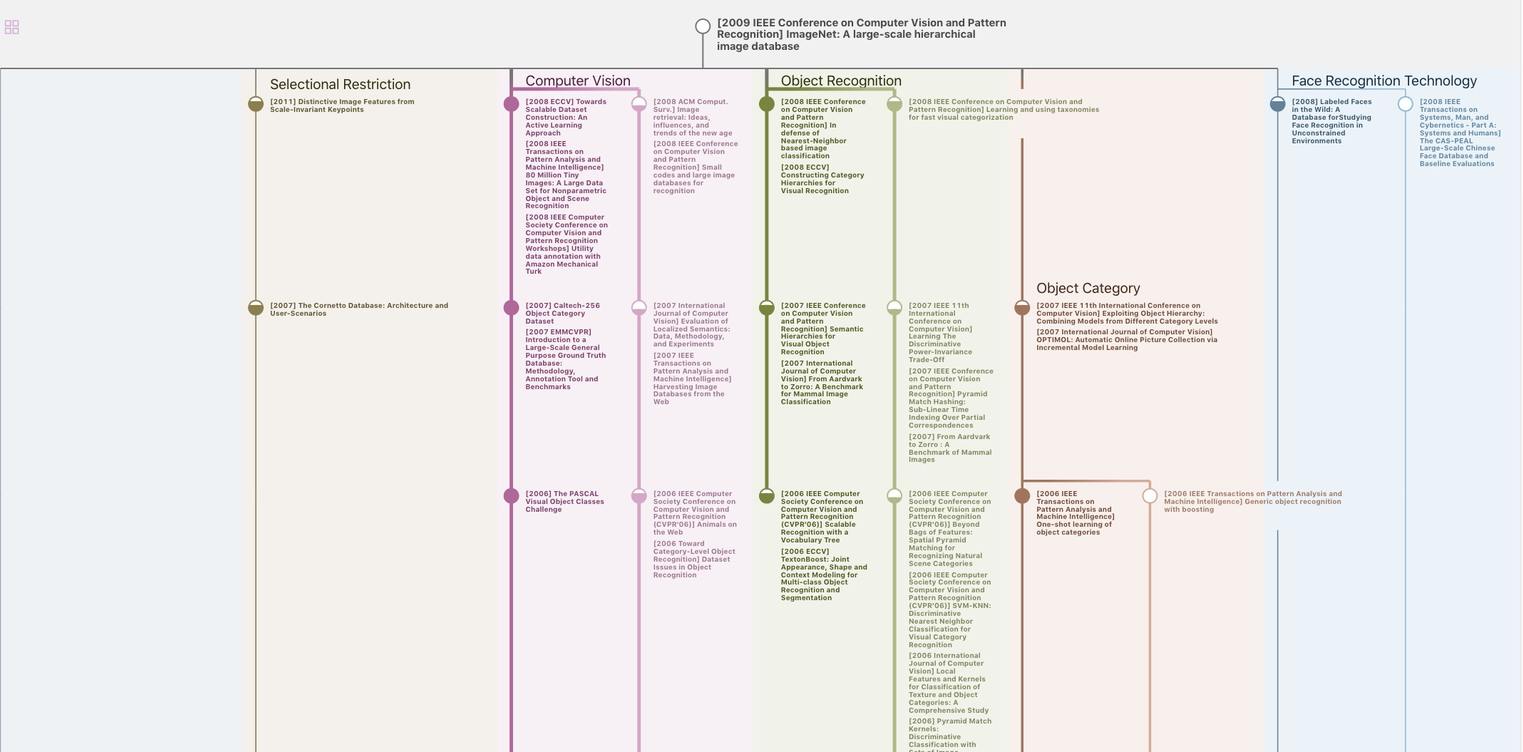
生成溯源树,研究论文发展脉络
Chat Paper
正在生成论文摘要