Deep Sequence-to-Sequence Entity Matching for Heterogeneous Entity Resolution
Proceedings of the 28th ACM International Conference on Information and Knowledge Management(2019)
摘要
Entity Resolution (ER) identifies records from different data sources that refer to the same real-world entity. Conventional ER approaches usually employ a structure matching mechanism, where attributes are aligned, compared and aggregated for ER decision. The structure matching approaches, unfortunately, often suffer from heterogeneous and dirty ER problems. That is, entities from different data sources are described using different schemas, and attribute values may be misplaced, missing, or noisy. In this paper, we propose a deep sequence-to-sequence entity matching model, denoted Seq2SeqMatcher, which can effectively solve the heterogeneous and dirty problems by modeling ER as a token-level sequence-to-sequence matching task. Specifically, we propose an align-compare-aggregate neural network for Seq2Seq entity matching, which can learn the representations of tokens, capture the semantic relevance between tokens, and aggregate matching evidence for accurate ER decisions in an end-to-end manner. Experimental results show that, by comparing entity records in token level and learning all components in an end-to-end manner, our Seq2Seq entity matching model can achieve remarkable performance improvements on 9 standard entity resolution benchmarks.
更多查看译文
关键词
attribute heterogeneity, deep learning, entity resolution, matching
AI 理解论文
溯源树
样例
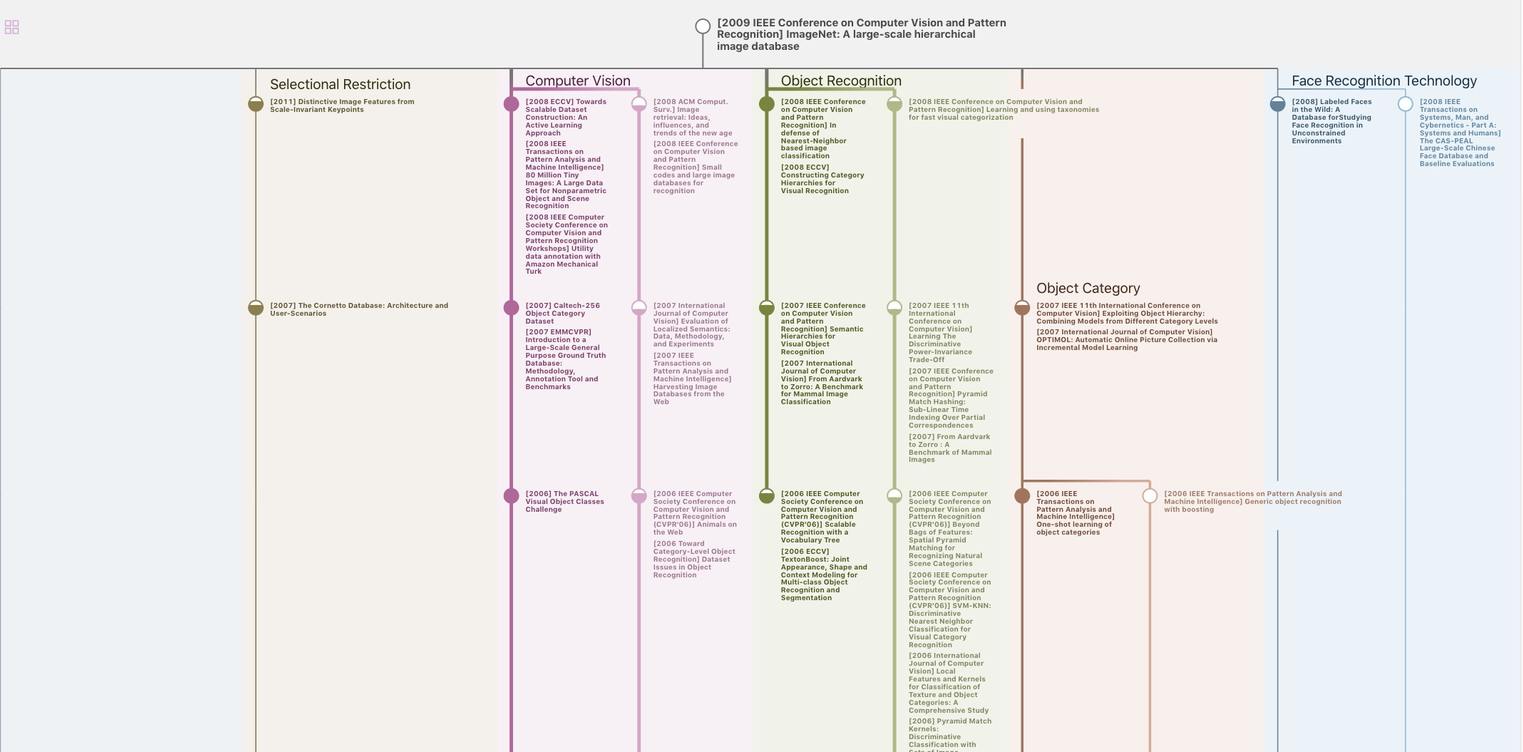
生成溯源树,研究论文发展脉络
Chat Paper
正在生成论文摘要