Adaptive Model-updated Correlation Filters for Real-time Visual Tracking
Proceedings of the 2019 International Conference on Artificial Intelligence and Advanced Manufacturing(2019)
摘要
The Correlation Filters has excellent performance in the field of visual tracking. However, most of the model-updated methods adopt the linear interpolation with a fixed learning rate. Although the tracked object information can be retained in a period of time, it is easy to learn the background information into the model due to a fixed learning rate. In this paper, a new adaptive Model-updated method is proposed. Firstly, the similarity metric method is used to construct the confidence function, and then the high confidence prediction image frame is used to construct the discriminant dataset. Finally, the adaptive learning rate is computed according to the confidence value of the predicted image. Perform an adaptive update of the model. The experimental results show that the proposed algorithm can be well embedded in the correlation filters tracker. The proposed method is embedded in the KCF and Staple algorithms and tested on three challenging benchmark datasets OTB2013, OTB100 and TC128, and the tracking performance is improved.
更多查看译文
关键词
adaptive model-update, correlation filters, discriminant dataset, similarity metric, visual tracking
AI 理解论文
溯源树
样例
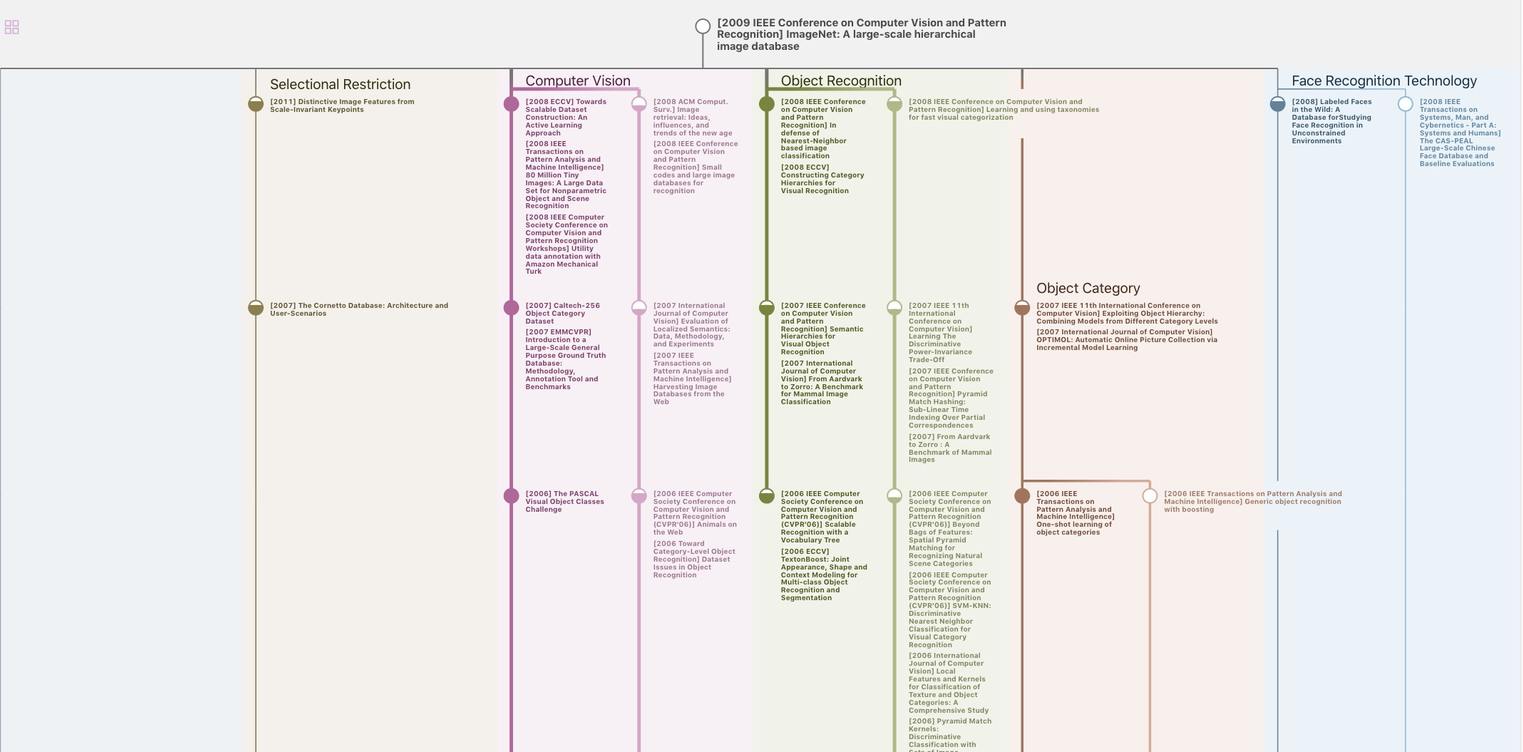
生成溯源树,研究论文发展脉络
Chat Paper
正在生成论文摘要