A scalable sparse Cholesky based approach for learning high-dimensional covariance matrices in ordered data
Machine Learning(2019)
摘要
Covariance estimation for high-dimensional datasets is a fundamental problem in machine learning, and has numerous applications. In these high-dimensional settings the number of features or variables p is typically larger than the sample size n . A popular way of tackling this challenge is to induce sparsity in the covariance matrix, its inverse or a relevant transformation. In many applications, the data come with a natural ordering. In such settings, methods inducing sparsity in the Cholesky parameter of the inverse covariance matrix can be quite useful. Such methods are also better positioned to yield a positive definite estimate of the covariance matrix, a critical requirement for several downstream applications. Despite some important advances in this area, a principled approach to general sparse-Cholesky based covariance estimation with both statistical and algorithmic convergence safeguards has been elusive. In particular, the two popular likelihood based methods proposed in the literature either do not lead to a well-defined estimator in high-dimensional settings, or consider only a restrictive class of models. In this paper, we propose a principled and general method for sparse-Cholesky based covariance estimation that aims to overcome some of the shortcomings of current methods, but retains their respective strengths. We obtain a jointly convex formulation for our objective function, and show that it leads to rigorous convergence guarantees and well-defined estimators, even when p > n . Very importantly, the approach always leads to a positive definite and symmetric estimator of the covariance matrix. We establish both high-dimensional estimation and selection consistency, and also demonstrate excellent finite sample performance on simulated/real data.
更多查看译文
关键词
Covariance estimation, High-dimensional data, Sparse Cholesky, Penalized likelihood
AI 理解论文
溯源树
样例
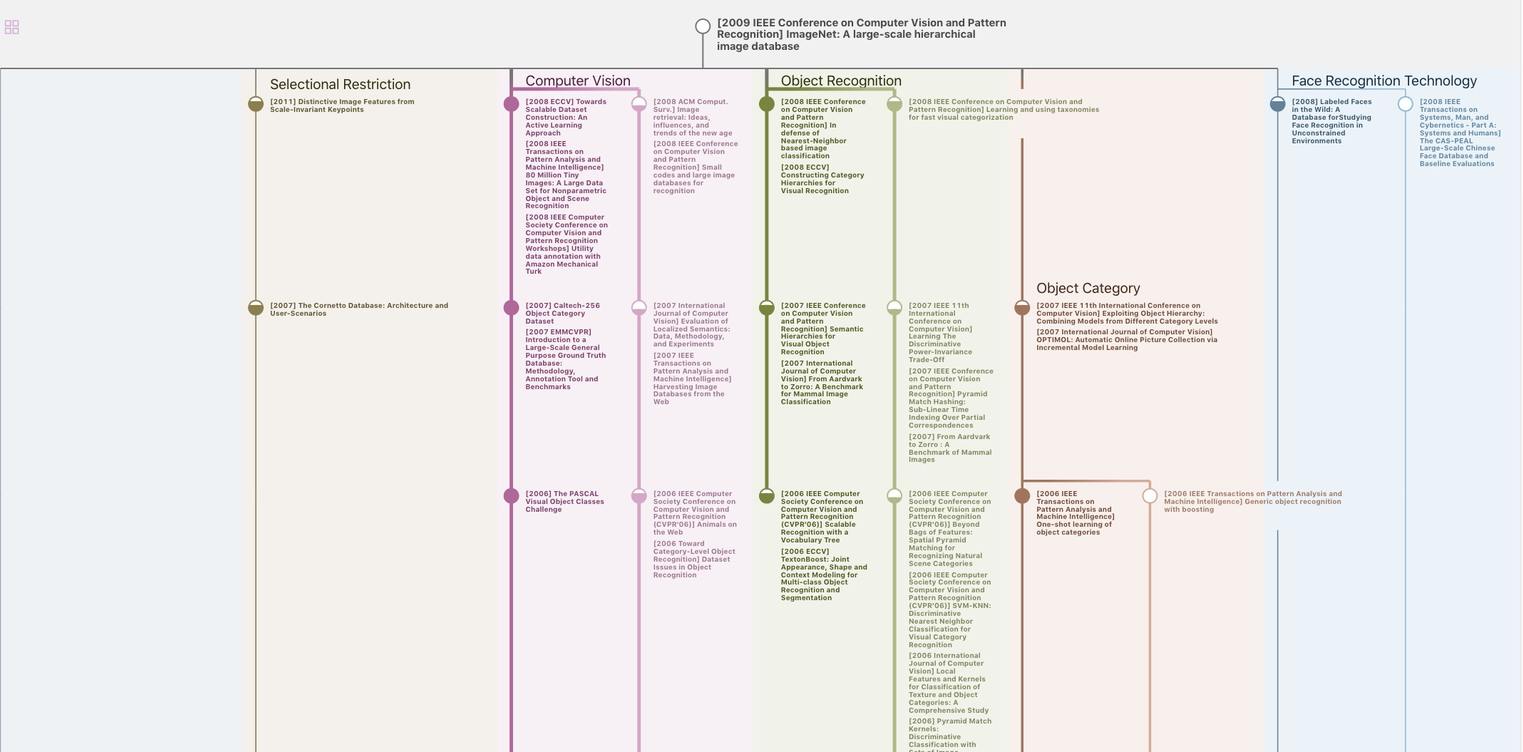
生成溯源树,研究论文发展脉络
Chat Paper
正在生成论文摘要