A fog computing framework for autonomous driving assist: architecture, experiments, and challenges
Proceedings of the 29th Annual International Conference on Computer Science and Software Engineering(2019)
Abstract
Autonomous driving is expected to provide a range of far-reaching economic, environmental and safety benefits. In this study, we propose a fog computing based framework to assist autonomous driving. Our framework relies on overhead views from cameras and data streams from vehicle sensors to create a network of distributed digital twins, called an edge twin, on fog machines. The edge twin will be continuously updated with the locations of both autonomous and human-piloted vehicles on the road segments. The vehicle locations will be harvested from overhead cameras as well as location feeds from the vehicles themselves. Although the edge twin can make fair road space allocations from a global viewpoint, there is a communication cost (delay) in reaching it from the cameras and vehicular sensors. To address this, we introduce a machine learning forecaster as a part of the edge twin which is responsible for predicting the future location of vehicles. Lastly, we introduce a box algorithm that will use the forecasted values to create a hazard map for the road segment which would be used by the framework to suggest safe manoeuvres for the autonomous vehicles such as lane changes and accelerations. We present the complete fog computing framework for autonomous driving assist and evaluate key portions of the proposed framework using simulations based on a real-world dataset of vehicle position traces on a highway.
MoreTranslated text
Key words
autonomous driving, edge twin, fog computing, machine learning
AI Read Science
Must-Reading Tree
Example
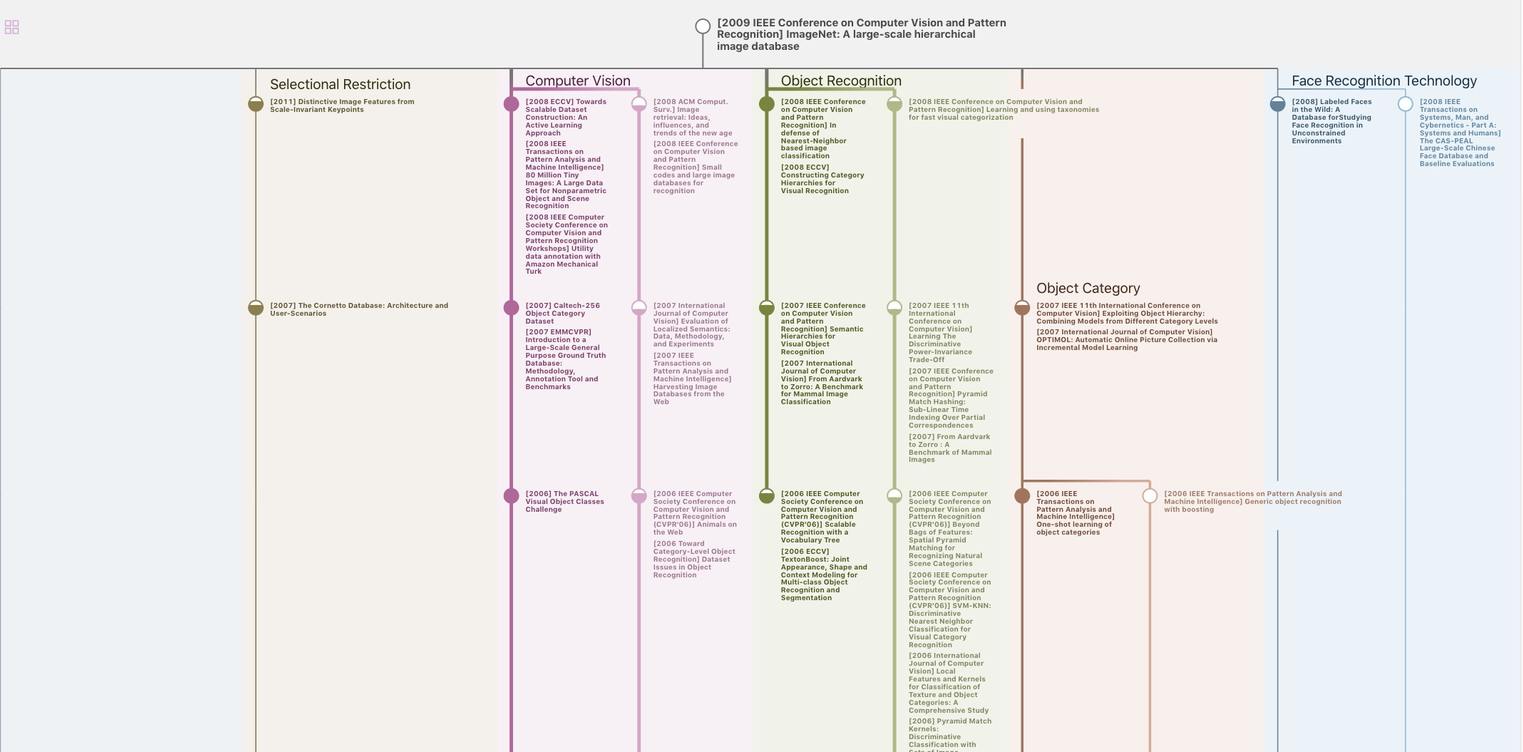
Generate MRT to find the research sequence of this paper
Chat Paper
Summary is being generated by the instructions you defined