Detecting distant-homology protein structures by aligning deep neural-network based contact maps.
PLOS COMPUTATIONAL BIOLOGY(2019)
摘要
Accurate prediction of atomic-level protein structure is important for annotating the biological functions of protein molecules and for designing new compounds to regulate the functions. Template-based modeling (TBM), which aims to construct structural models by copying and refining the structural frameworks of other known proteins, remains the most accurate method for protein structure prediction. Due to the difficulty in recognizing distant-homology templates, however, the accuracy of TBM decreases rapidly when the evolutionary relationship between the query and template vanishes. In this study, we propose a new method, CEthreader, which first predicts residue-residue contacts by coupling evolutionary precision matrices with deep residual convolutional neural-networks. The predicted contact maps are then integrated with sequence profile alignments to recognize structural templates from the PDB. The method was tested on two independent benchmark sets consisting collectively of 1,153 non-homologous protein targets, where CEthreader detected 176% or 36% more correct templates with a TM-score >0.5 than the best state-of-the-art profile- or contact-based threading methods, respectively, for the Hard targets that lacked homologous templates. Moreover, CEthreader was able to identify 114% or 20% more correct templates with the same Fold as the query, after excluding structures from the same SCOPe Superfamily, than the best profile- or contact-based threading methods. Detailed analyses show that the major advantage of CEthreader lies in the efficient coupling of contact maps with profile alignments, which helps recognize global fold of protein structures when the homologous relationship between the query and template is weak. These results demonstrate an efficient new strategy to combine ab initio contact map prediction with profile alignments to significantly improve the accuracy of template-based structure prediction, especially for distant-homology proteins. Author summary Despite decades of effort in computational method development, template-based modeling (TBM) still remains the most reliable approach to high-resolution protein structure prediction. Previous studies have shown that the PDB library is complete for single-domain proteins and TBM is in principle sufficient to solve the structure prediction problem if the most similar structure in the PDB could be reliably identified and used as template for model reconstruction. But in reality, the success of TBM depends on the availability of closely-homologous templates, where its accuracy and reliability decrease sharply when the evolutionary relationship between query and template becomes more distant. We developed a new threading approach, CEthreader, which allows for dynamic programing alignments of predicted contact-maps through eigen-decomposition. The large-scale benchmark tests show that the coupling of contact map with profile and secondary structure alignments through the proposed protocol can significantly improve the accuracy of template recognition for distantly-homologous protein targets.
更多查看译文
AI 理解论文
溯源树
样例
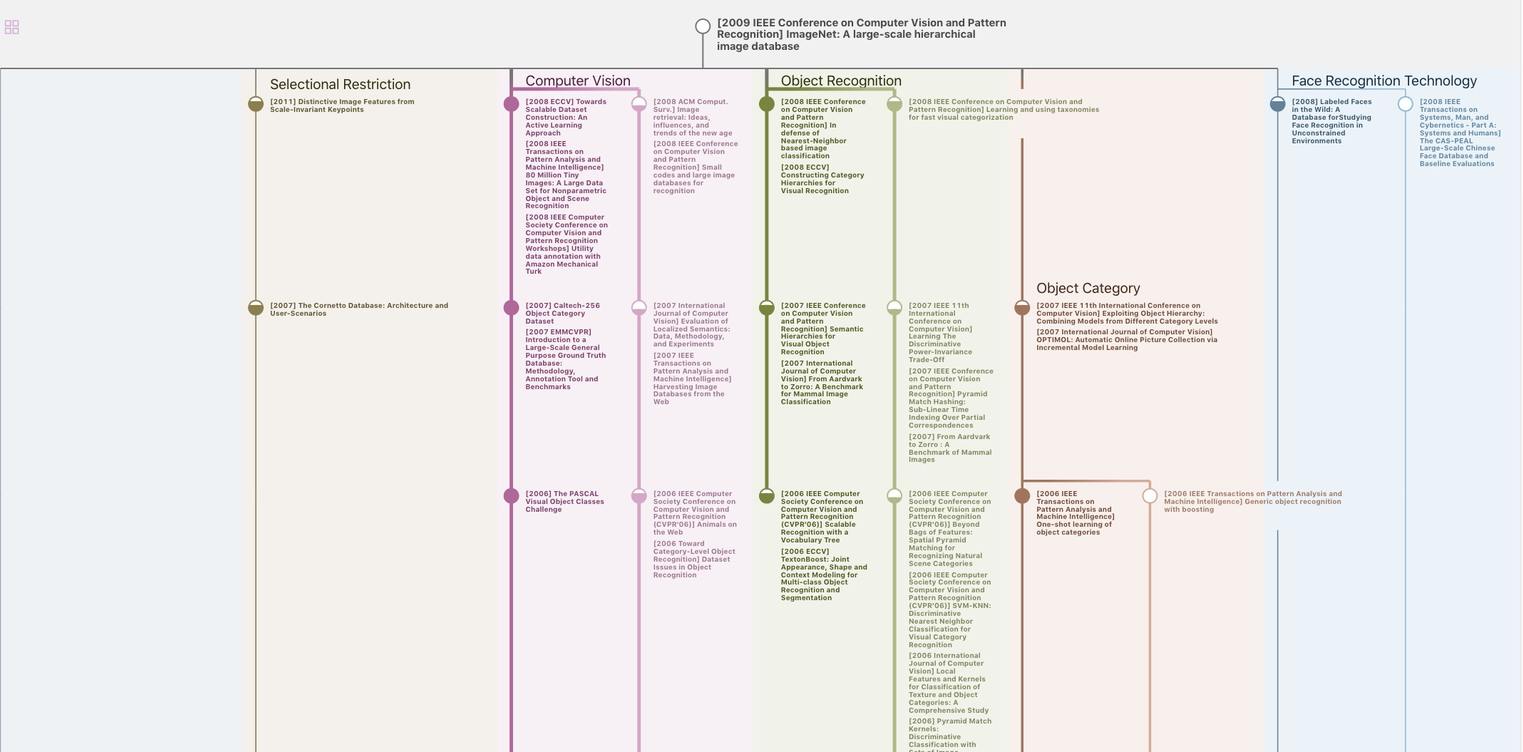
生成溯源树,研究论文发展脉络
Chat Paper
正在生成论文摘要