Training DNN IoT Applications for Deployment On Analog NVM Crossbars
2020 INTERNATIONAL JOINT CONFERENCE ON NEURAL NETWORKS (IJCNN)(2020)
摘要
A trend towards energy-efficiency, security and privacy has led to a recent focus on deploying deep-neural networks (DNN) on microcontrollers. However, limits on compute and memory resources restrict the size and the complexity of the machine-learning (ML) models deployable in these systems. Computation-In-Memory architectures based on resistive non-volatile memory (NVM) technologies hold great promise of satisfying the compute and memory demands of high-performance and low-power, inherent in modern DNNs. Nevertheless, these technologies are still immature and suffer from both the intrinsic analog-domain noise problems and the inability of representing negative weights in the NVM structures, incurring in larger crossbar sizes with concomitant impact on Analog-to-Digital Converters (ADCs) and Digital-to-Analog Converters (DACs). In this paper, we provide a training framework for addressing these challenges and quantitatively evaluate the circuit-level efficiency gains thus accrued. We make two contributions: Firstly, we propose a training algorithm that eliminates the need for tuning individual layers of a DNN ensuring uniformity across layer-weights and activations. This ensures analog-blocks that can be reused and peripheral hardware substantially reduced. Secondly, using Network Architecture Search (NAS) methods, we propose the use of unipolar-weighted (either all-positive or all-negative weights) matrices/sub-matrices. Weight unipolarity obviates the need for doubling crossbar area leading to simplified analog periphery. We validate our methodology with CIFAR10 and HAR applications by mapping to crossbars using 4-bit and 2-bit devices. We achieve up to 92.91% accuracy (95% floating-point) using 2-bit only-positive weights for HAR. A combination of the proposed techniques leads to 80% area improvement and up to 45% energy reduction.
更多查看译文
关键词
Deep Neural Networks, DNN, memristor, RRAM, MRAM, PCRAM
AI 理解论文
溯源树
样例
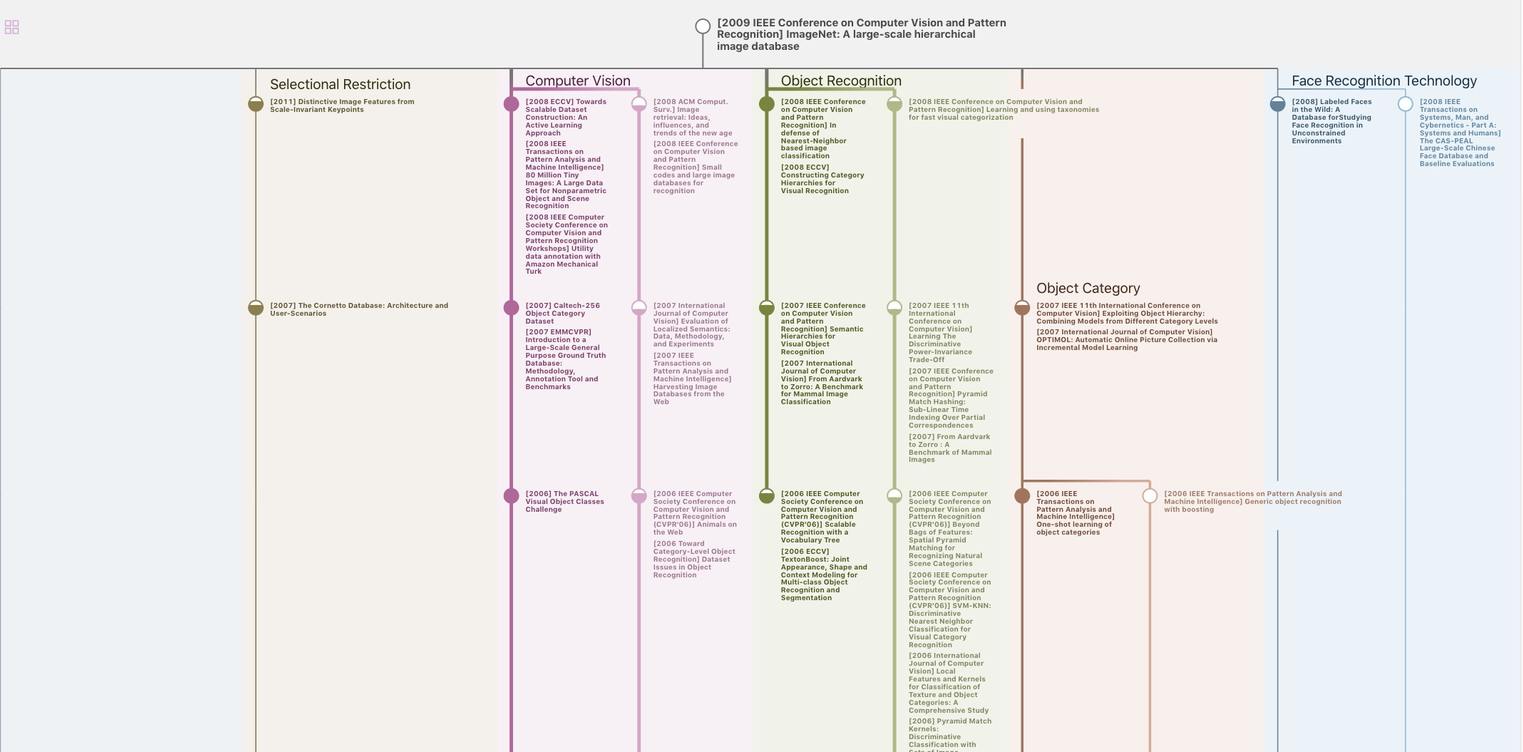
生成溯源树,研究论文发展脉络
Chat Paper
正在生成论文摘要