Deep Learning for Signal Detection in Non-Orthogonal Multiple Access Wireless Systems
2019 UK/ China Emerging Technologies (UCET)(2019)
摘要
This paper presents an initial investigation of deep learning (DL) for multi-user detection in non-orthogonal multiple access (NOMA) wireless systems. In NOMA systems, the successive interference cancellation (SIC) process is usually performed at the receiver, where multiple users are decoded in a sequential fashion. Due to error propagation effects, the detection accuracy will largely depend on the correct detection of previous users. A DL-based NOMA receiver is designed to decode messages for multiple users in a one-shot process, without estimating channels explicitly. The DL-based NOMA receiver is represented by a deep neural network (DNN), which performs channel estimation and signal detection in a joint manner. The DNN is first trained offline using simulation data based on channel statistics and then used to recover the transmitted symbols directly in the online deployment stage. Initial results show that the DL approach can outperform the conventional pilot-based channel estimation methods and is more robust to the number of pilot symbols. The DNN is shown to be capable of mitigating the potential error propagation effects that occur in the SIC detector. Furthermore, when the inter-symbol interference is severe, the DL approach can achieve better performance than a maximum likelihood detector that does not account for interference effects.
更多查看译文
关键词
Non-orthogonal multiple access,deep learning,channel estimation,signal detection,MATLAB
AI 理解论文
溯源树
样例
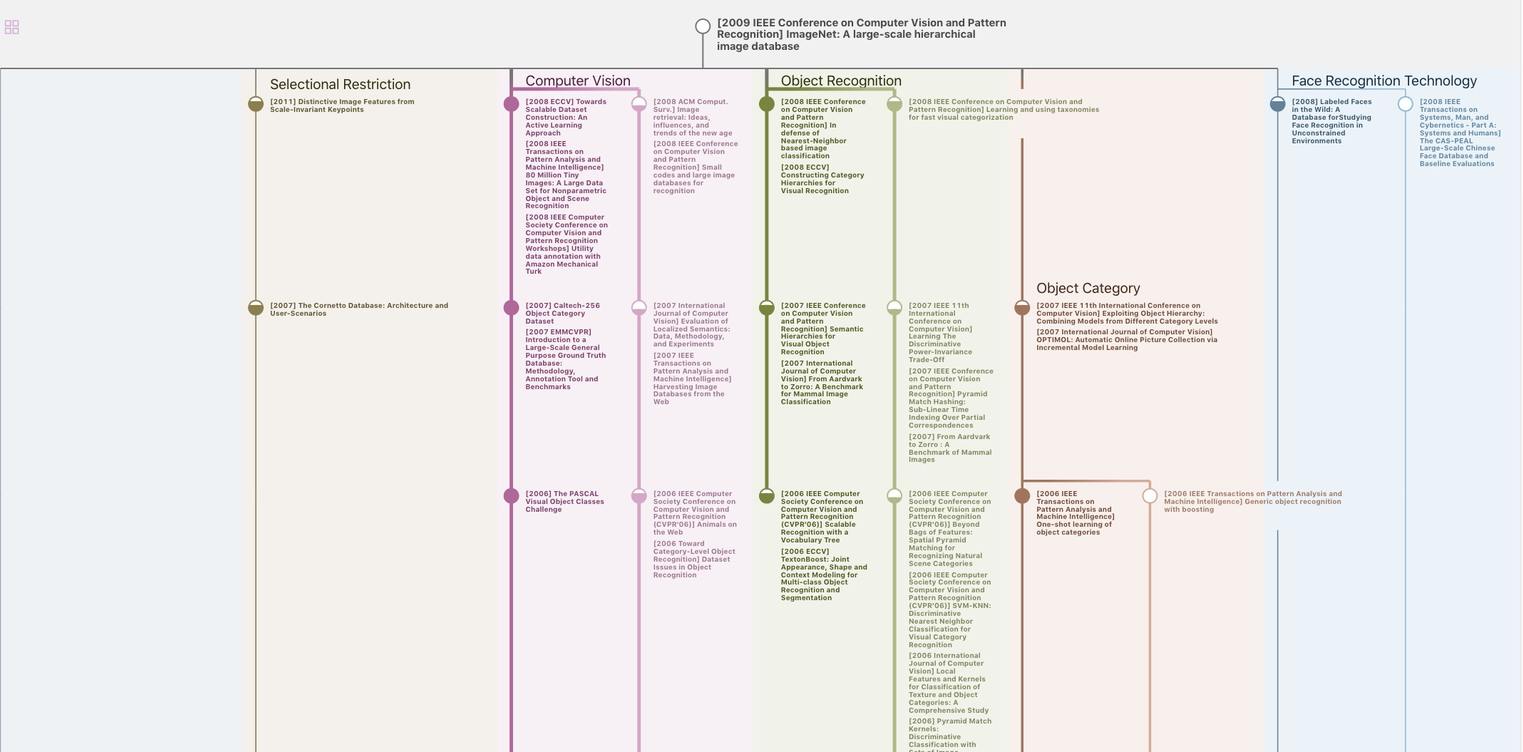
生成溯源树,研究论文发展脉络
Chat Paper
正在生成论文摘要