Vcos: A Novel Synergistic Oversampling Algorithm In Binary Imbalance Classification
IEEE ACCESS(2019)
摘要
Learning from class-imbalanced data is a challenging problem as standard classification algorithms are designed to handle balanced class distributions. Scholars solve this problem by modifying classifiers or and generating artificial data by oversampling. The former usually design corresponding classifier to adapt them to the imbalanced data, while the latter exploits the sampling algorithm, which are the data preprocessing steps independent of the classifier. In this paper, we propose a novel synergistic oversampling algorithm to combine the oversampling and classification into one without training the classifier repeatedly, which can generate new pertinent samples according to the classification performance of the classifier without repeat training or deep understanding of the classifier, so the generated samples can guarantee the performance improvement of the classifier. Moreover, The proposed framework enclosures the oversampling method without traditional parameters in oversampling methods. Experimental results on several real-life imbalanced datasets demonstrate the effectiveness and efficiency of the proposed algorithm in binary classification problems.
更多查看译文
关键词
Imbalanced classification,oversampling algorithm,variational auto-encoder,synergistic architecture,expected classifier
AI 理解论文
溯源树
样例
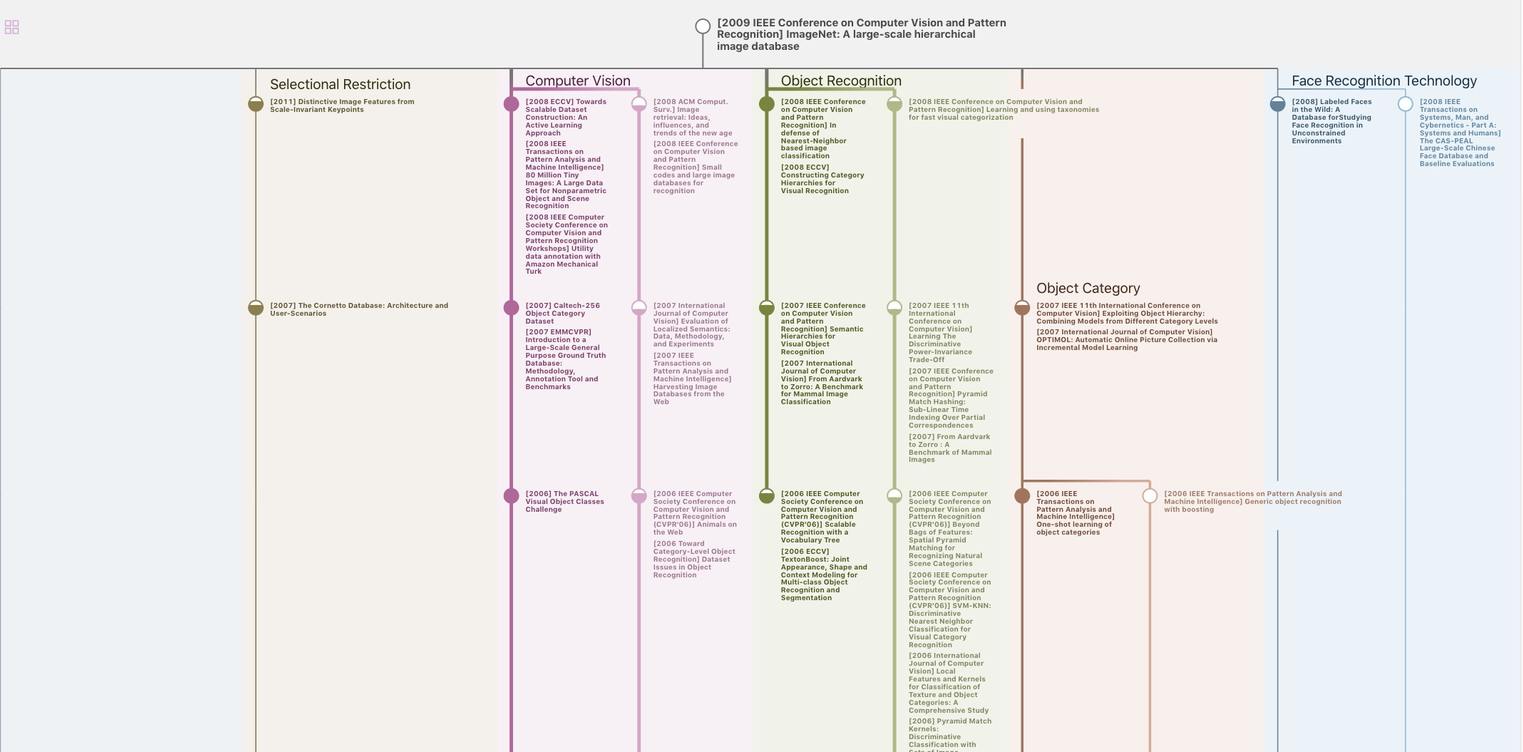
生成溯源树,研究论文发展脉络
Chat Paper
正在生成论文摘要