An ensemble method for inverse reinforcement learning
Information Sciences(2020)
摘要
In inverse reinforcement learning (IRL), a reward function is learnt to generalize experts’ behavior. This paper proposes a model-free IRL algorithm based on an ensemble method, where the reward function is regarded as a parametric function of expected features. In other words, the parameters are updated based on a weak classification method. The IRL is formulated as a problem of a boosting classifier, akin to the renowned Adaboost algorithm for classification, feature expectations from experts’ demonstration, and the trajectory induced by an agent's current policy. The proposed approach takes individual feature expectation as attractor or expeller, depending on the sign of the residuals of the state trajectories between expert's demonstration and the one induced by RL with the currently approximated reward function, so as to tackle its central challenges of accurate inference, generalizability, and correctness of prior knowledge. Then, the proposed method is applied further to approximate an abstract reward function from observations of more complex behavior composed of several basic actions. The results of the simulations in a labyrinth are shown to validate the proposed algorithm. Furthermore, behaviors composed of a set of primitive actions on a soccer robot field are examined for the applicability of the proposed method.
更多查看译文
关键词
Apprentice learning,Inverse reinforcement learning,Q-learning,Boosting classifier
AI 理解论文
溯源树
样例
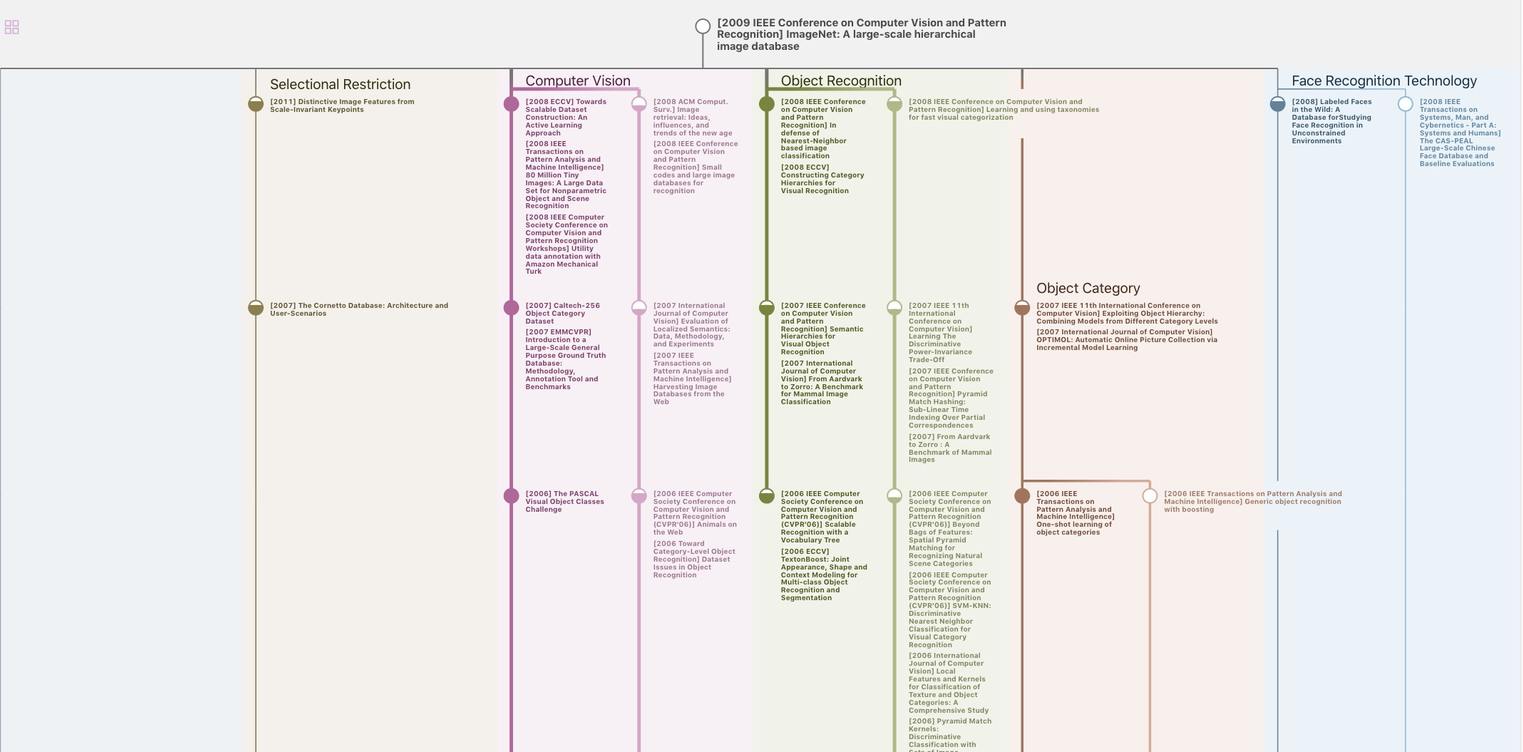
生成溯源树,研究论文发展脉络
Chat Paper
正在生成论文摘要