A Real-Time ATC Safety Monitoring Framework Using a Deep Learning Approach
IEEE Transactions on Intelligent Transportation Systems(2020)
摘要
A deep learning-based safety monitoring framework for air traffic control (ATC) systems is proposed in this paper to reduce human errors and relieve the controllers' workload by regulating the controlling procedure, eliminating communication misunderstanding, monitoring flight conformance, and detecting potential conflicts. The framework comprises automatic speech recognition (ASR), controlling intent inference (CII), and control safety monitoring (CSM) subsystems. The pipeline of the proposed framework can be described as follows: the ASR subsystem translates the pilot-controller voice communications (PCVCs) into texts, which are then converted to the predefined data structure by the CII subsystem. Three types of air traffic safety measures, including repetition check, flight conformance verification, and potential conflict detection, are finally validated by the CSM subsystem. An improved end-to-end ASR model with convolutional, bidirectional long short-term memory (BLSTM) and fully connected (FC) layers is trained using the connectionist temporal classification loss function. The BLSTM and FC combined CII model is designed to infer the controlling intent and slot filling. A language model is also trained in this subsystem to improve the overall performance of the framework. After converting the PCVCs to ATC data, the CSM subsystem checks the given safety monitoring tasks and sends warnings to the current system. The experimental results show that the proposed ASR model obtains a better performance than that of other approaches, and the tasks in the CII subsystem are fulfilled with a high classification precision. The CSM subsystem is also tested to confirm its safety monitoring function by playing back the data and several simulated instructions. To the best of our knowledge, this is pioneering work in the safety monitoring of flight control by recognizing the PCVCs with deep learning-based methods.
更多查看译文
关键词
Automatic speech recognition,pilot-controller voice communications,controlling intent inference,slot filling,control safety monitoring
AI 理解论文
溯源树
样例
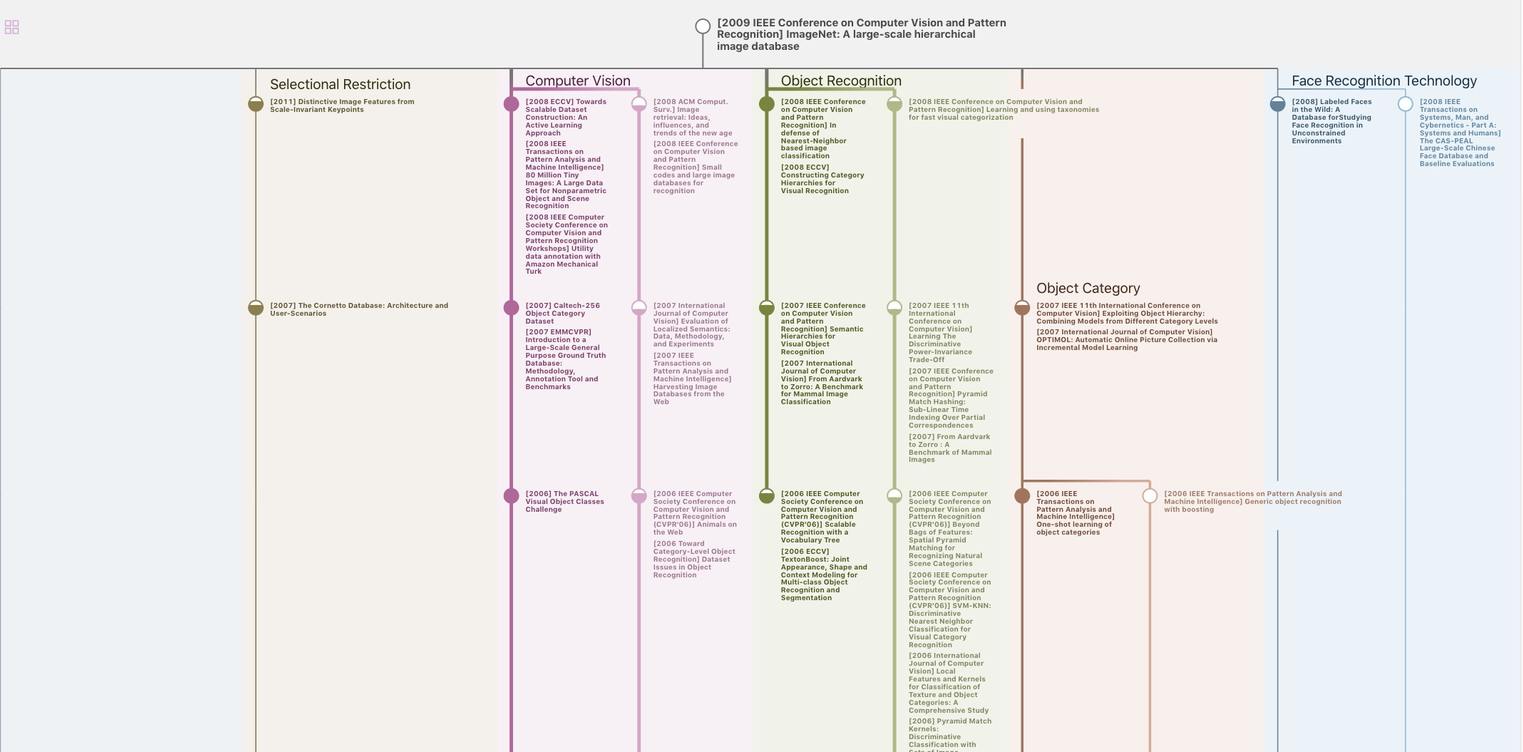
生成溯源树,研究论文发展脉络
Chat Paper
正在生成论文摘要