LOW-RANK MATRIX DECOMPOSITION METHOD FOR POTENTIAL FIELD DATA SEPARATION
GEOPHYSICS(2020)
摘要
Separation of potential field data forms the basis of inversion and interpretation. The low-rank matrix theory is used for the separation of potential field data. A theoretical analysis led to the approximate equation that demonstrates the relation between the amplitudes of the wavenumber components of potential field data and the singular values of the trajectory matrix embedded from the potential field data matrix. Therefore, the low-rank feature of the trajectory matrix of regional field data and the sparse feature of the trajectory matrix of residual field data can be obtained based on the features of the power spectrum of the potential field data. Based on this, we have developed a low-rank matrix decomposition model for the separation of the trajectory matrix of the potential field data. Minimizing the rank of the trajectory matrix of the regional field data and the l(0)-norm of the trajectory matrix of the residual field data is a double-objective optimization task, and this optimization task can be solved by the inexact augmented Lagrange multiplier algorithm. The weighting parameter is robust and easy to set. Numerical experiment results indicate that our method is robust, and the separation errors of the method are smaller compared to the matched filtering and wavelet analysis methods. In addition, our method can be easily implemented and has clear geophysical significance. Finally, our method is applied on real data sets in the Daye area, Hubei Province, China. The separated gravity and magnetic fields coincide well with target geologic sources.
更多查看译文
关键词
decomposition,low-rank
AI 理解论文
溯源树
样例
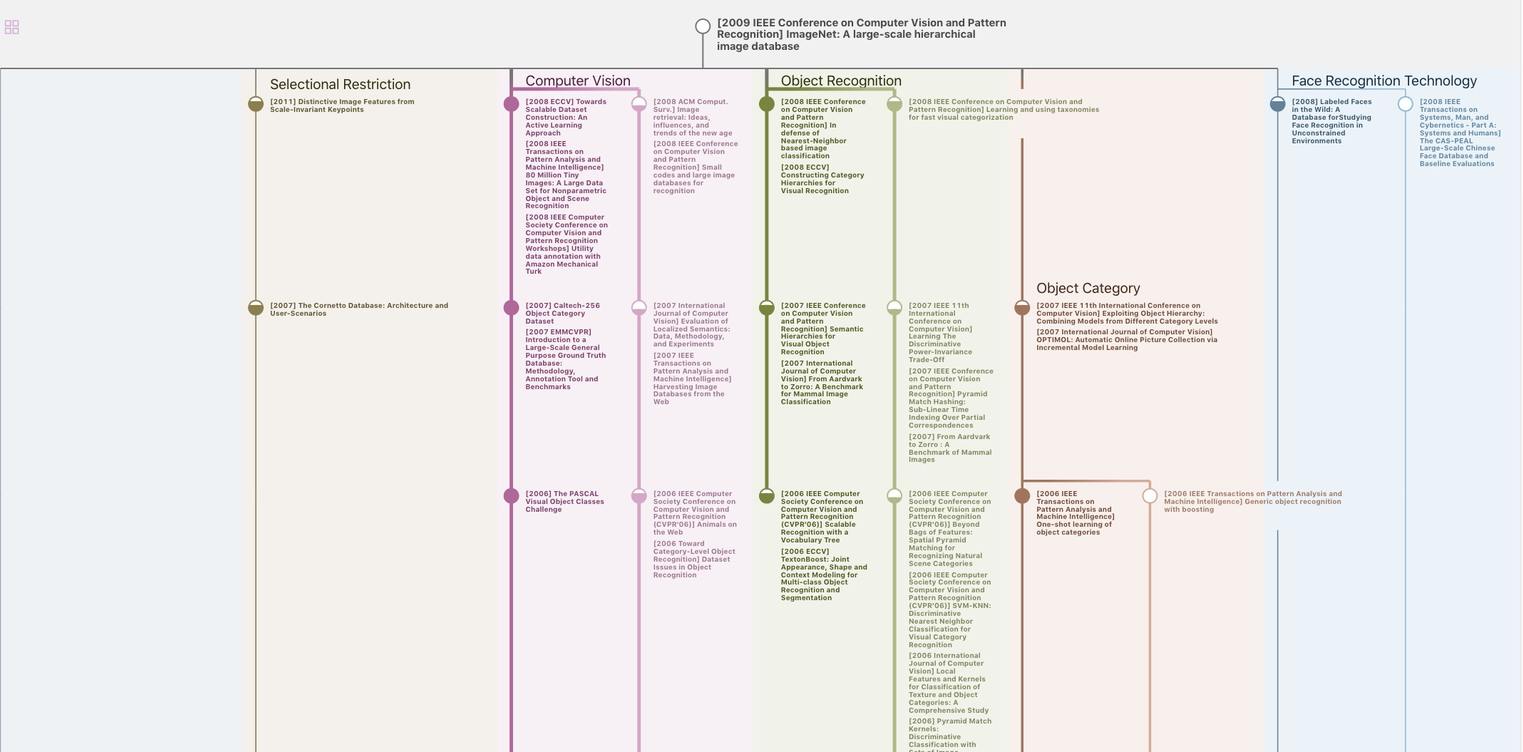
生成溯源树,研究论文发展脉络
Chat Paper
正在生成论文摘要