Binary Classification Rule Generation From Decomposed Data
INTERNATIONAL JOURNAL OF INTELLIGENT SYSTEMS(2019)
摘要
Learning classification rules from data that do not fit in the available memory is a challenging task. The goal of this study is to develop an approach for generating binary classification rules from decomposed data that are equivalent in terms of quality to those found over the whole data. In the proposed approach, each class is divided into the same arbitrary small number of subtables. For each pair of subsets from different classes, rule sets are induced using any sequential covering algorithm. Rule sets generated from the same positive class subset and different negative class subsets are merged using an operator constructed on the basis of Cartesian product and conjunction operators. The rule sets obtained in this way are joined into one set. During the rule merging, unnecessary rules are removed. It is proven that for training data, the quality of the rule set generated using the approach is the same as that for the whole data. It is experimentally verified that for test data, the quality of classification is comparable with that obtained using a nondecomposed data approach.
更多查看译文
关键词
classification rule generation, data decomposition, rule merging, sequential covering algorithm
AI 理解论文
溯源树
样例
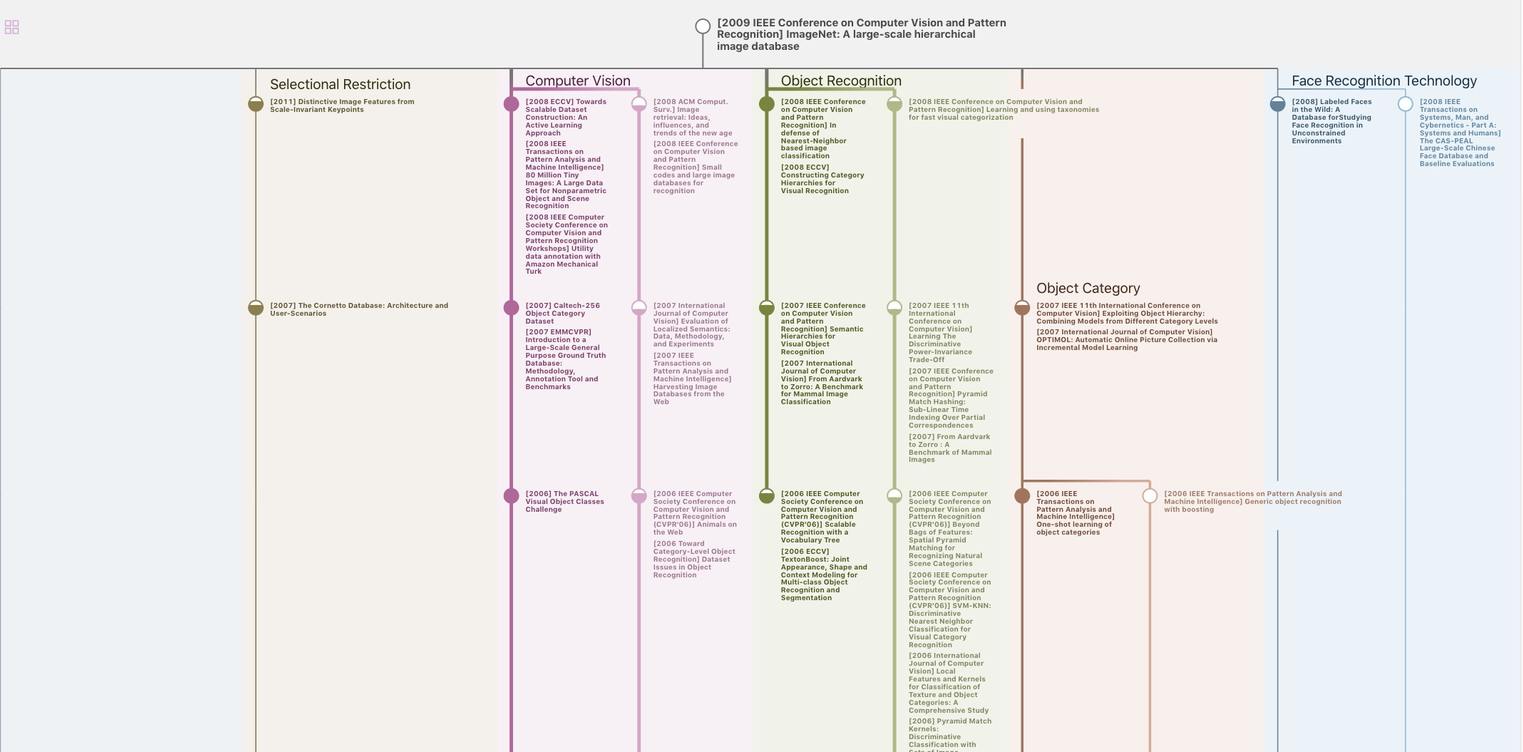
生成溯源树,研究论文发展脉络
Chat Paper
正在生成论文摘要