Deep learning acceleration of Total Lagrangian Explicit Dynamics for soft tissue mechanics
Computer Methods in Applied Mechanics and Engineering(2020)
摘要
Simulating complex soft tissue deformations has been an intense research area in the fields of computer graphics or computational physiology for instance. A desired property is the ability to perform fast, if not real-time, simulations while being physically accurate. Numerical schemes have been explored to speed up finite element methods, like the Total Lagrangian Explicit Dynamics (TLED). However, real-time applications still come at the price of accuracy and fidelity. In this work, we explore the use of neural networks as function approximators to accelerate the time integration of TLED, while being generic enough to handle various geometries, motion and materials without having to retrain the neural network model. The method is evaluated on a set of experiment, showing promising accuracy at time steps up to 20 times larger than the “breaking” time step, as well as in a simple medical application. Such an approach could pave the way to very fast but accurate acceleration strategies for computational biomechanics.
更多查看译文
关键词
Data-driven computational modeling,Soft tissue biomechanics,Deep learning,Finite element method,Total Lagrangian Explicit Dynamics
AI 理解论文
溯源树
样例
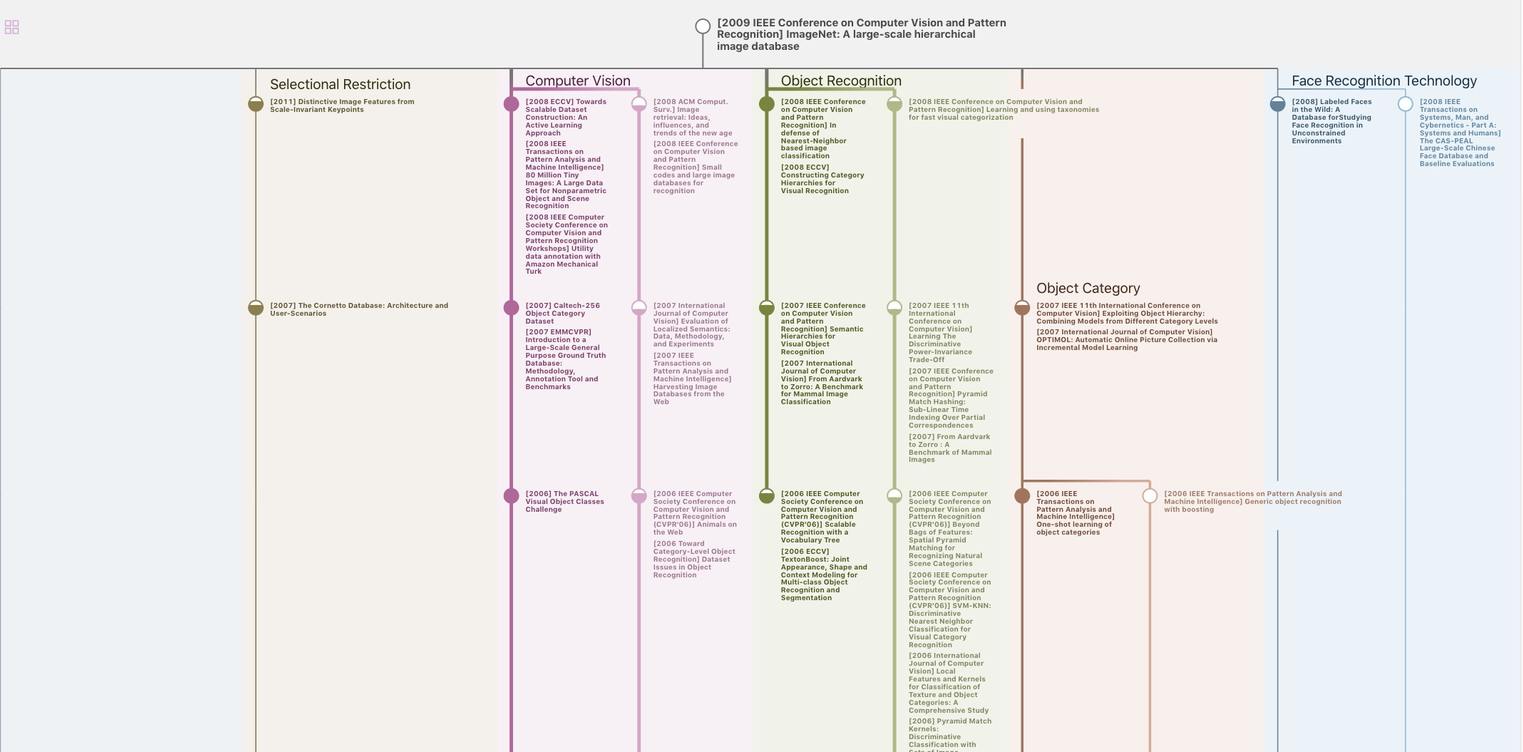
生成溯源树,研究论文发展脉络
Chat Paper
正在生成论文摘要