Plant-part segmentation using deep learning and multi-view vision
Biosystems Engineering(2019)
摘要
To accelerate the understanding of the relationship between genotype and phenotype, plant scientists and plant breeders are looking for more advanced phenotyping systems that provide more detailed phenotypic information about plants. Most current systems provide information on the whole-plant level and not on the level of specific plant parts such as leaves, nodes and stems. Computer vision provides possibilities to extract information from plant parts from images. However, the segmentation of plant parts is a challenging problem, due to the inherent variation in appearance and shape of natural objects. In this paper, deep-learning methods are proposed to deal with this variation. Moreover, a multi-view approach is taken that allows the integration of information from the two-dimensional (2D) images into a three-dimensional (3D) point-cloud model of the plant. Specifically, a fully convolutional network (FCN) and a masked R-CNN (region-based convolutional neural network) were used for semantic and instance segmentation on the 2D images. The different viewpoints were then combined to segment the 3D point cloud. The performance of the 2D and multi-view approaches was evaluated on tomato seedling plants. Our results show that the integration of information in 3D outperforms the 2D approach, because errors in 2D are not persistent for the different viewpoints and can therefore be overcome in 3D. (C) 2019 IAgrE. Published by Elsevier Ltd. All rights reserved.
更多查看译文
关键词
digital plant phenotyping,2D images and 3D point clouds,semantic segmentation,instance segmentation
AI 理解论文
溯源树
样例
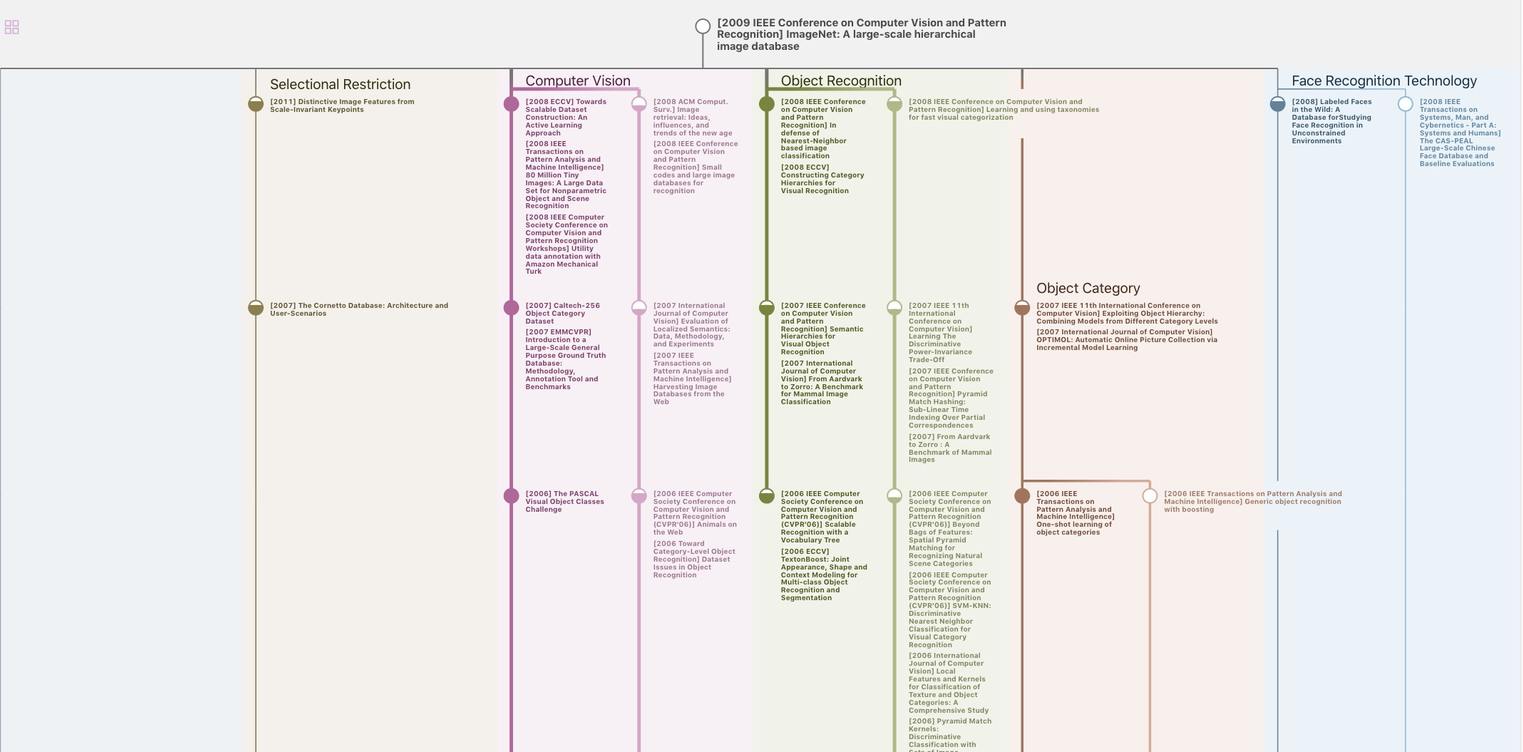
生成溯源树,研究论文发展脉络
Chat Paper
正在生成论文摘要