Deep-Learning Approach To The Structure Of Amorphous Silicon
PHYSICAL REVIEW B(2019)
摘要
We present a deep-learning approach for modeling the atomic structure of amorphous silicon (a-Si). While accurate models of disordered systems require an ab initio description of the energy landscape which severely limits the attainable system size, large-scale models rely on empirical potentials, at the price of reduced reliability and a computational load that is still restricting for many purposes. In this paper, we explore an approach based on deep learning, particularly generative modeling that could reconcile both requirements of accuracy and efficiency by learning structural features from data. When trained on a set of observations, such models can generate new structures very efficiently with the desired level of accuracy, as determined by the data set. We first validate our approach by training a convolutional neural network to approximate the potential-energy surface of a-Si, as given by the Stillinger-Weber potential, which results in a root-mean-square error of 5.05 meV per atom-about 0.16% of the atomic energy. We then train a deep generative model, the Wasserstein autoencoder, for the generation of a-Si configurations. Our approach leads to models which exhibit some of the essential features of a-Si while possessing too much structural disorder, thus suggesting that the method is viable; we indicate avenues for improving it towards the generation of state-of-the-art structures.
更多查看译文
关键词
silicon,deep-learning deep-learning,deep-learning deep-learning approach
AI 理解论文
溯源树
样例
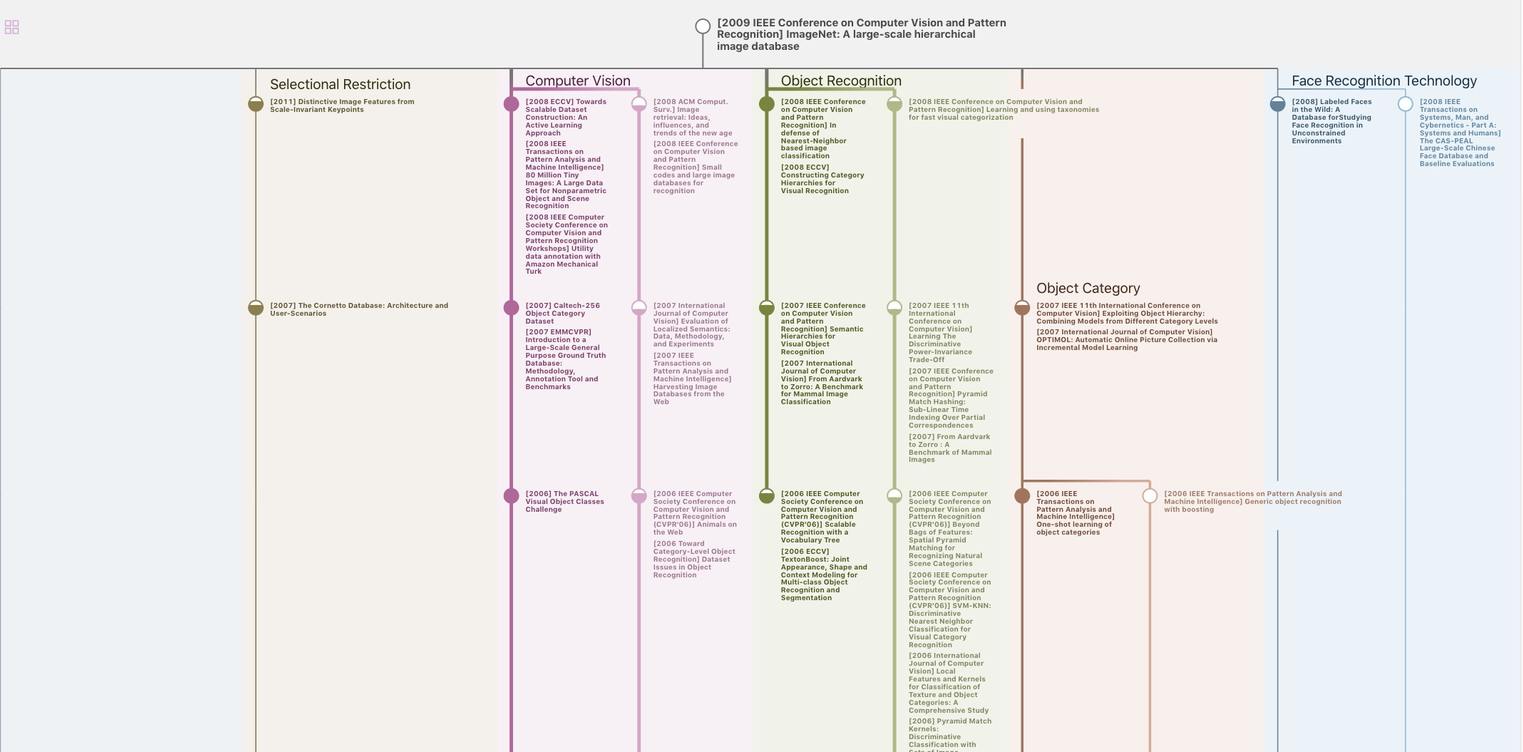
生成溯源树,研究论文发展脉络
Chat Paper
正在生成论文摘要