Machine Learning To Predict Final Fire Size At The Time Of Ignition
INTERNATIONAL JOURNAL OF WILDLAND FIRE(2019)
摘要
Fires in boreal forests of Alaska are changing, threatening human health and ecosystems. Given expected increases in fire activity with climate warming, insight into the controls on fire size from the time of ignition is necessary. Such insight may be increasingly useful for fire management, especially in cases where many ignitions occur in a short time period. Here we investigated the controls and predictability of final fire size at the time of ignition. Using decision trees, we show that ignitions can be classified as leading to small, medium or large fires with 50.4 +/- 5.2% accuracy. This was accomplished using two variables: vapour pressure deficit and the fraction of spruce cover near the ignition point. The model predicted that 40% of ignitions would lead to large fires, and those ultimately accounted for 75% of the total burned area. Other machine learning classification algorithms, including random forests and multi-layer perceptrons, were tested but did not outperform the simpler decision tree model. Applying the model to areas with intensive human management resulted in overprediction of large fires, as expected. This type of simple classification system could offer insight into optimal resource allocation, helping to maintain a historical fire regime and protect Alaskan ecosystems.
更多查看译文
关键词
boreal forests, decision trees, fire management, random forests, vapour pressure deficit
AI 理解论文
溯源树
样例
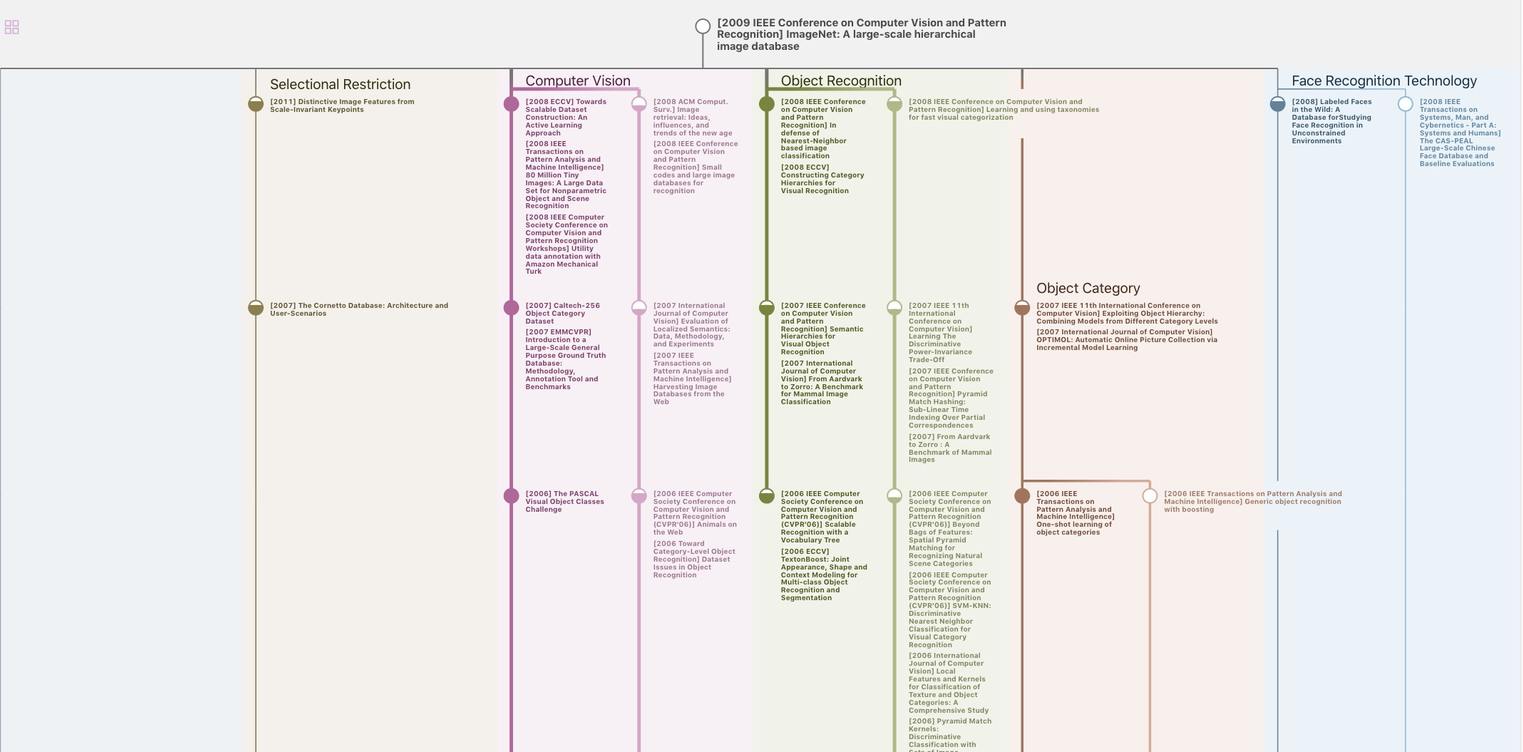
生成溯源树,研究论文发展脉络
Chat Paper
正在生成论文摘要