Performance evaluation of elephant flow predictors in data center networking
Future Generation Computer Systems(2020)
摘要
The dynamic characteristics and unpredictability of the traffic generated by applications and services have given rise to some problems in data center networks (DCN). In particular, the concentration of elephant flows in DCNs can lead to a load imbalance because the equal-cost multi-path (ECMP) routing maps them to the same path. This can result in packet discard, which generates packet re-transmission, causes additional latency, and further degrades link performance. In this paper, we evaluate the performance of different elephant flow predictors with the use of real DCN traces. A traffic time series from a Facebook data center is used to model the elephant flow predictors on a short-term basis and to provide the descriptive statistics and inferences the flows. The paper proposes a hybrid prediction model by combining aspects of the FARIMA and the Recurrent Neural Network (FARIMA-RNN) models. A methodology based on the rank of the prediction accuracy metrics is applied to compare the performance of the hybrid model with the ARIMA, GARCH, RBF, MLP, and LSTM models. The comparison allows the selection of an appropriate model for the implementation of elephant flow scheduling techniques in DCNs. Results show that the FARIMA-RNN model presents lower error rates than the other predictors.
更多查看译文
关键词
Data center networks,Elephant flow,Prediction,Performance evaluation
AI 理解论文
溯源树
样例
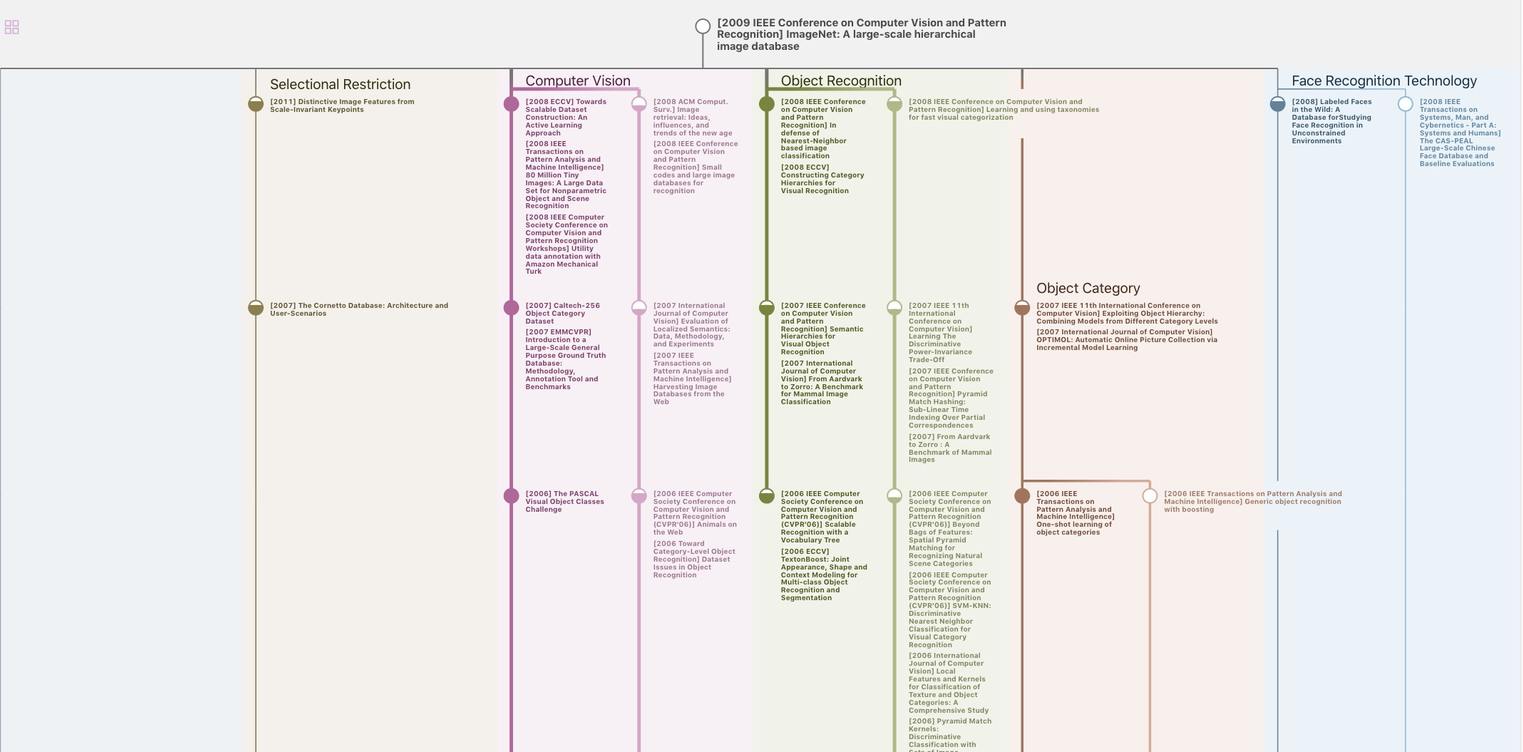
生成溯源树,研究论文发展脉络
Chat Paper
正在生成论文摘要