Rescoring Keyword Search Confidence Estimates with Graph-Based Re-Ranking Using Acoustic Word Embeddings
INTERSPEECH(2019)
摘要
Postprocessing of confidence scores in keyword search (KWS) task is known to be an efficient way of improving retrieval performance. In this paper, we extend the existing graph-based re-ranking algorithm proposed for KWS score calibration. We replace the originally used Dynamic Time Warping (DTW) distance measure between prospective hits with distances between their Acoustic Word Embeddings (AWEs) learned from Neural Networks. We argue that AWEs trained to discriminate between the same and different words should improve the graph-based re-ranking performance. Experimental results on two languages from IARPA Babel program show that our approach outperforms the DTW and improves the baseline KWS result between 3.0 - 7.5% relative on the Maximum Term Weighted Value (MTWV) measure. It was previously shown, that enhancing detection lists with keyword exemplars given high confidence, improved the algorithm performance. We additionally expanded the detection lists with negative query exemplars and observed further improvements in MTWV.
更多查看译文
关键词
keyword search, postprocessing, graph-based re-ranking, acoustic word embedding
AI 理解论文
溯源树
样例
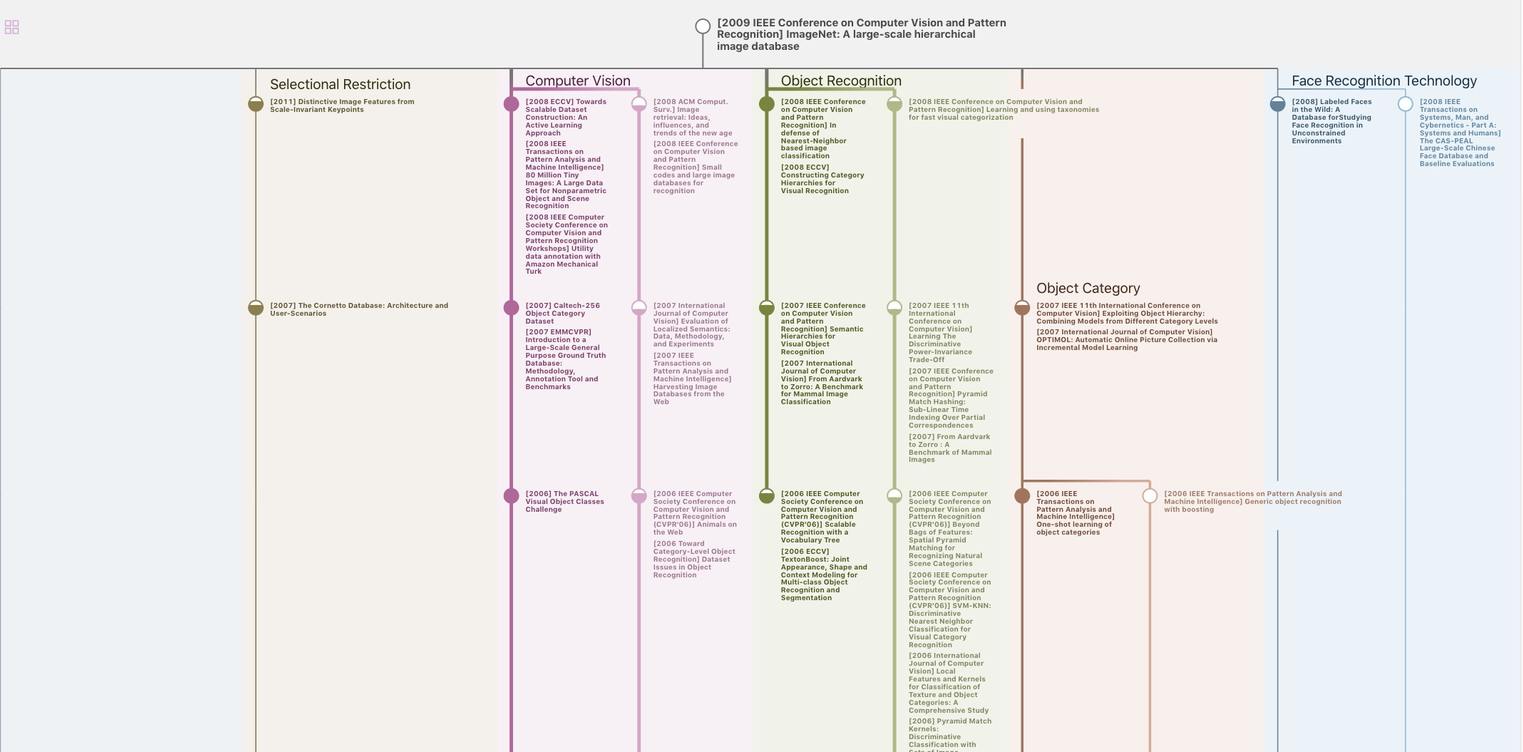
生成溯源树,研究论文发展脉络
Chat Paper
正在生成论文摘要