Hilbert Vector Convolutional Neural Network: 2d Neural Network On 1d Data
ARTIFICIAL NEURAL NETWORKS AND MACHINE LEARNING - ICANN 2019: THEORETICAL NEURAL COMPUTATION, PT I(2019)
摘要
Two-Dimensional Neural Network (2D CNN) has become an alternative method for one-dimensional data classification. Previous studies are focused either only on sequence or vector data. In this paper, we proposed a new 2D CNN classification method that suitable for both, sequence and vector data. The Hilbert space-filling curve was used as a 1D to 2D transfer function in the proposed method. It is used for two reasons: (i) to preserve the spatial locality of 1D data and (ii) to reduce the distance of far-flung data elements. Furthermore, a 1D convolution layer was added in the first stage of our proposed method. It can capture the correlation information of neighboring elements, which is effective for sequence data classification. Consequently, the trainable property of 1D convolutions is very helpful in extracting relevant information for vector data classification. Finally, the performance of the proposed Hilbert Vector Convolutional Neural Network (HVCNN) was compared with two 2D CNN based methods and two non-CNN based methods. Experimental results showed that the proposed HVCNN method delivers better numerical accuracy and generalization property than the other competitive methods. We also did weight distribution analysis to support this claim.
更多查看译文
关键词
Deep learning, Convolutional neural network, Learning representation
AI 理解论文
溯源树
样例
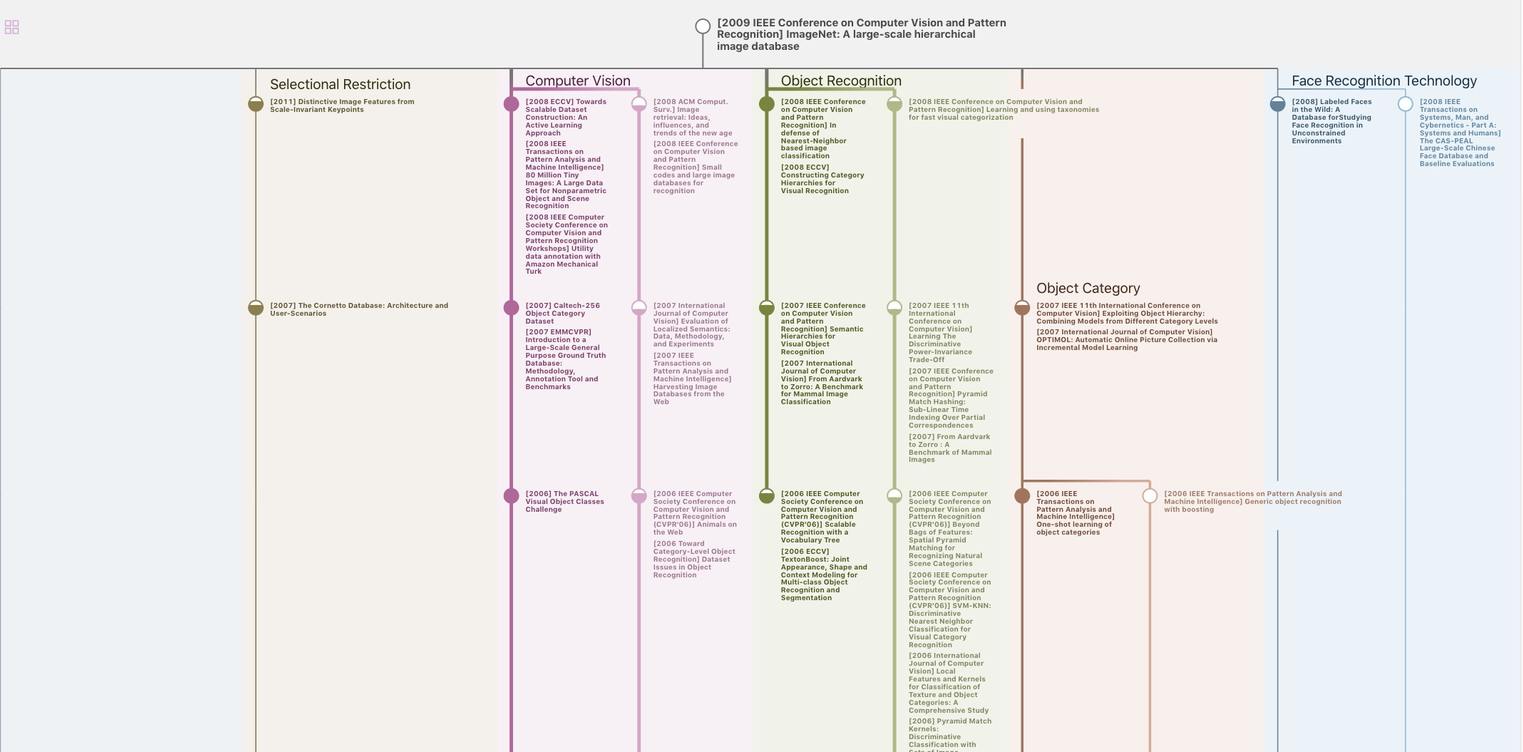
生成溯源树,研究论文发展脉络
Chat Paper
正在生成论文摘要