Two Tiered Distributed Training Algorithm for Acoustic Modeling
INTERSPEECH(2019)
摘要
We present a hybrid approach for scaling distributed training of neural networks by combining Gradient Threshold Compression (GTC) algorithm - a variant of stochastic gradient descent (SGD) - which compresses gradients with thresholding and quantization techniques and Blockwise Model Update Filtering (BMUF) algorithm - a variant of model averaging (MA). In this proposed method, we divide total number of workers into smaller subgroups in a hierarchical manner and limit frequent communication across subgroups. We update local model using GTC within a subgroup and global model using BMUF across different subgroups. We evaluate this approach in an Automatic Speech Recognition (ASR) task, by training deep long short-term memory (LSTM) acoustic models on 2000 hours of speech. Experiments show that, for a wide range in the number of GPUs used for distributed training, the proposed approach achieves a better trade-off between accuracy and scalability compared to GTC and BMUF.
更多查看译文
关键词
Speech Recognition, Distributed Stochastic Gradient Descent, Gradient Threshold Compression, BMUF
AI 理解论文
溯源树
样例
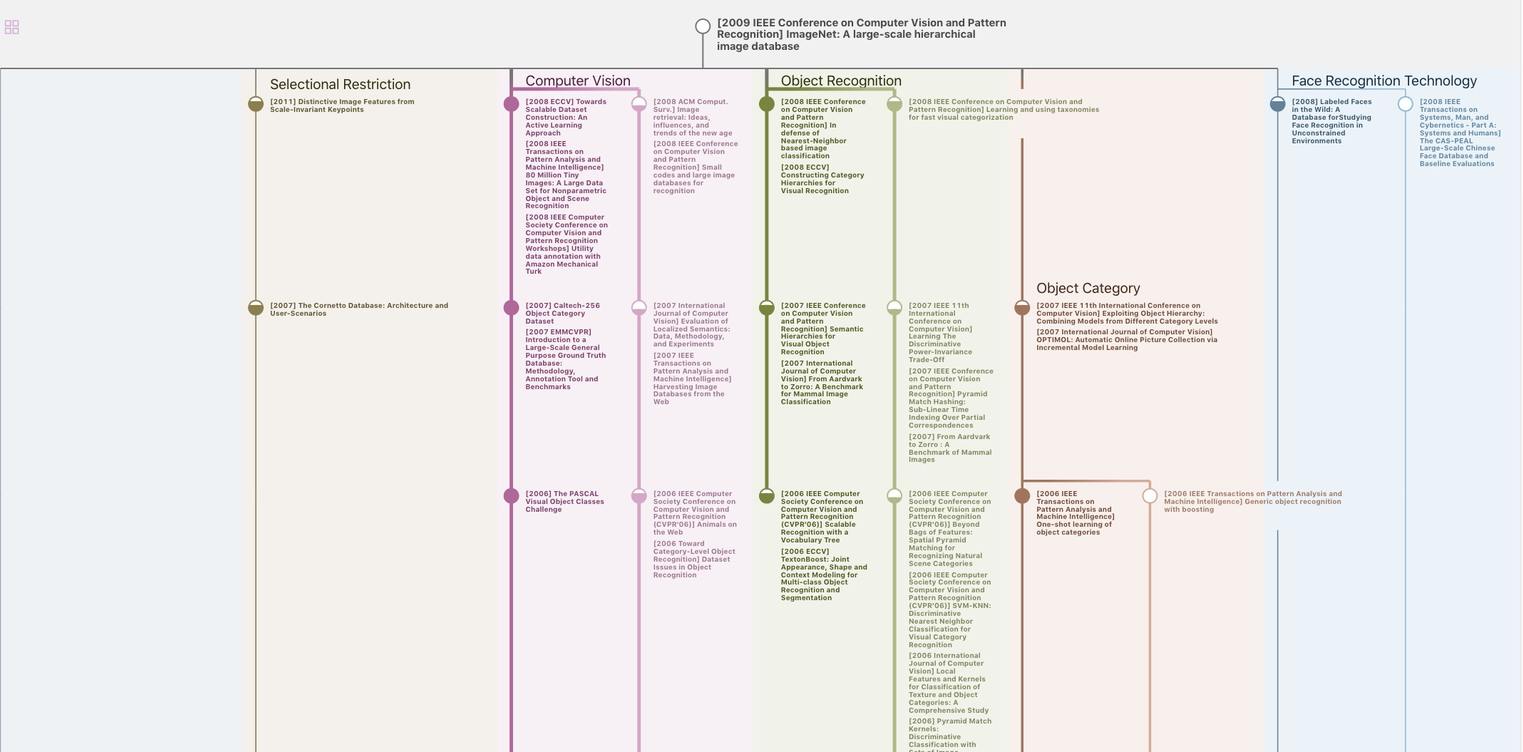
生成溯源树,研究论文发展脉络
Chat Paper
正在生成论文摘要