Temporally-Aware Acoustic Unit Discovery for Zerospeech 2019 Challenge
INTERSPEECH(2019)
摘要
Zero-resource speech processing efforts focus on unsupervised discovery of sub-word acoustic units. Common approaches work with spatial similarities between the acoustic frame representations within Bayesian or neural network-based frameworks. We propose two methods that utilize the temporal proximity information in addition to the acoustic similarity for clustering frames into acoustic units. The first approach uses a temporally biased self-organizing map (SOM) to discover such units. Since the SOM unit indices are correlated with (vector) spatial distance, we pool neighboring units and then train a recurrent neural network to predict each pooled unit. The second approach incorporates temporal awareness by training a recurrent sparse autoencoder, in which unsupervised clustering is done on the intermediate softmax layer. This network is then fine-tuned using aligned pairs of acoustically similar sequences obtained via unsupervised term discovery. Our approaches outperform the provided baseline system on two main metrics of the Zerospeech 2019 challenge, ABX-discriminability and bitrate of the quantized embeddings, both for English and the surprise language. Furthermore, the temporal-awareness and the post-filtering techniques adopted in this work resulted in an enhanced continuity of the decoding, yielding low bitrates.
更多查看译文
关键词
self-organizing map, gated recurrent unit, sparse recurrent autoencoder, correspondence autoencoder
AI 理解论文
溯源树
样例
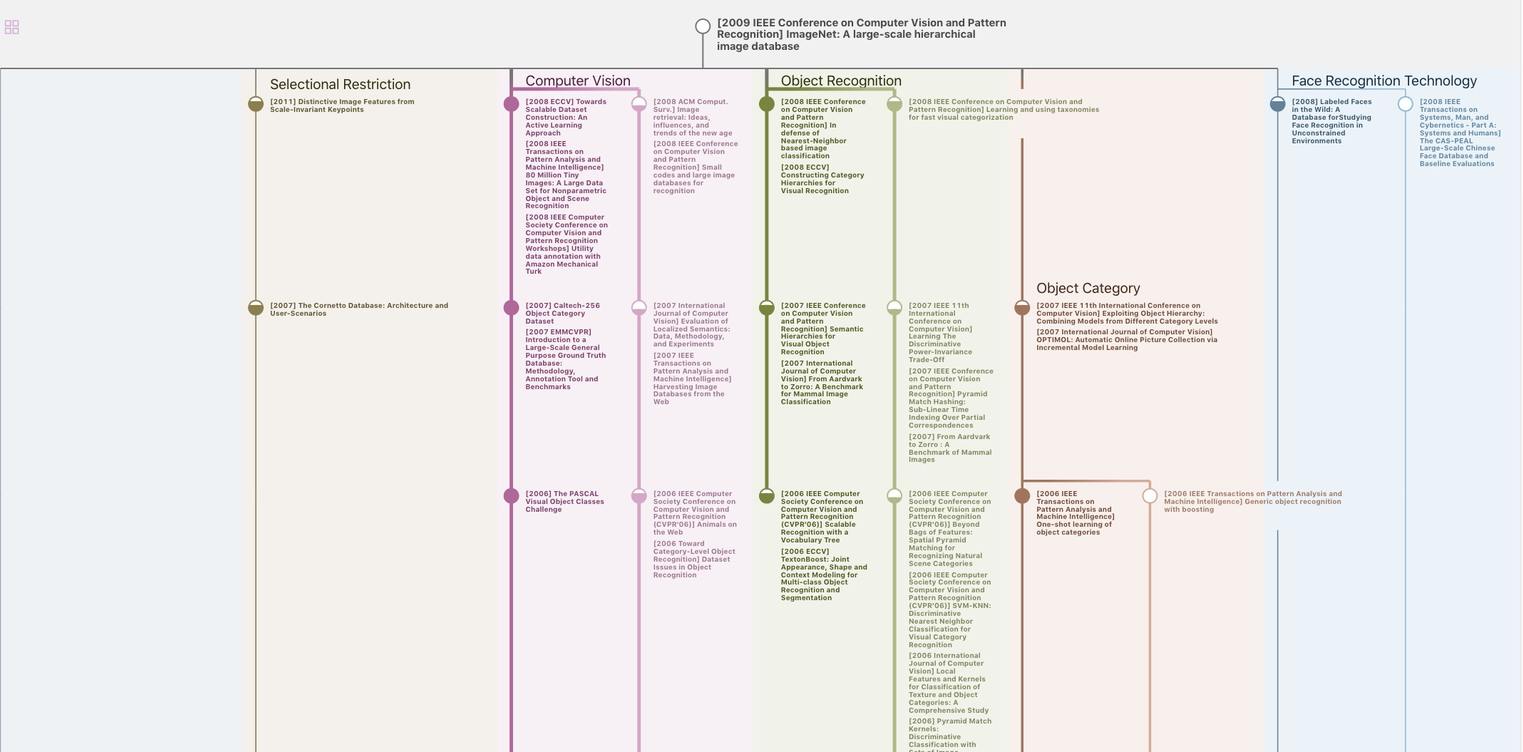
生成溯源树,研究论文发展脉络
Chat Paper
正在生成论文摘要