State-of-the-Art Speaker Recognition for Telephone and Video Speech: The JHU-MIT Submission for NIST SRE18
INTERSPEECH(2019)
摘要
We present a condensed description of the joint effort of JHUCLSP, JHU-HLTCOE, MIT-LL., MIT CSAIL and LSE-EPITA for NIST SRE18. All the developed systems consisted of x-vector/i-vector embeddings with some flavor of PLDA back-end. Very deep x-vector architectures-Extended and Factorized TDNN, and ResNets- clearly outperformed shallower x-vectors and i-vectors. The systems were tailored to the video (VAST) or to the telephone (CMN2) condition. The VAST data was challenging, yielding 4 times worse performance than other video based datasets like Speakers in the Wild. We were able to calibrate the VAST data with very few development trials by using careful adaptation and score normalization methods. The VAST primary fusion yielded EER=10.18% and Cprimary=0.431. By improving calibration in post-eval, we reached Cprimary=0.369. In CMN2, we used unsupervised SPLDA adaptation based on agglomerative clustering and score normalization to correct the domain shift between English and Tunisian Arabic models. The CMN2 primary fusion yielded EER=4.5% and Cprimary=0.313. Extended TDNN x-vector was the best single system obtaining EER=11.1% and Cprimary=0.452 in VAST; and 4.95% and 0.354 in CMN2.
更多查看译文
关键词
video speech,telephone,recognition,state-of-the-art,jhu-mit
AI 理解论文
溯源树
样例
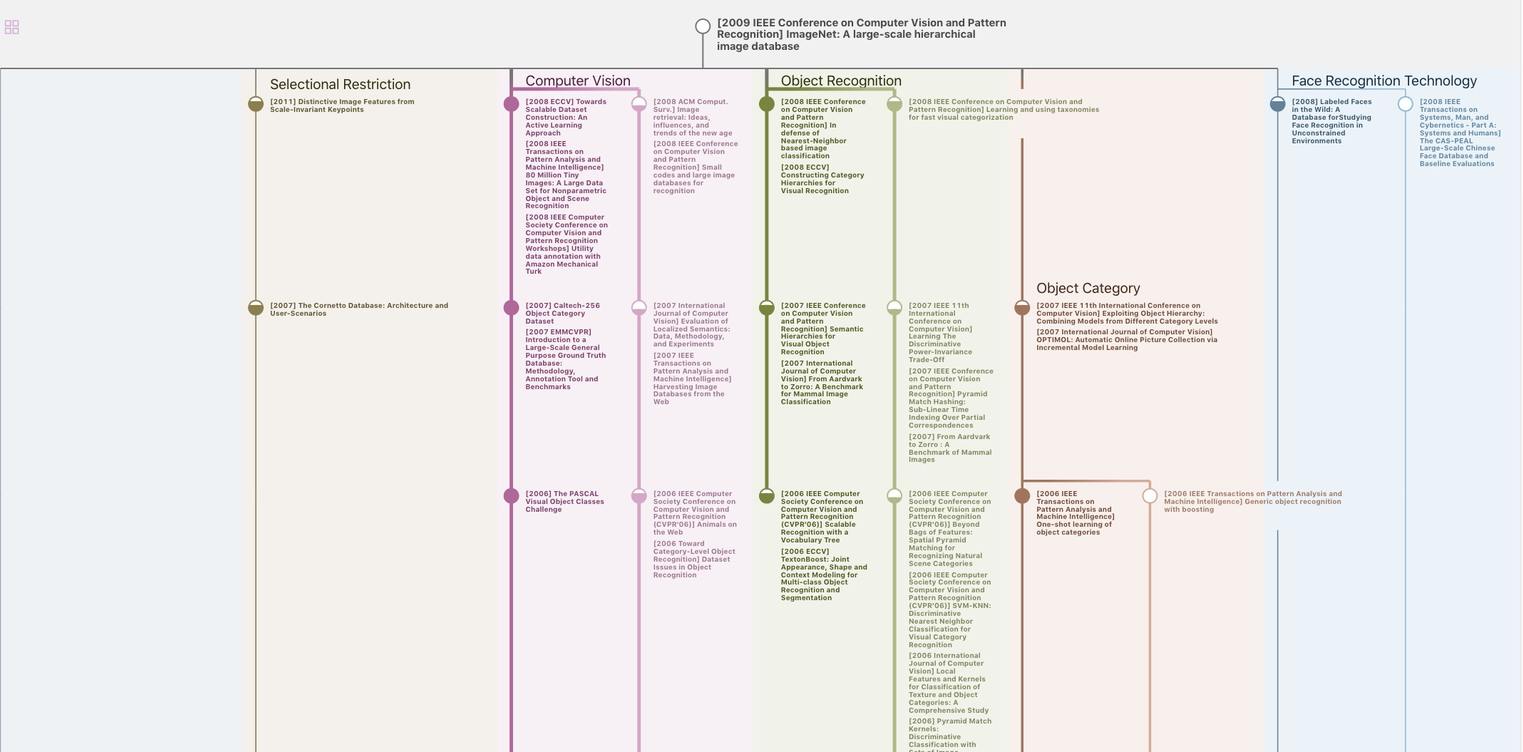
生成溯源树,研究论文发展脉络
Chat Paper
正在生成论文摘要