Mitigating Gender and L1 Differences to Improve State and Trait Recognition
INTERSPEECH(2019)
摘要
Automatic detection of speaker states and traits is made more difficult by intergroup differences in how they are distributed and expressed in speech and language. In this study, we explore various deep learning architectures for incorporating demographic information into the classification task. We find that early and late fusion of demographic information both improve performance on the task of personality recognition, and a multitask learning model, which performs best, also significantly improves deception detection accuracy. Our findings establish a new state-of-the-art for personality recognition and deception detection on the CXD corpus, and suggest new best practices for mitigating intergroup differences to improve speaker state and trait recognition.
更多查看译文
关键词
Personality, Deception, L1, Gender, Multi-Task Learning
AI 理解论文
溯源树
样例
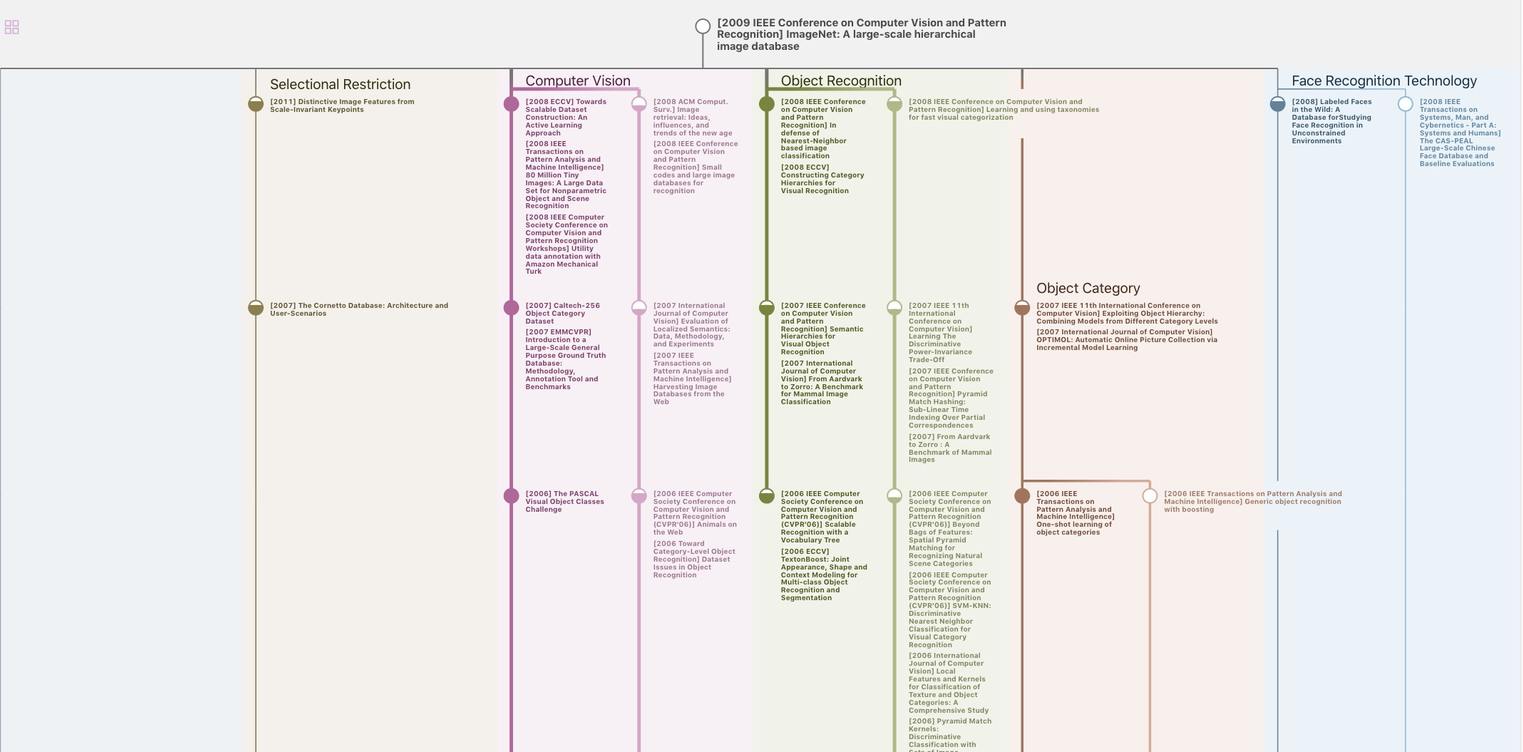
生成溯源树,研究论文发展脉络
Chat Paper
正在生成论文摘要