Diagnosing Dysarthria with Long Short-Term Memory Networks
INTERSPEECH(2019)
Abstract
This paper proposes the use of Recurrent Neural Networks (RNNs) with Long Short-Term Memory (LSTM) units for determining whether Mandarin-speaking individuals are afflicted with a form of Dysarthria based on samples of syllable pronunciations. Several LSTM network architectures are evaluated on this binary classification task, using accuracy and Receiver Operating Characteristic (ROC) curves as metrics. The LSTM models are shown to significantly improve upon a baseline fully connected network, reaching over 90% area under the ROC curve on the task of classifying new speakers, when a sufficient number of cepstrum coefficients are used. The results show that the LSTM's ability to leverage temporal information within its input makes for an effective step in the pursuit of accessible Dysarthria diagnoses.
MoreTranslated text
Key words
Dysarthria, RNN, LSTM, speech processing
AI Read Science
Must-Reading Tree
Example
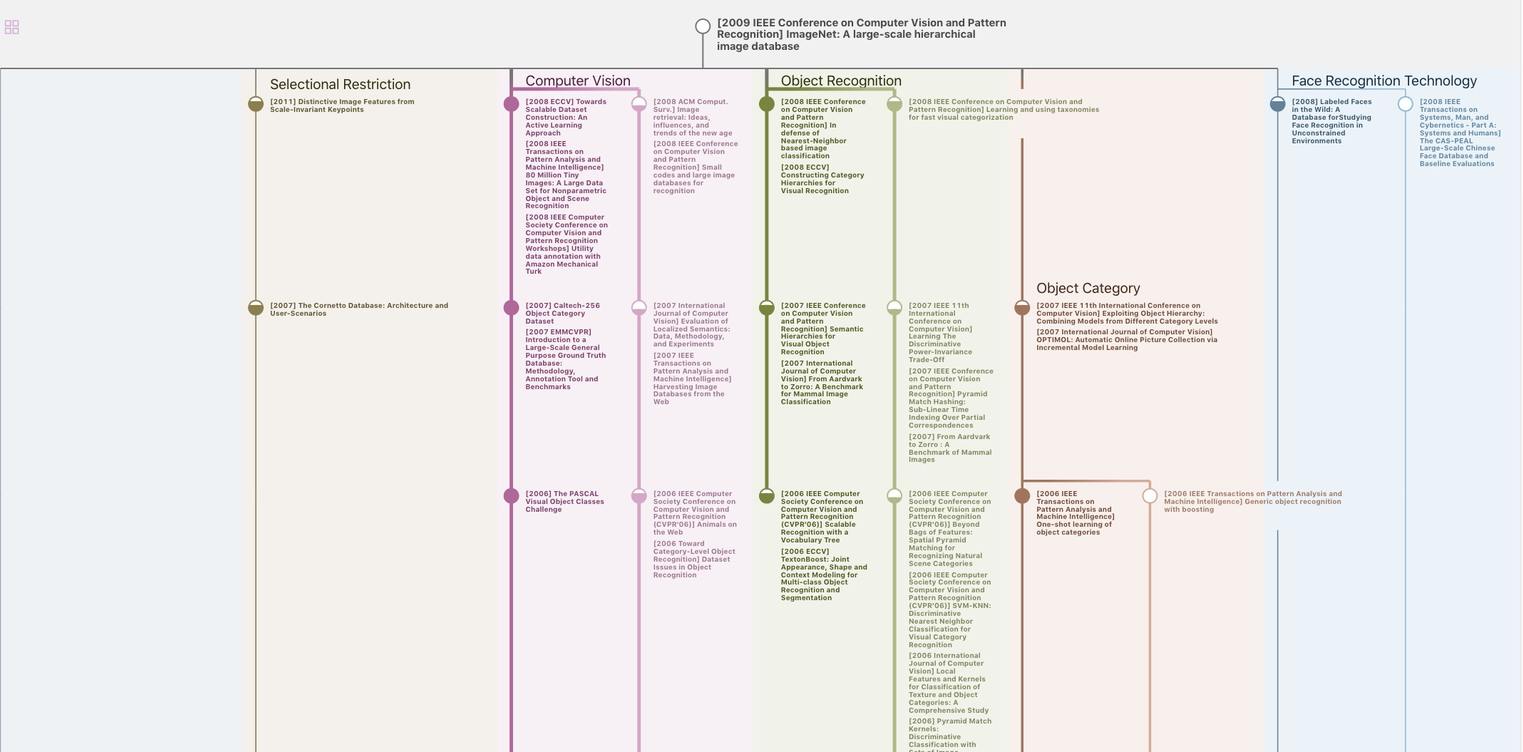
Generate MRT to find the research sequence of this paper
Chat Paper
Summary is being generated by the instructions you defined