Fast And Accurate Binary Response Mixed Model Analysis Via Expectation Propagation
JOURNAL OF THE AMERICAN STATISTICAL ASSOCIATION(2020)
摘要
Expectation propagation is a general prescription for approximation of integrals in statistical inference problems. Its literature is mainly concerned with Bayesian inference scenarios. However, expectation propagation can also be used to approximate integrals arising in frequentist statistical inference. We focus on likelihood-based inference for binary response mixed models and show that fast and accurate quadrature-free inference can be realized for the probit link case with multivariate random effects and higher levels of nesting. The approach is supported by asymptotic calculations in which expectation propagation is seen to provide consistent estimation of the exact likelihood surface. Numerical studies reveal the availability of fast, highly accurate and scalable methodology for binary mixed model analysis.
更多查看译文
关键词
Best prediction, Generalized linear mixed models, Maximum likelihood, Kullback-Leibler projection, Message passing, Quasi-Newton methods, Scalable statistical methodology
AI 理解论文
溯源树
样例
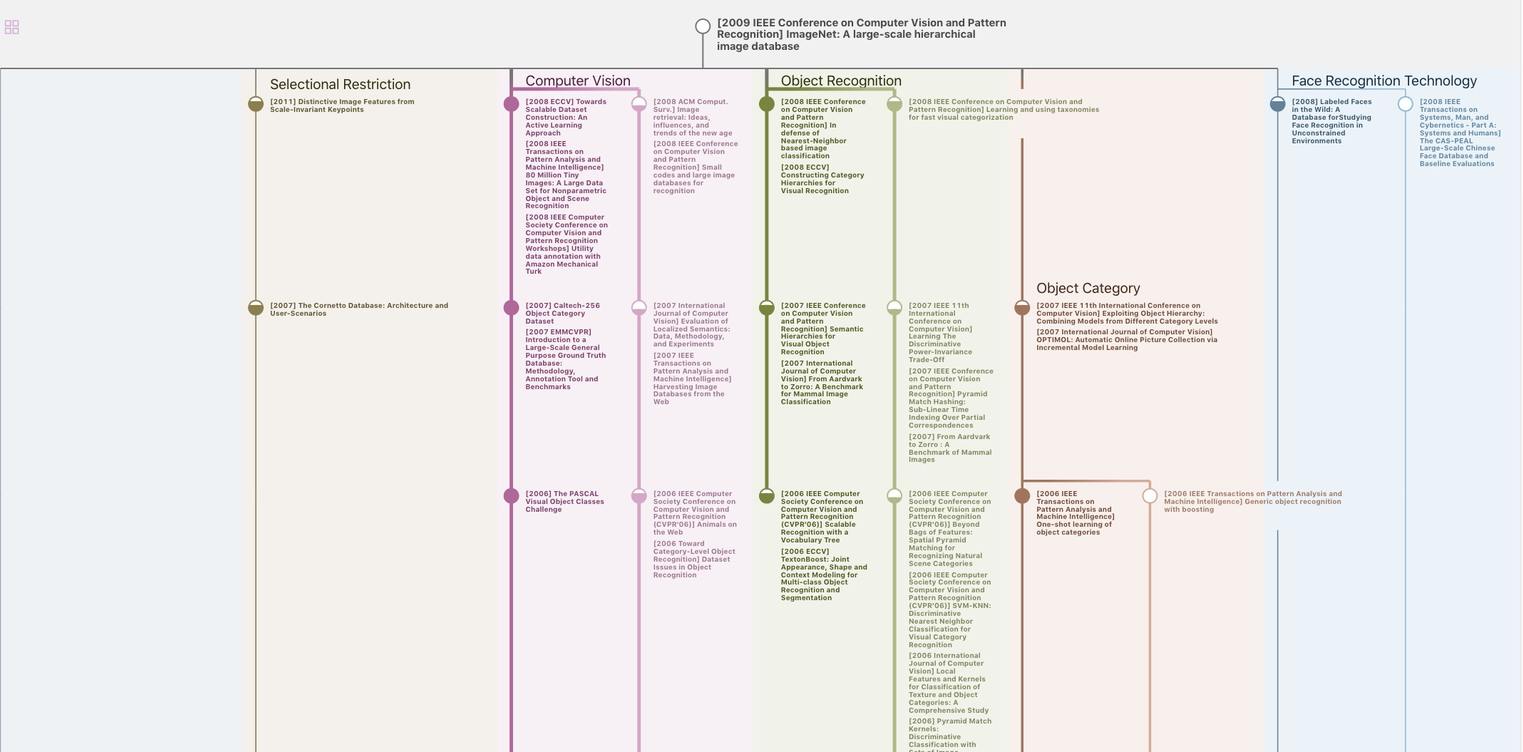
生成溯源树,研究论文发展脉络
Chat Paper
正在生成论文摘要