Detecting Road Surface Wetness Using Microphones And Convolutional Neural Networks
146TH AES CONVENTION(2019)
摘要
The automatic detection of road conditions in next-generation vehicles is an important task that is getting increasing interest from the research community. Its main applications concern driver safety, autonomous vehicles and in-car audio equalization. These applications rely on sensors that must be deployed following a trade-off between installation and maintainance costs and effectiveness. In this article we tackle road surface wetness classification using microphones and comparing convolutional neural networks (CNN) with bi-directional long-short term memory networks (BLSTM), following previous motivating works. We introduce a new dataset to assess the role of different tire types and discuss the deployment of the microphones. We find a solution that is immune to water and sufficiently robust to in-cabin interference and tire type changes. Classification results with the recorded dataset reach a 95% F-score and a 97% F-score using the CNN and BLSTM methods, respectively.
更多查看译文
AI 理解论文
溯源树
样例
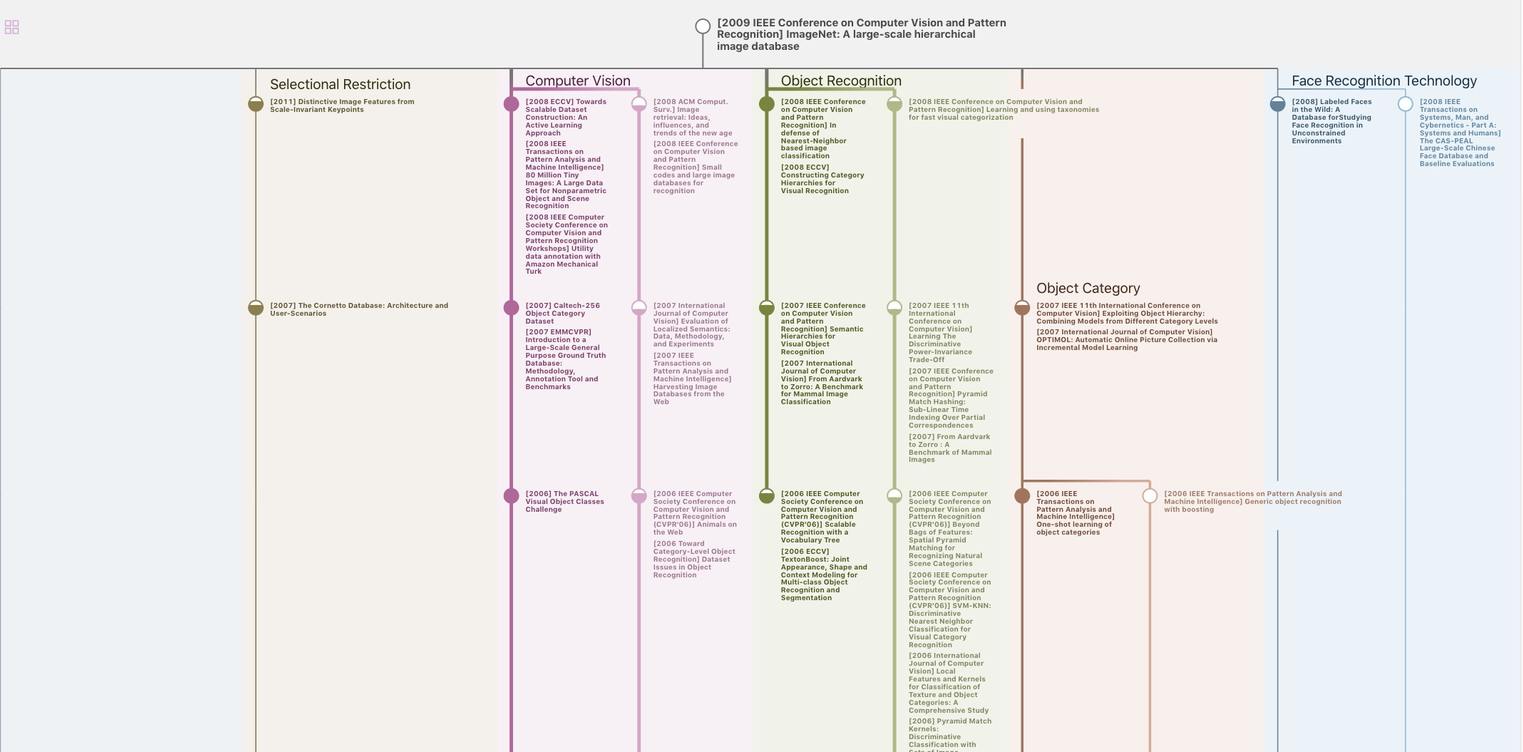
生成溯源树,研究论文发展脉络
Chat Paper
正在生成论文摘要