Hypergcn: A New Method Of Training Graph Convolutional Networks On Hypergraphs
ADVANCES IN NEURAL INFORMATION PROCESSING SYSTEMS 32 (NIPS 2019)(2019)
摘要
In many real-world networks such as co-authorship, co-citation, etc., relationships are complex and go beyond pairwise associations. Hypergraphs provide a flexible and natural modeling tool to model such complex relationships. The obvious existence of such complex relationships in many real-world networks naturally motivates the problem of learning with hypergraphs. A popular learning paradigm is hypergraph-based semi-supervised learning (SSL) where the goal is to assign labels to initially unlabelled vertices in a hypergraph. Motivated by the fact that a graph convolutional network (GCN) has been effective for graph-based SSL, we propose HyperGCN, a novel way of training a GCN for SSL on hypergraphs based on tools from sepctral theory of hypergraphs. We demonstrate HyperGCN's effectiveness through detailed experimentation on real-world hypergraphs for SSL and combinatorial optimisation and analyse when it is going to be more effective than state-of-the art baselines. We have made the source code available.
更多查看译文
关键词
supervised learning,semi-supervised learning
AI 理解论文
溯源树
样例
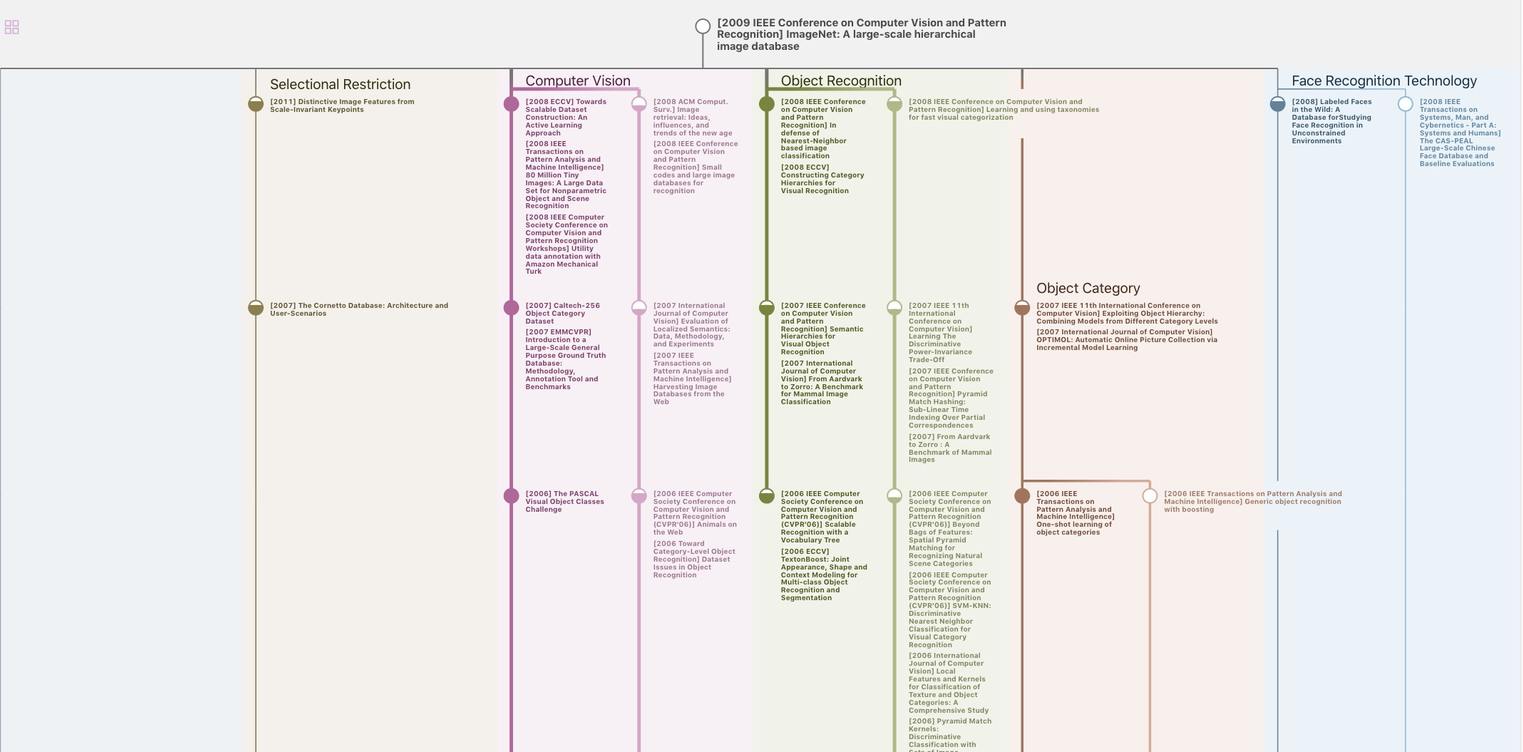
生成溯源树,研究论文发展脉络
Chat Paper
正在生成论文摘要