Managing Uncertainty in Data-Driven Simulation-Based Optimization
Computers & Chemical Engineering(2020)
摘要
Optimization using data from complex simulations has become an attractive decision-making option, due to ability to embed high-fidelity, non-linear understanding of processes within the search for optimal values. Due to lack of tractable algebraic equations, the link between simulations and optimization is oftentimes a surrogate metamodel. However, several forms of uncertainty exist within the cycle that links simulation data, to metamodels, to optimization. Uncertainty may originate from parameters of the simulation, or the form and fitted parameters of the metamodel. This paper reviews different literatures that are relevant to surrogate-based optimization and proposes different strategies for handling uncertainty, by combining machine learning with stochastic programming, robust optimization, and discrepancy modeling. We show that incorporating uncertainty management within simulation-based optimization leads to more robust solutions, which protect the decision-maker from infeasible solutions. We present the results of our proposed approaches through a case study for direct-air capture through temperature swing adsorption.
更多查看译文
关键词
Surrogate modeling,Simulation optimization,Direct air capture,Neural networks,Polynomial interpolation
AI 理解论文
溯源树
样例
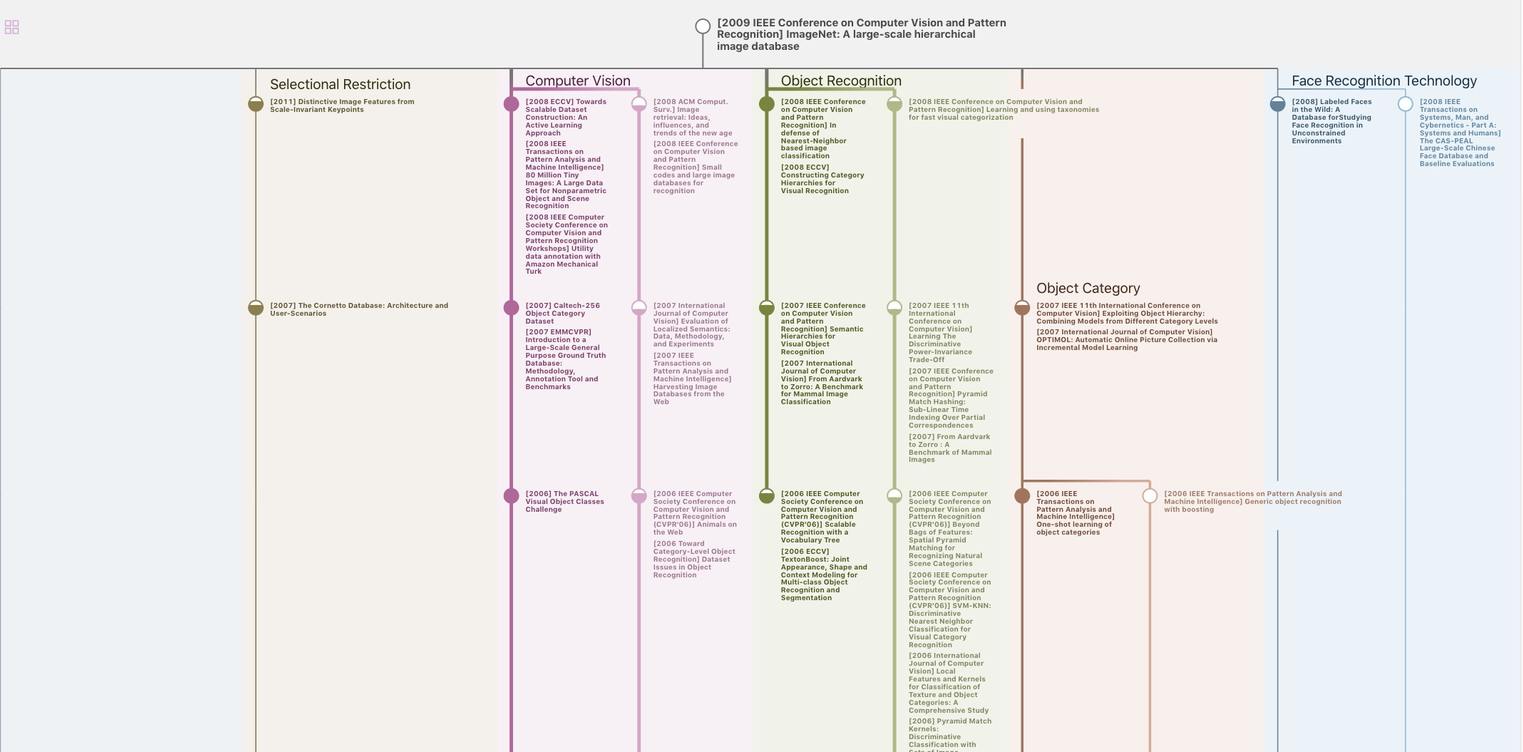
生成溯源树,研究论文发展脉络
Chat Paper
正在生成论文摘要