Prediction of hydrogen adsorption in nanoporous materials from the energy distribution of adsorption sites
MOLECULAR PHYSICS(2019)
摘要
We present a fast and accurate, semi-analytical method for predicting hydrogen adsorption in nanoporous materials. For any temperature and pressure, the adsorbed amount is calculated as an integral over the energy density of adsorption sites (guest-host interactions) plus an average guest-guest term. The guest-host interaction energy is calculated using a classical force field with hydrogen modelled as a single-site probe. The guest-guest interaction energy is approximated using an average coordination number, which is regressed using Gaussian Process Regression (GPR). Local adsorption at each site is then modelled using a Langmuir isotherm, which when weighted with its probability density gives an accurate description of hydrogen adsorption. The method is tested on 933 metal-organic frameworks (MOFs) from the Computation-Ready Experimental (CoRE) MOF database at 77 K from to 100 bar, and the results are compared against GCMC predictions. To demonstrate the utility of the method, we calculated hydrogen adsorption isotherms for 12,914 existing MOF structures, at two different temperatures at a speed about 100 times that of GCMC simulations and analyzed the results. We found 13 MOFs with predicted deliverable capacities exceeding the DOE target of 50 g/L for adsorption at 100 bar, 77 K and desorption at 5 bar, 160 K.
更多查看译文
关键词
Hydrogen storage,high-throughput screening,metal-organic frameworks,MOFs,machine learning,Gaussian process regression
AI 理解论文
溯源树
样例
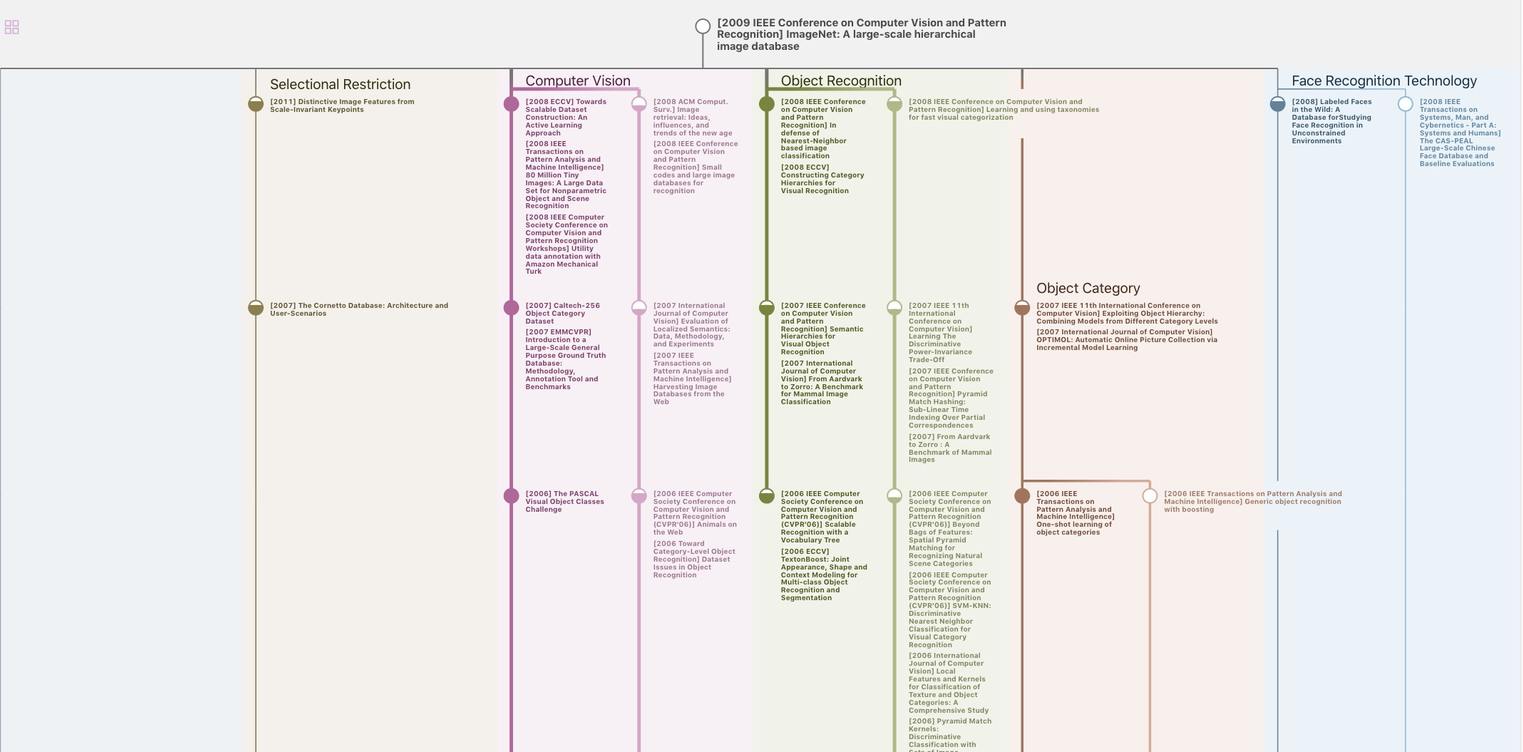
生成溯源树,研究论文发展脉络
Chat Paper
正在生成论文摘要