Multi-Label Zero-Shot Learning with Transfer-Aware Label Embedding Projection
2019 IEEE INTERNATIONAL CONFERENCE ON IMAGE PROCESSING (ICIP)(2018)
Abstract
Zero-shot learning transfers knowledge from seen classes to novel unseen classes to reduce human labor of labelling data for building new classifiers. Much effort on zero-shot learning however has focused on the standard multi-class setting, the more challenging multi-label zero-shot problem has received limited attention. In this paper we propose a transfer-aware embedding projection approach to tackle multi-label zero-shot learning. The approach projects the label embedding vectors into a low-dimensional space to induce better inter-label relationships and explicitly facilitate information transfer from seen labels to unseen labels, while simultaneously learning a max-margin multi-label classifier with the projected label embeddings. Auxiliary information can be conveniently incorporated to guide the label embedding projection to further improve label relation structures for zero-shot knowledge transfer. We conduct experiments for zero-shot multi-label image classification. The results demonstrate the efficacy of the proposed approach.
MoreTranslated text
Key words
Zero-shot learning, multi-label classification, label embedding, auxiliary information
AI Read Science
Must-Reading Tree
Example
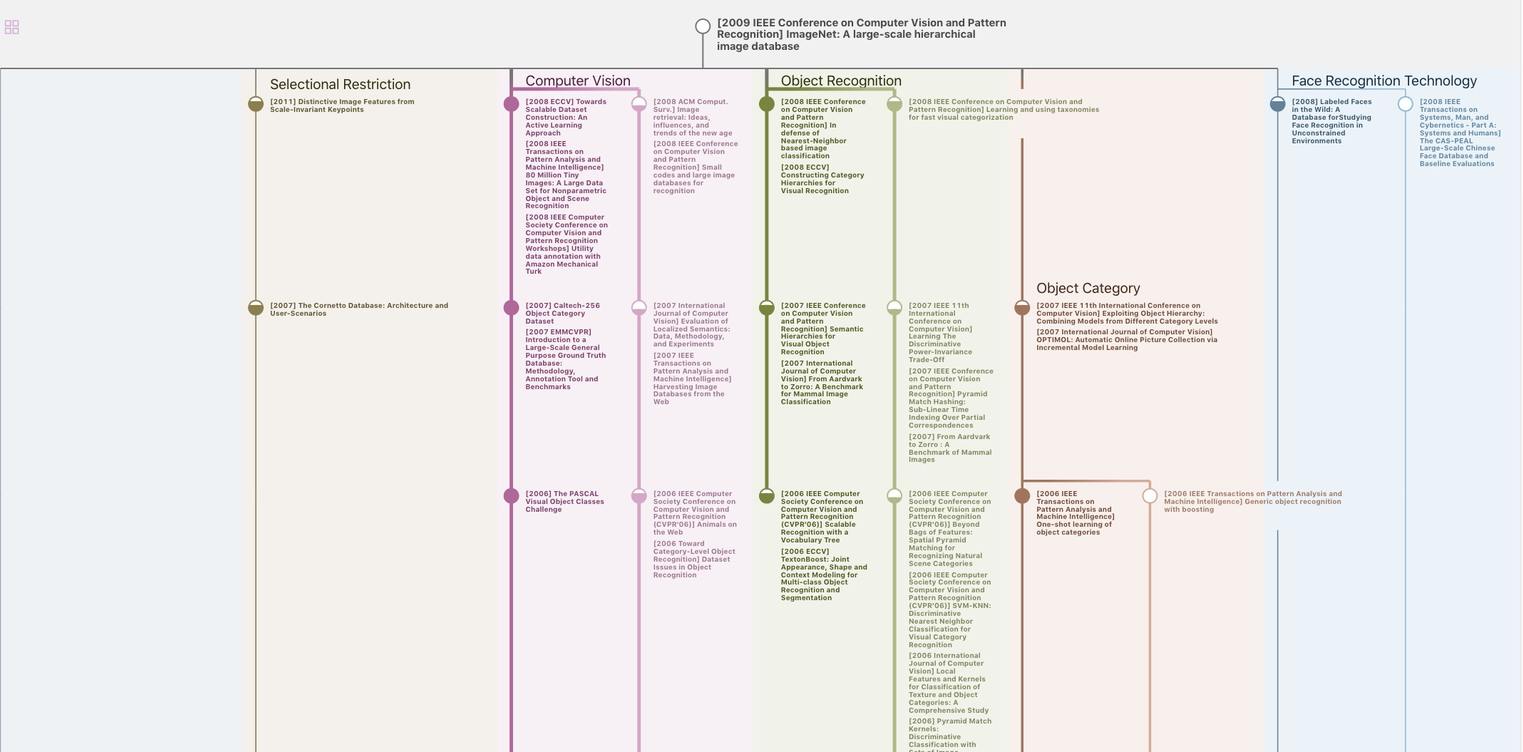
Generate MRT to find the research sequence of this paper
Chat Paper
Summary is being generated by the instructions you defined