An Analysis of Differential Privacy Research in Location Data
2019 IEEE 5th Intl Conference on Big Data Security on Cloud (BigDataSecurity), IEEE Intl Conference on High Performance and Smart Computing, (HPSC) and IEEE Intl Conference on Intelligent Data and Security (IDS)(2019)
摘要
Location data is becoming ubiquitous with the spread of smart devices, and social media geo-tagged feeds. However, sharing location data may lead to serious privacy risks that must not be overlooked. Differential privacy is the standard technique that provides strong privacy guarantees regardless of the adversary's side information. Usually, this is achieved by adding noise to the true result of the statistical query extracted from the data. However, a straight forward application of differential privacy to location data is not always possible. The growing interest in designing solutions to achieve differential privacy that take into account the characteristics of location data is evident from the substantial number of works done in this field. This paper briefly reviews research works done in differential privacy targeted toward location data from the data flow perspective, including the collection, aggregation, and mining. Our goal is to help newcomers to the field to better understand the state-of-the art by providing a research map that highlights the different challenges in designing frameworks, as well as novel approaches, that tackle the characteristics of location data. We identify multiple challenges to the application of differential privacy to location data, such as the calibration of the added noise to assure utility, finding the optimal spatial division to release the location aggregate per region while balancing privacy and utility. We also discuss the future directions concluded from the analysis.
更多查看译文
关键词
differential privacy,location data
AI 理解论文
溯源树
样例
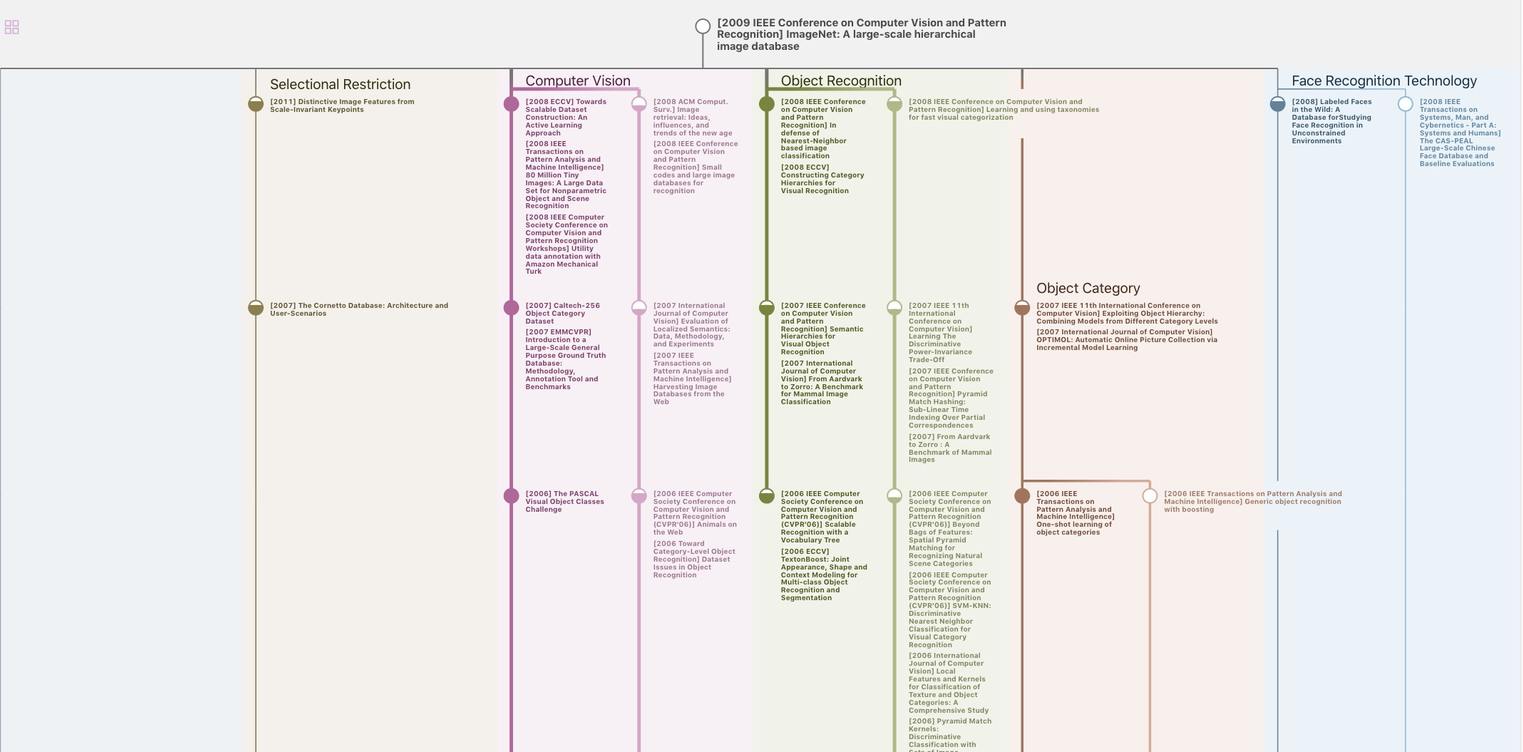
生成溯源树,研究论文发展脉络
Chat Paper
正在生成论文摘要