Marginalized Off-Policy Evaluation for Reinforcement Learning
neural information processing systems(2019)
摘要
Off-policy evaluation is concerned with evaluating the performance of a policy using the historical data obtained by different behavior policies. In the real-world application of reinforcement learning, acting a policy can be costly and dangerous, and off-policy evaluation usually plays as a crucial step. Currently, the existing methods for off-policy evaluation are mainly based on the Markov decision process (MDP) model of discrete tree MDPs, and they suffer from high variance due to the cumulative product of importance weights. In this paper, we propose a new off-policy evaluation approach directly based on the discrete directed acyclic graph (DAG) MDPs. Our approach can be applied to most of the estimators of off-policy evaluation without modification and could reduce the variance dramatically. We also provide a theoretical analysis of our approach and evaluate it by empirical results.
更多查看译文
AI 理解论文
溯源树
样例
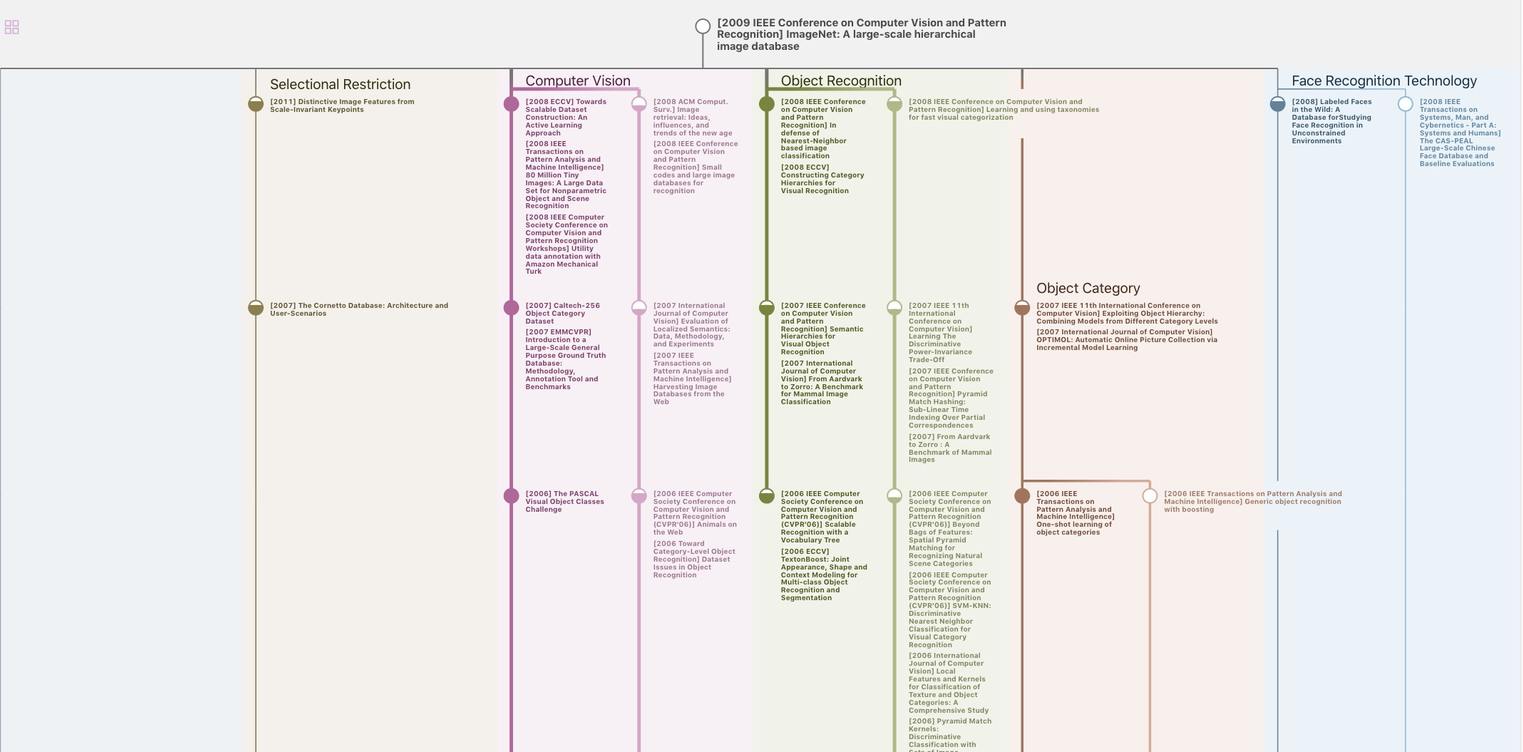
生成溯源树,研究论文发展脉络
Chat Paper
正在生成论文摘要