Heteroscedastic and heavy-tailed regression with mixtures of skew Laplace normal distributions
JOURNAL OF STATISTICAL COMPUTATION AND SIMULATION(2019)
Abstract
Joint modelling skewness and heterogeneity is challenging in data analysis, particularly in regression analysis which allows a random probability distribution to change flexibly with covariates. This paper, based on a skew Laplace normal (SLN) mixture of location, scale, and skewness, introduces a new regression model which provides a flexible modelling of location, scale and skewness parameters simultaneously. The maximum likelihood (ML) estimators of all parameters of the proposed model via the expectation-maximization (EM) algorithm as well as their asymptotic properties are derived. Numerical analyses via a simulation study and a real data example are used to illustrate the performance of the proposed model.
MoreTranslated text
Key words
EM algorithm,joint location,scale and skewness models,mixture model,ML estimation,SLN,SN
AI Read Science
Must-Reading Tree
Example
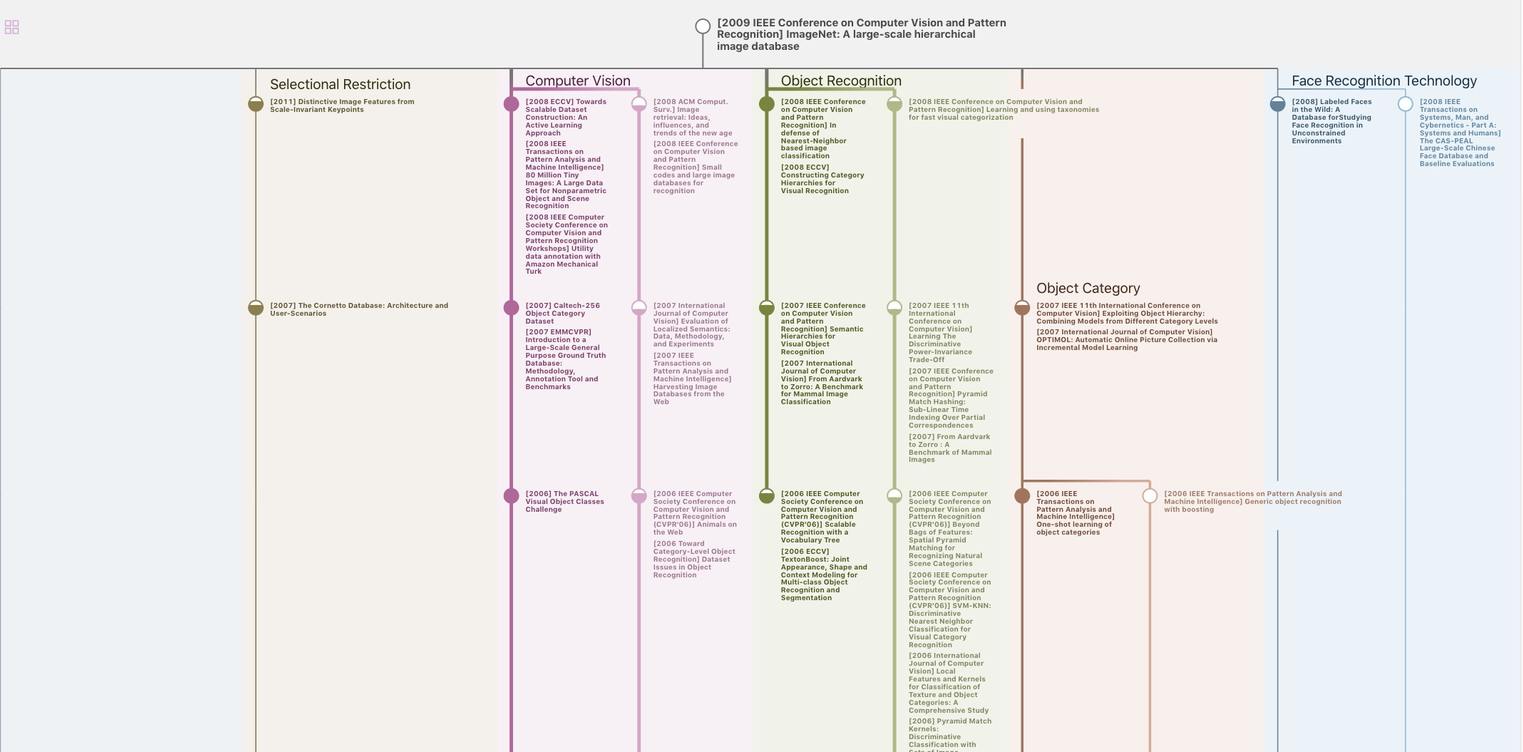
Generate MRT to find the research sequence of this paper
Chat Paper
Summary is being generated by the instructions you defined