Learning Pushing Skills Using Object Detection and Deep Reinforcement Learning
2019 IEEE International Conference on Mechatronics and Automation (ICMA)(2019)
摘要
Pushing objects to the target place on a tabletop surface is a task which requires skillful interaction with the physical world. Usually, this is achieved by precisely modeling physical properties of the objects, robot, and the environment for explicit planning. In contrast, as explicitly modeling the physical environment is not always feasible and involves various uncertainties, we learn robotic pushing skills with deep reinforcement learning and based on only visual feedback. For this, we model the task with rewards and use deep deterministic policy gradient (DDPG) algorithms to update the control strategy. And we combine You Only Look Once (YOLO) which is one of state-of-the-art object detection algorithm with DDPG algorithm, the method reduces the exploring space of the robot, produces more effective learning samples, and improves the learning efficiency greatly. In simulation experiments, the robot learned pushing skills after training 400 episodes, the success rate is around 95% and the learning efficiency is much higher than using DDPG alone. In real-world experiments, the robot learned pushing skills after training 800 episodes and the success rate is around 85%.
更多查看译文
关键词
DDPG,YoloV3,UR5
AI 理解论文
溯源树
样例
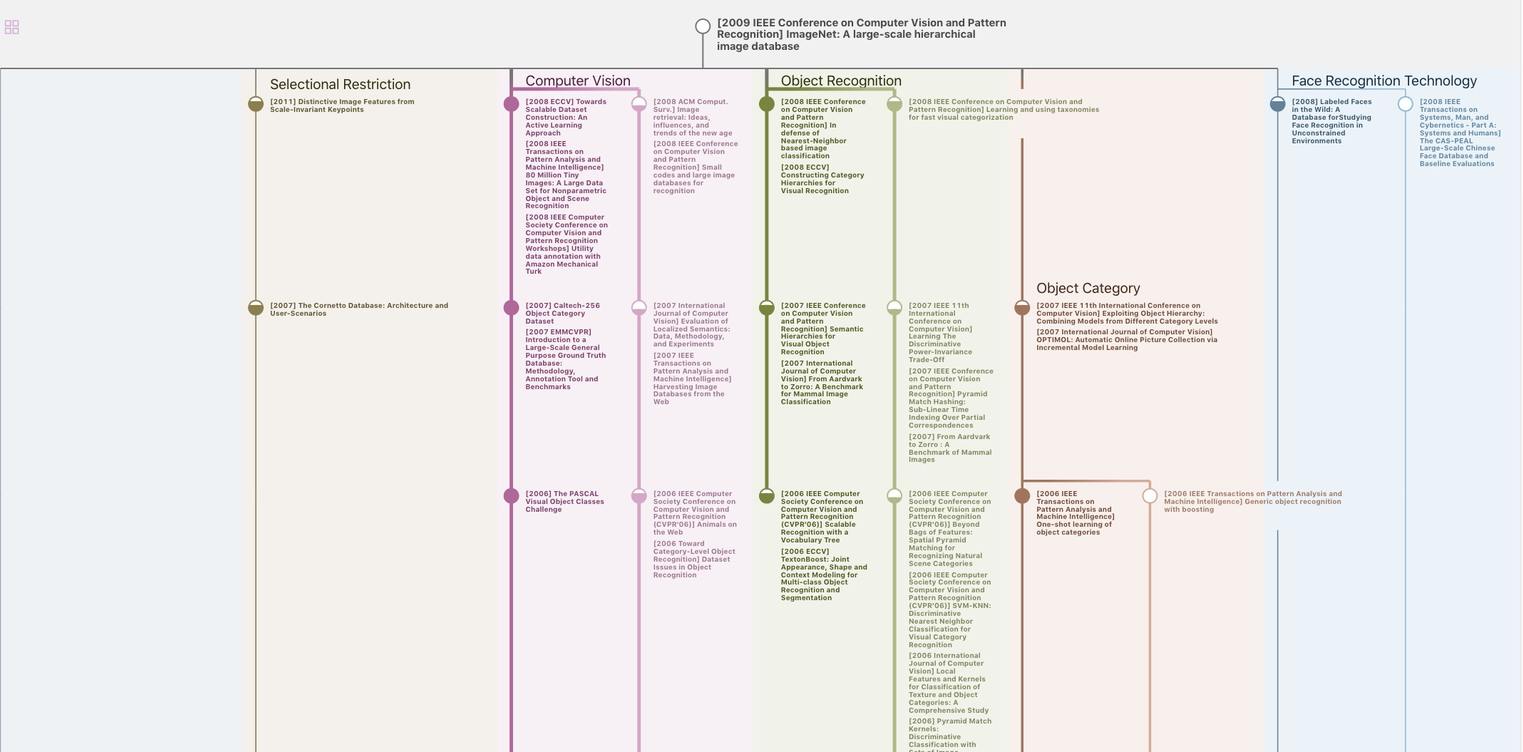
生成溯源树,研究论文发展脉络
Chat Paper
正在生成论文摘要