Digital Ampelographer: A CNN Based Preliminary Approach
EPIA (1)(2019)
Abstract
Authenticity, traceability and certification are key to assure both quality and confidence to wine consumers and an added commercial value to farmers and winemakers. Grapevine variety stands out as one of the most relevant factors to be considered in wine identification within the whole wine sector value chain. Ampelography is the science responsible for grapevine varieties identification based on (i) in-situ visual inspection of grapevine mature leaves and (ii) on the ampelographer experience. Laboratorial analysis is a costly and time-consuming alternative. Both the lack of experienced professionals and context-induced error can severely hinder official regulatory authorities' role and therefore bring about a significant impact in the value chain. The purpose of this paper is to assess deep learning potential to classify grapevine varieties through the ampelometric analysis of leaves. Three convolutional neural networks architectures performance are evaluated using a dataset composed of six different grapevine varieties leaves. This preliminary approach identified Xception architecture as very promising to classify grapevine varieties and therefore support a future autonomous tool that assists the wine sector stakeholders, particularly the official regulatory authorities.
MoreTranslated text
Key words
CNN, Xception, ResNet, VGG, Precision viticulture, Ampelography, Grapevine identification, Wine Certification, Douro Demarcated Region
AI Read Science
Must-Reading Tree
Example
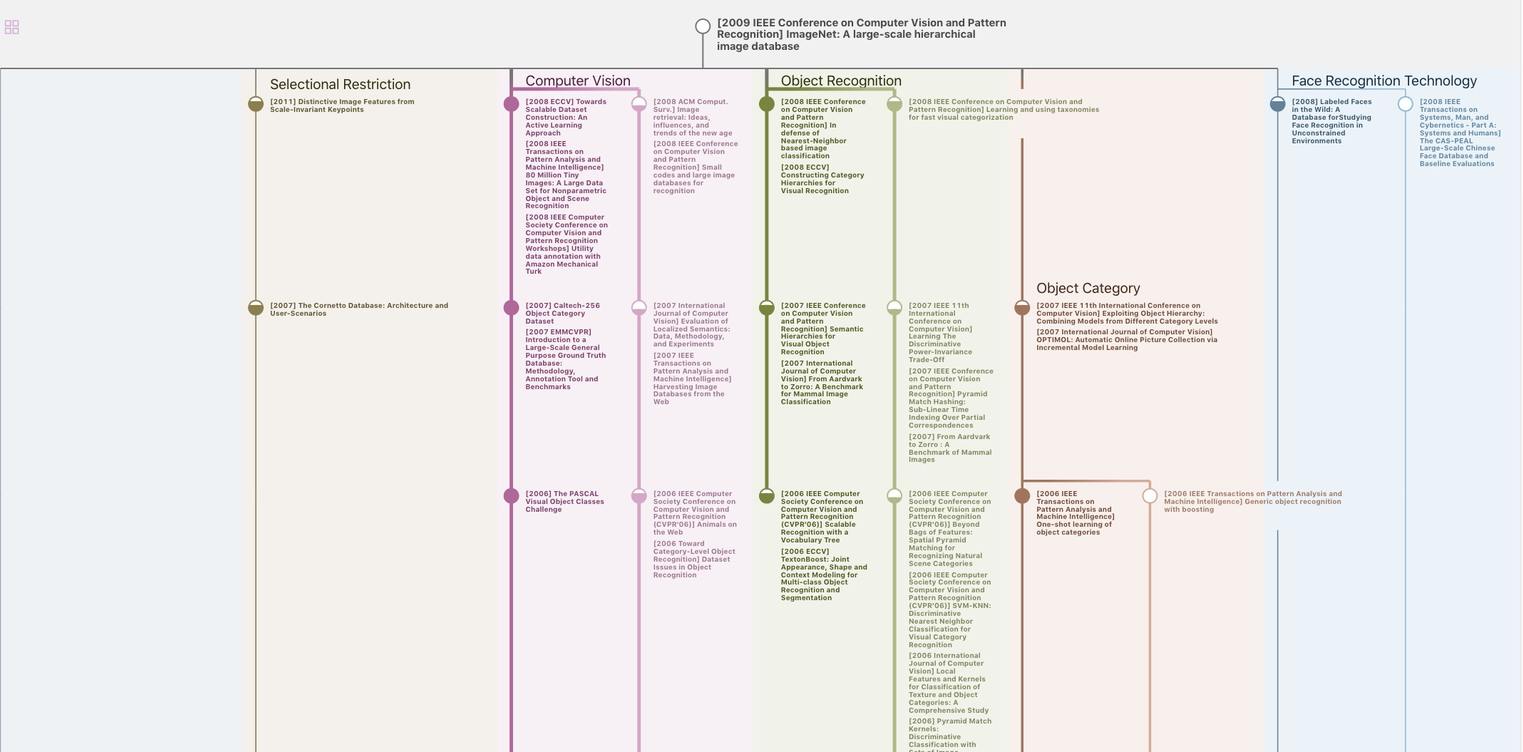
Generate MRT to find the research sequence of this paper
Chat Paper
Summary is being generated by the instructions you defined