Adaptive-Skip-TransE Model: Breaking Relation Ambiguities for Knowledge Graph Embedding
KSEM (1)(2019)
摘要
Knowledge graph embedding aims to encode entities and relations into a low-dimensional vector space, obtaining its distributed vector representation for further knowledge learning and reasoning. Most existing methods assume that each relation owns one unique vector. However, in the real world, many relations are multi-semantic. We note that a reasonable adaptive learning method for the number of semantics for a given relation is lacking in knowledge graph embedding. In this paper, we propose a probabilistic model Skip-TransE, which comprehensively considers the two-way prediction ability and global loss intensity of the golden triplets. Then based on Skip-TransE, its non-parametric Bayesian extended model Adaptive-Skip-TransE is presented to automatically learn the number of semantics for each relation. Extensive experiments show that the proposed models can achieve some substantial improvements above the state-of-the-art baselines.
更多查看译文
关键词
Knowledge embedding, Multi-semantic relations, Adaptive learning
AI 理解论文
溯源树
样例
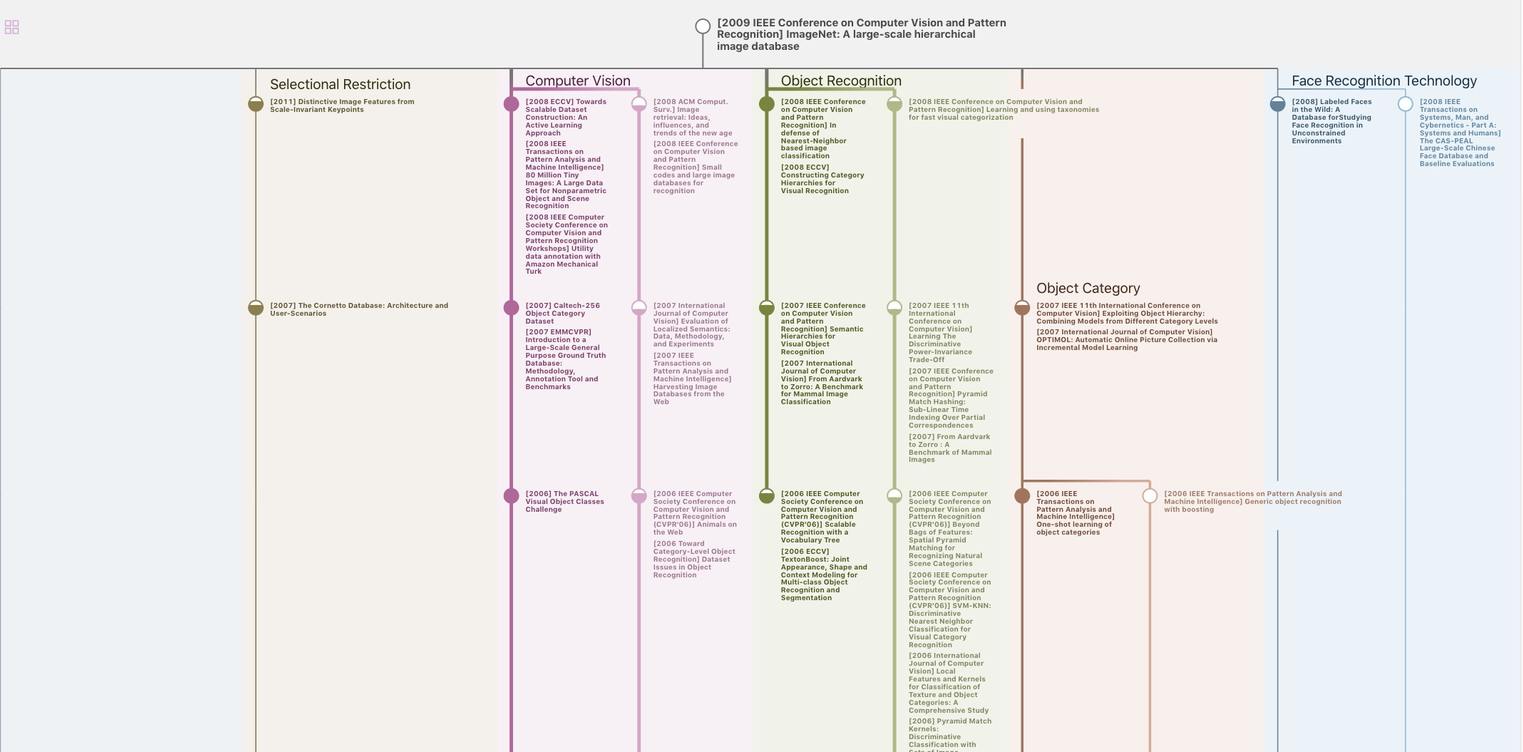
生成溯源树,研究论文发展脉络
Chat Paper
正在生成论文摘要