Prognostic Value of Admission Parameters in a Machine Learning Predictive Model in Patients With Severe Traumatic Brain Injury and Acute Subdural Hematomas
NEUROSURGERY(2019)
Abstract
INTRODUCTION: Acute subdural hematoma (aSDH) in the context of severe traumatic brain injury (TBI) is a neurosurgical emergency. Predictive models have been used in an attempt to modulate the morbidity and mortality of patient outcomes. We used machine learning (ML) to identify admission risk factors predictive of long-term morbidity in the severe TBI patient population with aSDH. METHODS: Between 2013 and 2016, 85 patients with severe TBI and aSDH were included in the analysis. Random forest, ML architecture, was used to create a predictive model of long-term morbidity stratification. About 46 patients were included in the high morbidity group [Glasgow Outcome Scale (GOS) 1-2] and 39 patients were in the low morbidity group (GOS 3-5). We included 30 admission input variables including medical and surgical co-morbidities, neurological examination, laboratory values, and radiographic findings. RESULTS: The predictive model showed a 78% precision. The highest scoring input variable was the pupillary examination in predicting high vs low morbidity (bilaterally unreactive vs symmetrically reactive; P < .0001). GCS on admission was higher in the low morbidity group (4 [3-7] vs 7 [3-7]; P < .0101). Rotterdam scores were higher in the high-morbidity group (3 [3-5] vs 4 [4-5]; P < .0032). GCS motor examination on admission was higher in the low-morbidity group (5 [1-5] vs. 2 [1-5]; P < .0106). The basal cisterns were found to be more patent in patients with the low-morbidity group (P = .0012). CONCLUSION: ML is an efficient tool that can provide a reasonable level of accuracy in predicting long-term morbidity in patients with severe TBI and aSDH. Monitoring these admission criteria can help with risk-stratification of patients into higher and low risk tracks. Integration of ML into the treatment algorithm may allow the development of more refined guidelines to guide goal-directed therapy.
MoreTranslated text
Key words
severe traumatic brain injury,brain injury,prognostic value,machine learning predictive model,predictive model
AI Read Science
Must-Reading Tree
Example
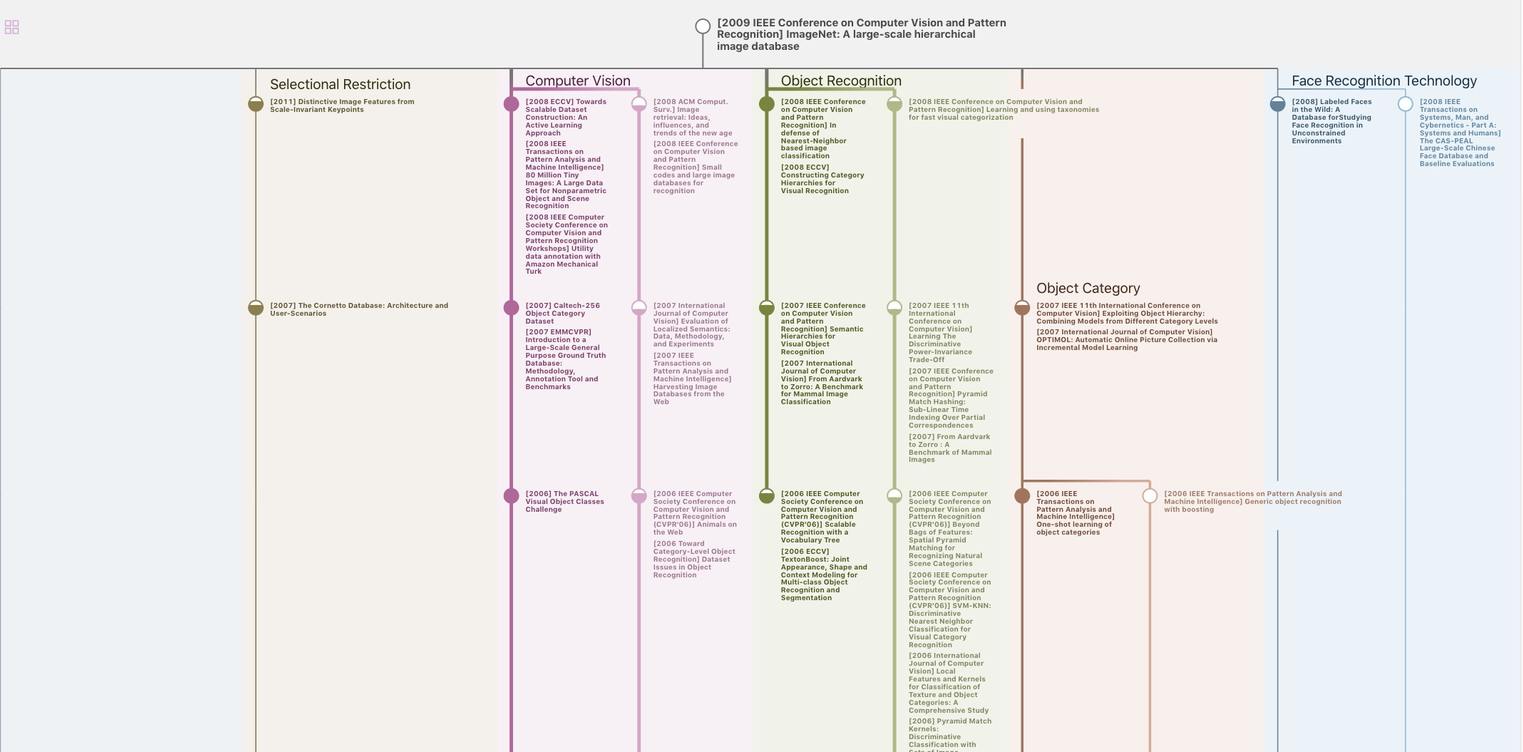
Generate MRT to find the research sequence of this paper
Chat Paper
Summary is being generated by the instructions you defined