Document-Level Named Entity Recognition With Q-Network
PRICAI 2019: TRENDS IN ARTIFICIAL INTELLIGENCE, PT III(2019)
摘要
Named entity recognition (NER) is typically viewed as a sequence labeling problem, where the solution is optimized in sentence level. In this paper, we explore utilizing document-level label consistency to improve NER performance by reinforcement learning. The process entails searching similar mentions and reconciling the labels, which are repeated until sufficient evidence is collected. We employ a Q-network, trained to maximize the total reward that reflects labeling accuracy while penalizing extra effort. On three publicly available datasets, our approach achieves an F-1 of 90.75%, outperforming the ensemble method (89.80%) by 0.95% and the best base tagger (88.16%) by 2.59% (The source code is available at https://github.com/KrisWentaoWong/dqnner/).
更多查看译文
关键词
Document-level named entity recognition, Q-network, Reinforcement learning
AI 理解论文
溯源树
样例
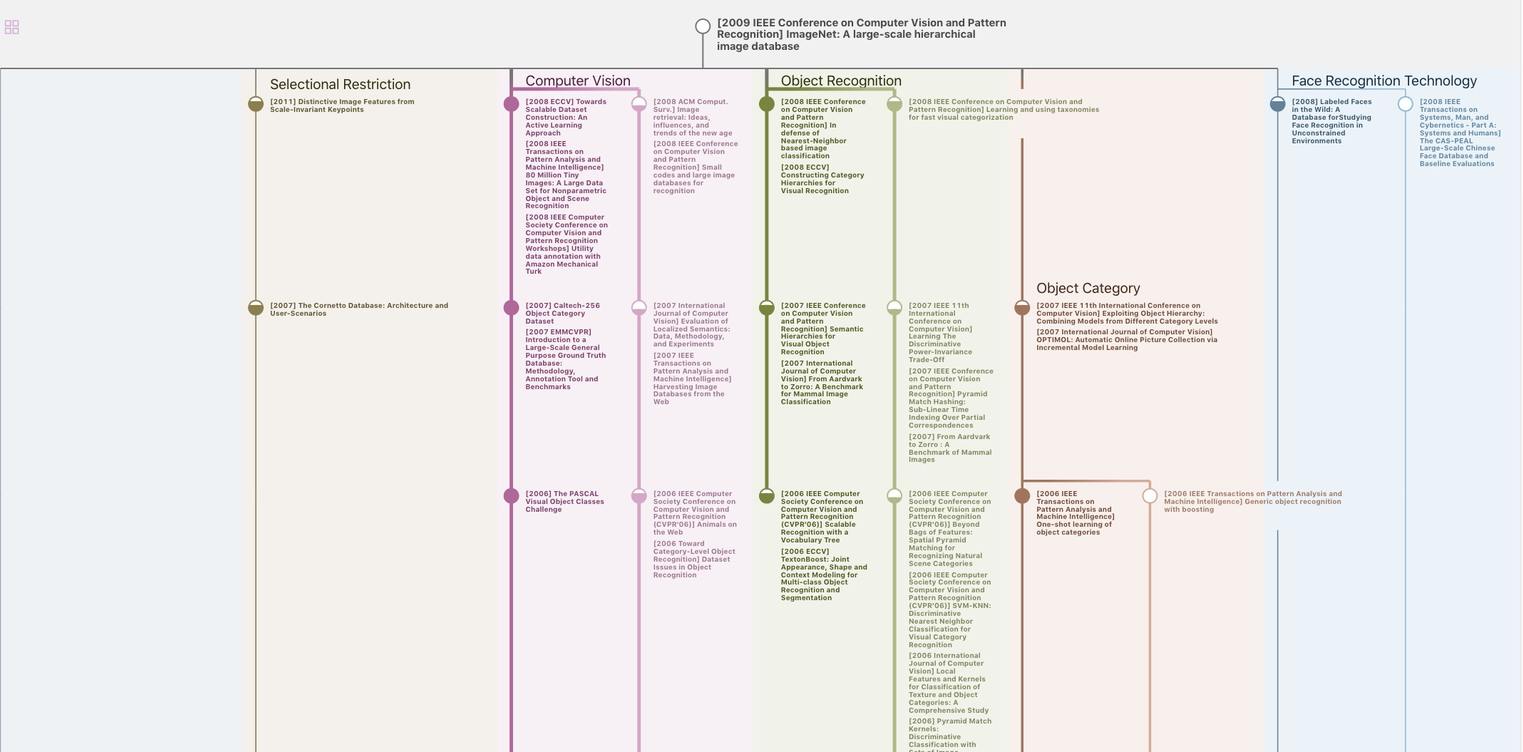
生成溯源树,研究论文发展脉络
Chat Paper
正在生成论文摘要