Multi-sensor prediction of Eucalyptus stand volume: A support vector approach
ISPRS Journal of Photogrammetry and Remote Sensing(2019)
摘要
Stem volume is a key attribute of Eucalyptus forest plantations upon which decision-making is based at diverse levels of planning. Quantifying volume through remote sensing can support a proper management of forests. Because of limitations on spaceborne optical and synthetic aperture radar sensors, this study integrated both types of datasets assembled using support vector regression (SVR) to retrieve the stand volume of Eucalyptus plantations. We assessed different combinations of sensors and a minimum number of plots to develop an SVR model. Finally, the best SVR performance was compared with other analytical methods already tested and in the literature: multilinear regression, artificial neural networks (ANN), and random forest (RF). Here, we introduce a test for comparative analysis of the performance of different methods. We found that SVR accurately predicted stem volume of Brazilian fast-growing Eucalyptus forest plantations. Gaussian radial basis was the most suitable kernel function. Integrating the optical and L-band backscatter data increased the predictive accuracy compared to a single sensor model. Combining NIR-band data from ALOS AVNIR-2 and backscatter of L-band horizontal emitted and vertical received (HV) electric fields from ALOS PALSAR produced the most accurate SVR model (with an R2 of 0.926 and root mean square error of 11.007 m3/ha). The number of field plots sufficient for model development with non-redundant explanatory variables was 77. Under this condition, SVR performed similarly to ANN and outperformed the multiple linear regression and random forest methods.
更多查看译文
关键词
ALOS AVNIR-2,ALOS PALSAR,Machine learning,Monte Carlo cross-validation,Sampling intensity,L-band,Synthetic aperture radar
AI 理解论文
溯源树
样例
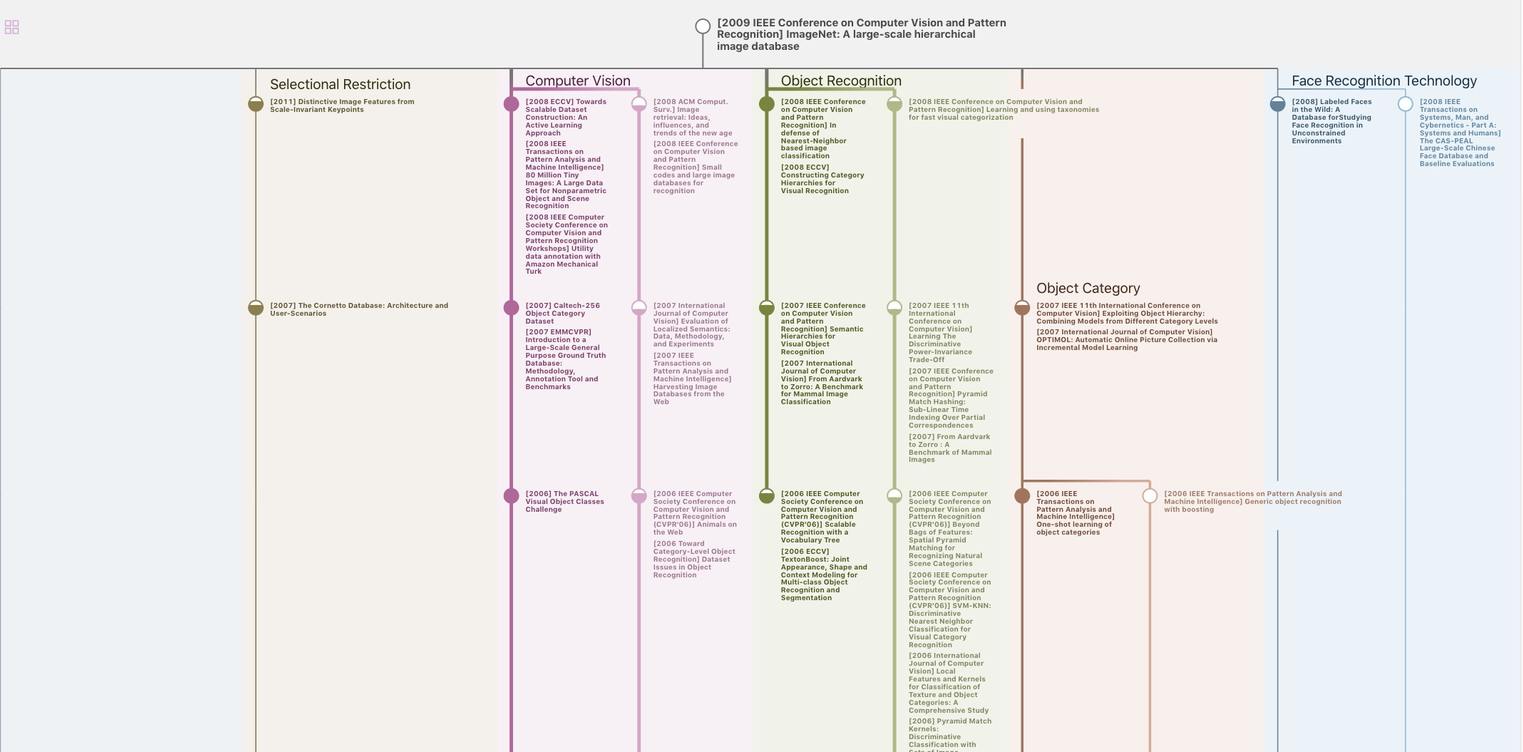
生成溯源树,研究论文发展脉络
Chat Paper
正在生成论文摘要