A Stationary Spatio-Temporal GARCH Model
JOURNAL OF TIME SERIES ANALYSIS(2020)
摘要
We introduce a lagged nearest-neighbour, stationary spatio-temporal generalized autoregressive conditional heteroskedasticity (GARCH) model on an infinite spatial grid that opens for GARCH innovations in a space-time ARMA model. This is illustrated by a real data application to a classical dataset of sea surface temperature anomalies in the Pacific Ocean. The model and its translation invariant neighbourhood system are wrapped around a torus forming a model with finite spatial domain, which we call circular spatio-temporal GARCH. Such a model could be seen as an approximation of the infinite one and simulation experiments show that the circular estimator with a straightforward bias correction performs well on such non-circular data. Since the spatial boundaries are tied together, the well-known boundary issue in spatial statistical modelling is effectively avoided. We derive stationarity conditions for these circular processes and study the spatio-temporal correlation structure through an ARMA representation. We also show that the matrices defined by a vectorized version of the model are block circulants. The maximum quasi-likelihood estimator is presented and we prove its strong consistency and asymptotic normality by generalizing results from univariate GARCH theory.
更多查看译文
关键词
Circulant,circular,CSTGARCH,GARCH,space-time,STGARCH
AI 理解论文
溯源树
样例
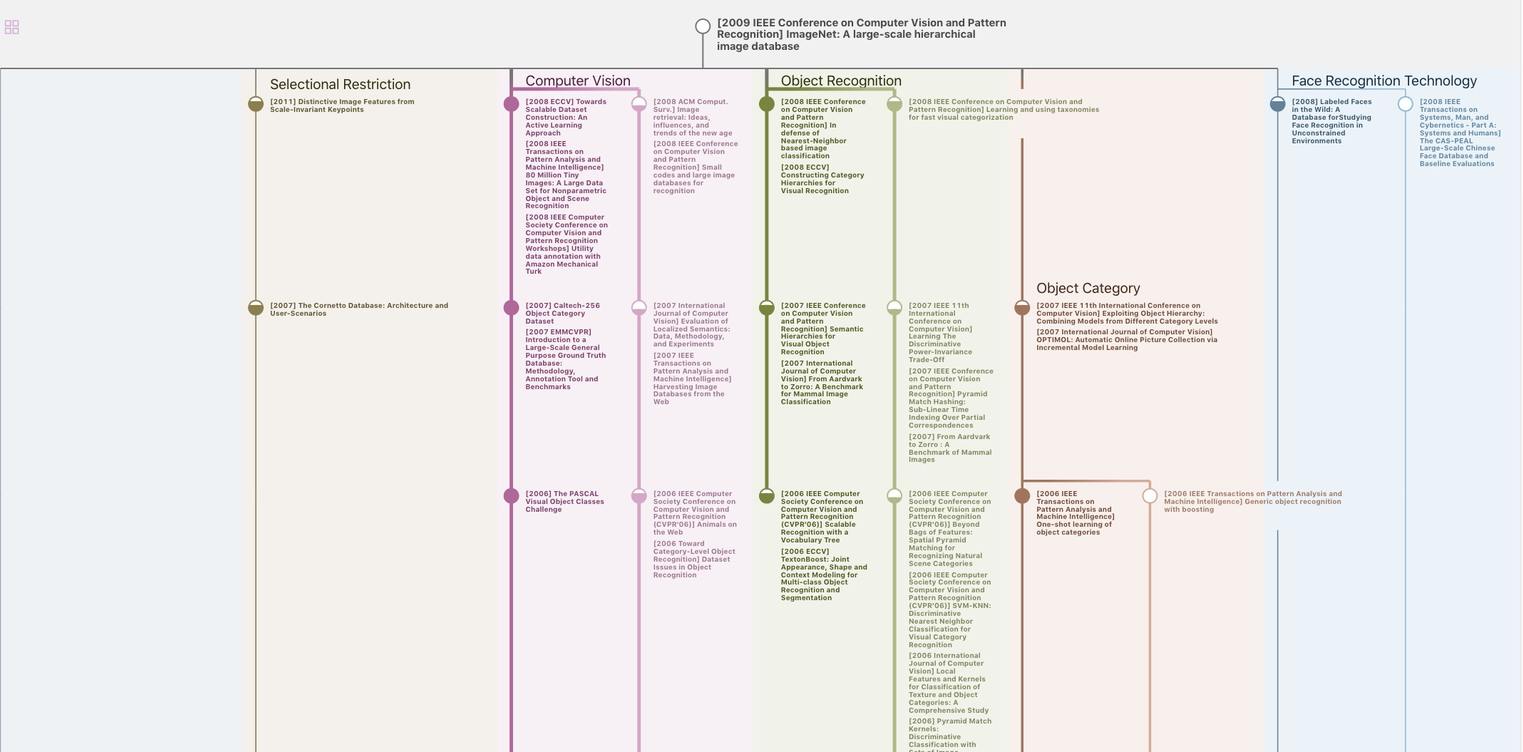
生成溯源树,研究论文发展脉络
Chat Paper
正在生成论文摘要