An Improved Sarsa(λ) Reinforcement Learning Algorithm for Wireless Communication Systems.
IEEE Access(2019)
摘要
In this article, we provide a novel improved model-free temporal-difference control algorithm, namely, Expected Sarsa(lambda), using the average value as an update target and introducing eligibility traces in wireless communication networks. In particular, we construct the update target using the average action value of all possible successive actions, and apply eligibility traces to record the historical access of every state action pair, which greatly improve the model's convergence property and learning efficiency. Numerical results demonstrate that the proposed algorithm has the advantage of high learning efficiency and a higher learning-rate tolerance range than Q Learning, Sarsa, Expected Sarsa, and Sarsa(lambda) in the tabular case of a finite Markov decision process, thereby providing an efficient solution for the study and design wireless communication networks. This provides an efficient and effective solution to design further artificial intelligent communication networks.
更多查看译文
关键词
Model-free reinforcement learning,Sarsa,Q learning,eligibility traces
AI 理解论文
溯源树
样例
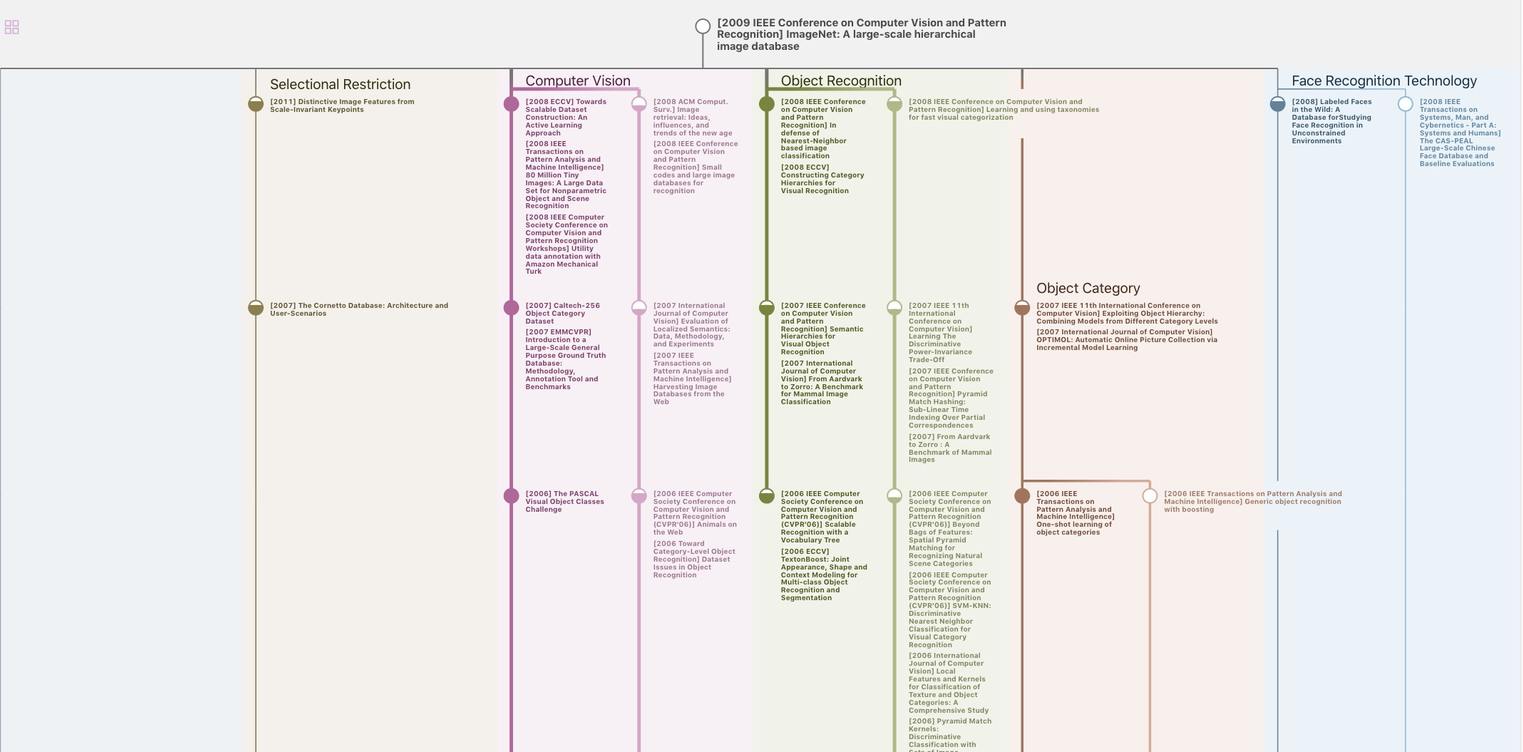
生成溯源树,研究论文发展脉络
Chat Paper
正在生成论文摘要